Image classification ¶
Getting Started ¶
Loading Google Drive ¶
First, we need to mount the Google Drive so we can access the Distributed Acoustic Sensing data stored in it. After execution, the following script will provide a link. Open the link and follow the instructions to get the one-time password. Copy-paste the authorization code in the dialog box below. If you have several Google accounts logged on your computer, make sure you use the one the DAS data were shared with.
[ ]:
from google.colab import drive
drive.mount('/content/drive')
Go to this URL in a browser: https://accounts.google.com/o/oauth2/auth?client_id=947318989803-6bn6qk8qdgf4n4g3pfee6491hc0brc4i.apps.googleusercontent.com&redirect_uri=urn%3aietf%3awg%3aoauth%3a2.0%3aoob&scope=email%20https%3a%2f%2fwww.googleapis.com%2fauth%2fdocs.test%20https%3a%2f%2fwww.googleapis.com%2fauth%2fdrive%20https%3a%2f%2fwww.googleapis.com%2fauth%2fdrive.photos.readonly%20https%3a%2f%2fwww.googleapis.com%2fauth%2fpeopleapi.readonly&response_type=code
Enter your authorization code:
··········
Mounted at /content/drive
To keep things organized, you can create a new directory on your Google Drive where all the saved outputs will be stored. For example, in my case, I created a
Vincent_Dumont
directory under the
ML4DAS/Analysis/
repository. You can then move to that directory as follows:
[ ]:
import os
os.chdir('/content/drive/Shared drives/ML4DAS/Analysis/Vincent_Dumont/Probability Maps')
MLDAS Software ¶
In this section, we will use a set of pre-selected images produced in the
Systematic search for suitable training images
notebook where clear surface wave or noise patterns are distinguished based on there data distribution. In this section, we will functions from the
`mldas
<
https://ml4science.gitlab.io/mldas/
>`__ Python package.
[ ]:
%%capture
!pip install -U mldas
Mapping and Plotting ¶
[ ]:
import numpy
from PIL import Image
from matplotlib import cm
import torch.nn.functional as F
from torchvision import transforms
def extract_prob_map(data,model,img_size=50,channel_stride=1,sample_stride=10,verbose=False,stage_plot=False):
model.eval() # Set model to evalutation mode
prob_array = numpy.zeros((2,*data.shape)) # Initialize probability map
idxs = numpy.array([[[i,j] for j in range(0,data.shape[1]-img_size+1,sample_stride)] for i in range(0,data.shape[0]-img_size+1,channel_stride)])
idxs = idxs.reshape(idxs.shape[0]*idxs.shape[1],2)
if verbose: print('Processing %i regions...'%len(idxs))
for k,(i,j) in enumerate(idxs):
if verbose and (k+1)%(len(idxs)//10)==0:
print('%i%% processed (%i/%i)'%(round((k+1)/len(idxs)*100),k+1,len(idxs)))
im = data[i:i+img_size,j:j+img_size].copy() # Create copy of square data window
im = (im-im.min())/(im.max()-im.min()) # Normalize data
im = Image.fromarray(numpy.uint8(cm.gist_earth(im)*255)).convert("RGB") # Convert data to RGB image
image = transforms.ToTensor()(im).float().unsqueeze(0) # Convert image to tensor and use first channel
output = model(image) # Run trained model to image
prob = F.softmax(output,dim=1).topk(2) # Get probability for each class
assert int(prob[1][0,0]) in [0,1],"Maximum probability class has an unknown label..." # Check if label not found
wave_prob = 1-float(prob[0][0,0]) if int(prob[1][0,0])==0 else float(prob[0][0,0]) # Get surface wave probability
prob_array[0,i:i+img_size,j:j+img_size]+=wave_prob # Increment probability to map
prob_array[1,i:i+img_size,j:j+img_size]+=1 # Increment scanning index to map
if stage_plot: # If frame requested to be plotted
numpy.seterr(divide='ignore', invalid='ignore') # Ignore divide by zero warning message
plot_frame(i,j,data,im,prob_array[0]/prob_array[1],img_size,wave_prob,n+1) # Plot data and probability map at current scanning stage
return prob_array[0]/prob_array[1] # Return weighted probability for every pixel
[ ]:
import matplotlib.pyplot as plt
def plot_prob_map(data,prob):
plt.style.use('seaborn')
fig,ax = plt.subplots(1,2,figsize=(18,6),dpi=80,sharex=True,sharey=True)
ax[0].imshow(data,cmap='seismic',aspect='auto')
ax[0].set_title('Raw data')
ax[0].set_xlabel('Time [second]')
ax[0].set_ylabel('Channels')
im = ax[1].imshow(prob,aspect='auto',cmap='jet',vmin=0,vmax=1)
ax[1].set_title('Probability map')
ax[1].set_xlabel('Time [second]')
plt.colorbar(im,ax=ax[1])
plt.tight_layout()
plt.show()
[ ]:
import h5py,numpy
from scipy.fft import fft,fftfreq
from matplotlib.colors import LogNorm
from matplotlib.offsetbox import AnchoredText
import torch.nn.functional as F
import matplotlib.pyplot as plt
from PIL import Image
from matplotlib import cm
from torchvision import transforms
def freq_content(fname,i,j,model,img_size=100):
model.eval()
# Load file
f = h5py.File('/content/drive/Shared drives/ML4DAS/RawData/1min_ch4650_4850/'+fname,'r')
data = numpy.array(f[f.get('variable/dat')[0,0]][i:i+img_size,j:j+img_size])
f.close()
# Get surface wave probability
im = data.copy()
im = (im-im.min())/(im.max()-im.min())
im = Image.fromarray(numpy.uint8(cm.gist_earth(im)*255)).convert("RGB")
image = transforms.ToTensor()(im).float().unsqueeze(0)
output = model(image)
prob = F.softmax(output,dim=1).topk(2)
wave_prob = 1-float(prob[0][0,0]) if int(prob[1][0,0])==0 else float(prob[0][0,0])
print(wave_prob)
# Plot results
plt.style.use('seaborn')
fig,ax = plt.subplots(1,4,figsize=(20,5),dpi=80)
ffts = numpy.array([2.0/len(time_serie)*numpy.abs(fft(time_serie)[:len(time_serie)//2]) for time_serie in data])
img_max = abs(data).max()
# Show larger image
ax[0].imshow(data,aspect='auto',cmap='seismic',extent=[0,img_size,img_size,0],vmin=-img_max,vmax=img_max)
ax[0].set_xlabel('Sample')
ax[0].set_ylabel('Channel')
# Plotting data distribution
ax[1].hist(data.reshape(numpy.prod(data.shape)),bins=50)
at = AnchoredText('$\sigma=%i$'%numpy.std(data),prop=dict(size=12),loc='upper left')
ax[1].add_artist(at)
ax[1].set_xlabel('Strain Measurement')
# D2 and plot FFT for each channel
ax[2].imshow(ffts,extent=[0,250,data.shape[0],0],aspect='auto',norm=LogNorm(),cmap='jet')
ax[2].set_xlabel('Frequency (Hz)')
ax[2].set_ylabel('Channels')\
# Plot average amplitude for each frequency
x = fftfreq(img_size,d=1/500)[:img_size//2]
y = [numpy.average(freq) for freq in ffts.T]
ax[3].plot(x,y)
ax[3].set_xlabel('Frequency (Hz)')
ax[3].set_xlim(0,250)
ax[3].set_ylabel('Average Amplitude')
plt.show()
plt.close()
20k 2-class 50x50 dataset ¶
A total of 20,000 surface wave images and 20,000 noise images were saved and split into 3 sets: 70% for training, 15% for testing and 15% for validation. The training images were saved and compressed into a tar file which can be extracted locally as follows:
[ ]:
%%capture
!cd /content && tar -zxvf /content/drive/Shared\ drives/ML4DAS/Analysis/Vincent_Dumont/Systematic\ Search/datasets_20k.tar.gz
The tensor data can be loaded using the
`ImageFolder
<
https://pytorch.org/docs/stable/torchvision/datasets.html#torchvision.datasets.ImageFolder
>`__ class from the
torchvision
package.
[ ]:
from torchvision import transforms, datasets
mytransforms = transforms.Compose([transforms.ToTensor()])
# Load training and testing binary class datasets
trainset = datasets.ImageFolder(root='/content/train',transform=mytransforms)
testset = datasets.ImageFolder(root='/content/test',transform=mytransforms)
# Create data loader for binary class datasets
train_loader = torch.utils.data.DataLoader(trainset,batch_size=500,shuffle=True)
test_loader = torch.utils.data.DataLoader(testset,batch_size=500,shuffle=True)
Finally, in order to visualize how each training performs, we will use the data patch and look at the probability map.
[ ]:
import h5py,numpy
fname = args.datapath+'30min_files_NoTrain/Dsi_30min_170804230056_170804233056_ch5500_6000.mat'
sample_min,sample_max = 330900,331800
channel_min,channel_max = 200,500
f = h5py.File(fname,'r')
data = numpy.array(f[f.get('dsi30/dat')[0,0]][channel_min:channel_max,sample_min:sample_max])
f.close()
Here, we will not do the Adaptive Batch Size Adversarial training and instead execute a simple training process with standard back-propagation and gradient descent implementation. We start by running only one epoch and test the validation after each batch iteration. The validation step was implemented using a similar scheme than what described in this blog .
Training 1: 20-layer 1-epoch model with 0.001 learning rate ¶
[ ]:
import mldas
model = mldas.ResNet(depth=20,num_classes=2)
criterion = torch.nn.CrossEntropyLoss()
optimizer = torch.optim.SGD(model.parameters(), lr=0.001, momentum=0.9)
loss_hist,models = mldas.suplearn_simple(model,criterion,optimizer,train_loader,test_loader,epochs=1,save_model=True,verbose=True)
[ ]:
import os
fname = 'training'
os.system('mkdir %s'%fname)
numpy.save('%s/loss_hist'%fname,loss_hist)
for key in models.keys():
torch.save(models[key].state_dict(),'%s/model%02i.pt'%(fname,key))
[ ]:
import mldas
model = mldas.ResNet(depth=20,num_classes=2)
loss_hist = numpy.load('training1/loss_hist.npy')
for k in range(len(loss_hist)):
mldas.plot_map_stage(data,model,loss_hist,'training1',k,savefig=True)
[ ]:
%%capture
!ffmpeg -i training1/model%02d.png training1/video.mp4
[ ]:
from IPython.display import HTML
from base64 import b64encode
mp4 = open('training1/video.mp4','rb').read()
data_url = "data:video/mp4;base64," + b64encode(mp4).decode()
HTML("""<video width=100%% controls loop><source src="%s" type="video/mp4"></video>""" % data_url)
Training 2: 8-layer 1-epoch model with 0.01 learning rate ¶
[ ]:
import mldas
model = mldas.ResNet(depth=8,num_classes=2)
loss_hist = numpy.load('training2/loss_hist.npy')
for k in range(len(loss_hist)):
mldas.plot_map_stage(data,model,loss_hist,'training2',k,savefig=True)
[ ]:
%%capture
!ffmpeg -i training2/model%02d.png training2/video.mp4
[ ]:
from IPython.display import HTML
from base64 import b64encode
mp4 = open('training2/video.mp4','rb').read()
data_url = "data:video/mp4;base64," + b64encode(mp4).decode()
HTML("""<video width=100%% controls loop><source src="%s" type="video/mp4"></video>""" % data_url)
Test on single images ¶
[ ]:
%%capture
!cd /content && tar -zxvf /content/drive/Shared\ drives/ML4DAS/Analysis/Vincent_Dumont/Systematic\ Search/set_20k_50x50_class2.tar.gz
[ ]:
import h5py,numpy,mldas,torch,glob
import torch.nn.functional as F
import matplotlib.pyplot as plt
from torchvision import transforms
from matplotlib import cm
from PIL import Image
model = mldas.ResNet(depth=20,num_classes=2)
model.load_state_dict(torch.load('training1/model55.pt'))
model.eval()
noise = glob.glob('/content/train/noise/*.jpg')[0]
waves = glob.glob('/content/train/waves/*.jpg')[0]
for data in [noise,waves]:
img = Image.open(data)
# img = img.convert('RGB')
# img = torch.from_numpy(numpy.asarray(img))
plt.imshow(img)
plt.show()
# img = Image.fromarray(numpy.uint8(img))
print(img)
# img = transforms.ToTensor()(img).float().unsqueeze(0)
img = Image.fromarray(numpy.uint8(cm.gist_earth(img)*255)).convert("RGB")
img = transforms.ToTensor()(img).float().unsqueeze(0)
output = model(img)
prob = F.softmax(output,dim=1).topk(2)
print(prob)
wave_prob = 1-float(prob[0][0,0]) if int(prob[1][0,0])==0 else float(prob[0][0,0])
print(wave_prob)
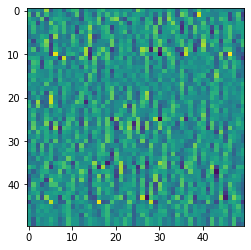
<PIL.JpegImagePlugin.JpegImageFile image mode=L size=50x50 at 0x7FEFAC08AA90>
torch.return_types.topk(
values=tensor([[1.0000e+00, 2.7292e-28]], grad_fn=<TopkBackward>),
indices=tensor([[1, 0]]))
1.0
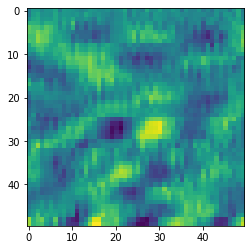
<PIL.JpegImagePlugin.JpegImageFile image mode=L size=50x50 at 0x7FEFAC08AA90>
torch.return_types.topk(
values=tensor([[1.0000e+00, 2.7292e-28]], grad_fn=<TopkBackward>),
indices=tensor([[1, 0]]))
1.0
[ ]:
import h5py,numpy,mldas,torch
import torch.nn.functional as F
import matplotlib.pyplot as plt
from torchvision import transforms
from matplotlib import cm
from PIL import Image
model = mldas.ResNet(depth=8,num_classes=2)
model.load_state_dict(torch.load('training2/model45.pt'))
model.eval()
f = h5py.File('/content/drive/Shared drives/ML4DAS/RawData/30min_files_Train/Dsi_30min_170730023007_170730030007_ch5500_6000_NS.mat','r')
data = f[f.get('dsi30/dat')[0,0]]
print
for n,(label,i,j) in enumerate([['noise', 201, 384672], ['waves', 193, 921372], ['high', 157, 685516]]):
im = numpy.array(data[i+100:i+150,j+100:j+150])
plt.imshow(im)
plt.show()
im = (im-im.min())/(im.max()-im.min())
im = Image.fromarray(numpy.uint8(cm.gist_earth(im)*255)).convert("RGB")
image = transforms.ToTensor()(im).float().unsqueeze(0)
output = model(image)
prob = F.softmax(output,dim=1).topk(2)
wave_prob = 1-float(prob[0][0,0]) if int(prob[1][0,0])==0 else float(prob[0][0,0])
print(wave_prob)
f.close()
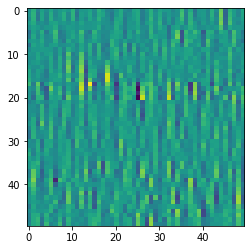
0.22629565000534058
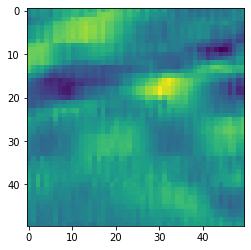
0.8843898773193359
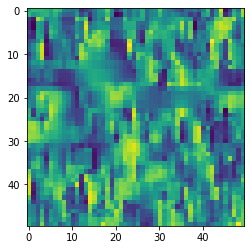
0.16676467657089233
Training 3: 8-layer 1-epoch model with 0.05 learning rate ¶
[ ]:
import mldas
model = mldas.ResNet(depth=8,num_classes=2)
loss_hist = numpy.load('training3/loss_hist.npy')
for k in range(len(loss_hist)):
print(k+1,'/',len(loss_hist))
mldas.plot_map_stage(data,model,loss_hist,'training3',k,savefig=True,overwrite=True)
[ ]:
%%capture
!ffmpeg -i training3/model%02d.png training3/video.mp4
[ ]:
from IPython.display import HTML
from base64 import b64encode
mp4 = open('training3/video.mp4','rb').read()
data_url = "data:video/mp4;base64," + b64encode(mp4).decode()
HTML("""<video width=100%% controls loop><source src="%s" type="video/mp4"></video>""" % data_url)
Training 4: 8-layer 2-epoch model with 0.05 learning rate ¶
[ ]:
import mldas
model = mldas.ResNet(depth=8,num_classes=2)
loss_hist = numpy.load('training4/loss_hist.npy')
for k in range(len(loss_hist)):
print(k+1,'/',len(loss_hist))
mldas.plot_map_stage(data,model,loss_hist,'training4',k,savefig=True,overwrite=True)
[ ]:
%%capture
!ffmpeg -i training4/model%02d.png training4/video.mp4
[ ]:
from IPython.display import HTML
from base64 import b64encode
mp4 = open('training4/video.mp4','rb').read()
data_url = "data:video/mp4;base64," + b64encode(mp4).decode()
HTML("""<video width=100%% controls loop><source src="%s" type="video/mp4"></video>""" % data_url)
Full one-minute data ¶
Trained ResNet model ¶
[ ]:
%%capture
!pip install mldas==0.7.8
[ ]:
import mldas,torch
model = mldas.ResNet(depth=20,num_classes=2)
model.load_state_dict(torch.load('../Unary vs. Binary/model55.pt'))
<All keys matched successfully>
Refined 1-min probability map ¶
[ ]:
import h5py,numpy
f = h5py.File('/content/drive/Shared drives/ML4DAS/RawData/1min_ch4650_4850/westSac_180103010131_ch4650_4850.mat','r')
data = numpy.array(f[f.get('variable/dat')[0,0]])[:200]
f.close()
[ ]:
import numpy
prob_map = mldas.extract_prob_map(data,model,img_size=50,channel_stride=2,sample_stride=10)
[ ]:
import re,glob,numpy,h5py
import matplotlib.pyplot as plt
from matplotlib.colors import LogNorm
# Initialize the figure
plt.style.use('seaborn')
fig,ax = plt.subplots(1,2,figsize=(18,5),dpi=80,sharex=True,sharey=True)
# Plot original image
ax[0].imshow(abs(data),cmap='plasma',aspect='auto',norm=LogNorm())
ax[0].set_title('Raw strain measurements')
# Plot probability map
ax[1].imshow(prob_map,cmap ='inferno',aspect='auto',vmin=0,vmax=1)
ax[1].set_title('Probability map')
plt.tight_layout()
plt.show()
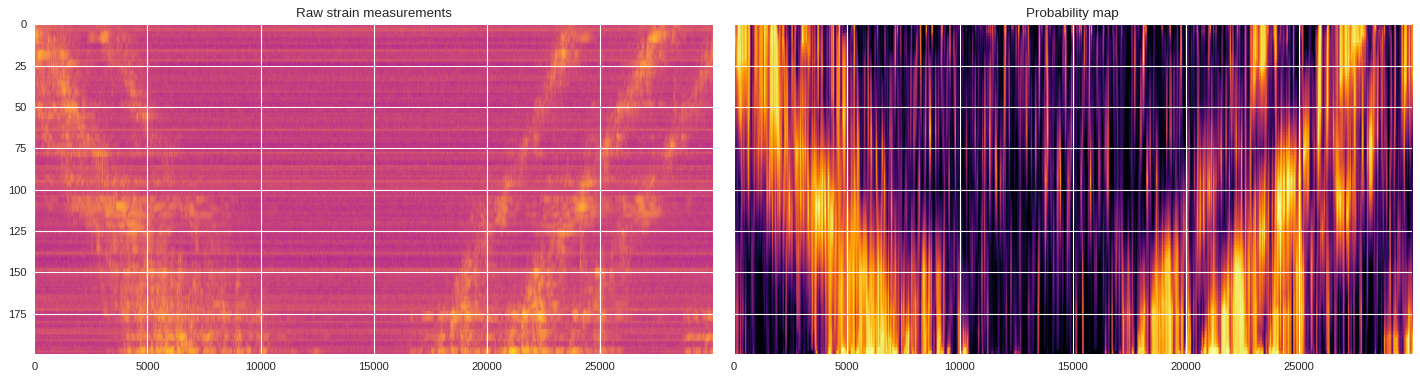
Probability distribution ¶
[ ]:
import re,glob,numpy,h5py
import matplotlib.pyplot as plt
from google.colab import widgets
div = 20
tb = widgets.TabBar([str(i) for i in range(div)])
for k in range(div):
pmin,pmax = k/div,(k+1)/div
with tb.output_to(k, select=False):
# Initialize the figure
plt.style.use('seaborn')
fig,ax = plt.subplots(3,1,figsize=(18,10),dpi=80)
# Plot probability map
ax[0].imshow(prob_map,cmap ='jet',aspect='auto')
ax[0].set_title('Probability map')
# Plot threshold map
probmap = numpy.logical_and(pmin<prob_map,prob_map<=pmax)
ax[1].imshow(probmap,cmap ='spring',aspect='auto')
ax[1].set_title('{:,d} pixels with probability between {:.2f} and {:.2f}'.format(len(prob_map[numpy.where(probmap)]),pmin,pmax))
# Plot distribution of probability values
ax[2].axvspan(pmin,pmax,color='yellow',alpha=0.7)
ax[2].hist(prob_map.reshape(numpy.prod(prob_map.shape)),bins='auto')
ax[2].set_yscale('log', nonposy='clip')
ax[2].axvline(pmin,ls='dotted',color='yellow')
ax[2].axvline(pmax,ls='dotted',color='yellow')
ax[2].set_title('Distribution of probability values')
ax[2].set_xlim(0,1)
plt.tight_layout()
plt.show()
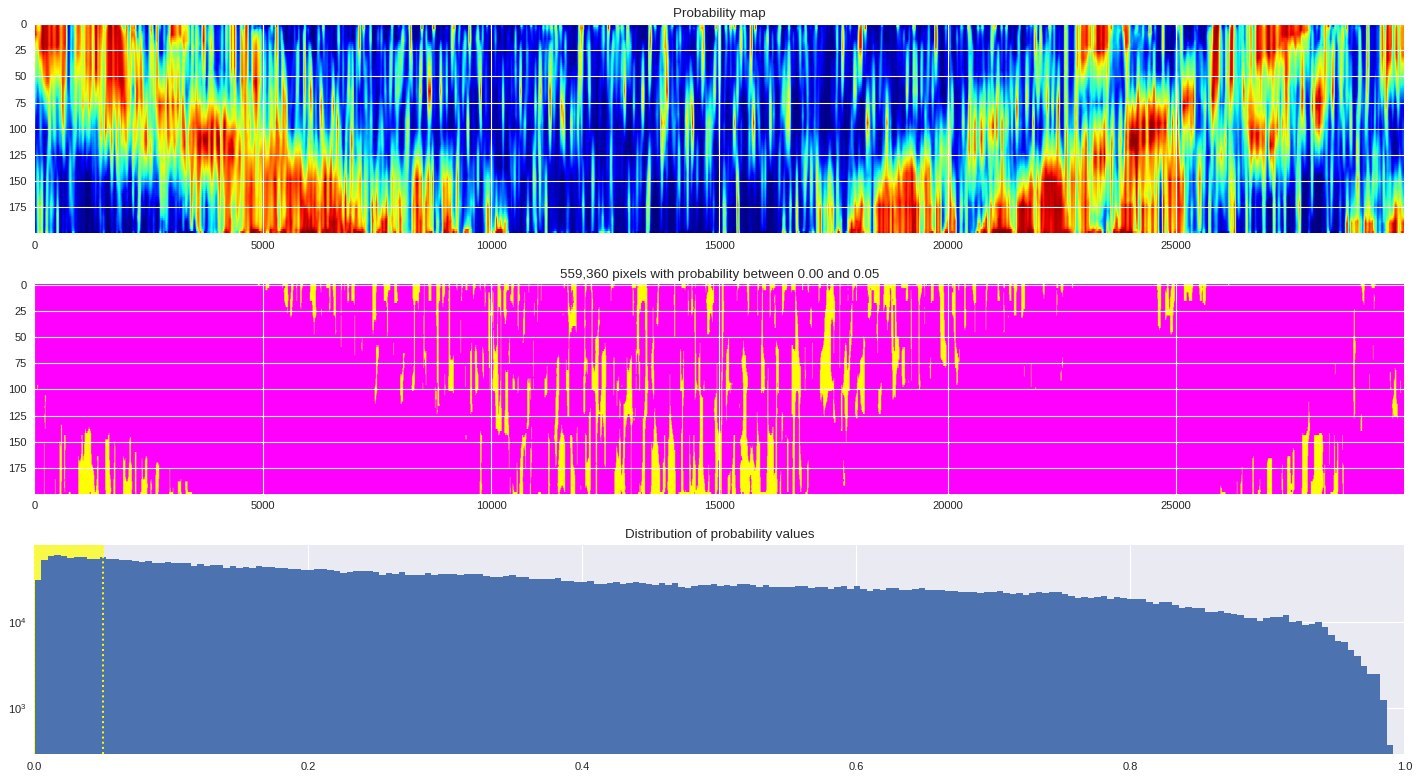
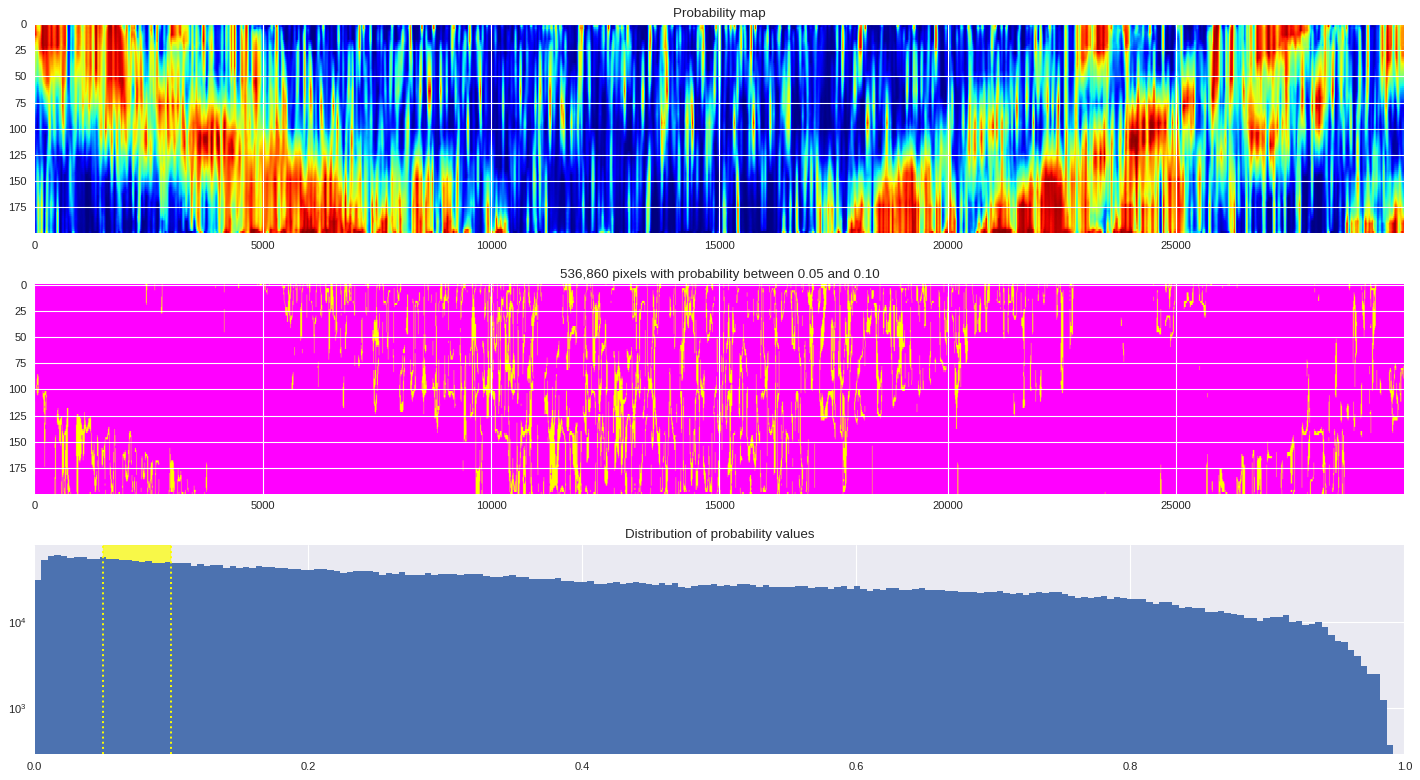
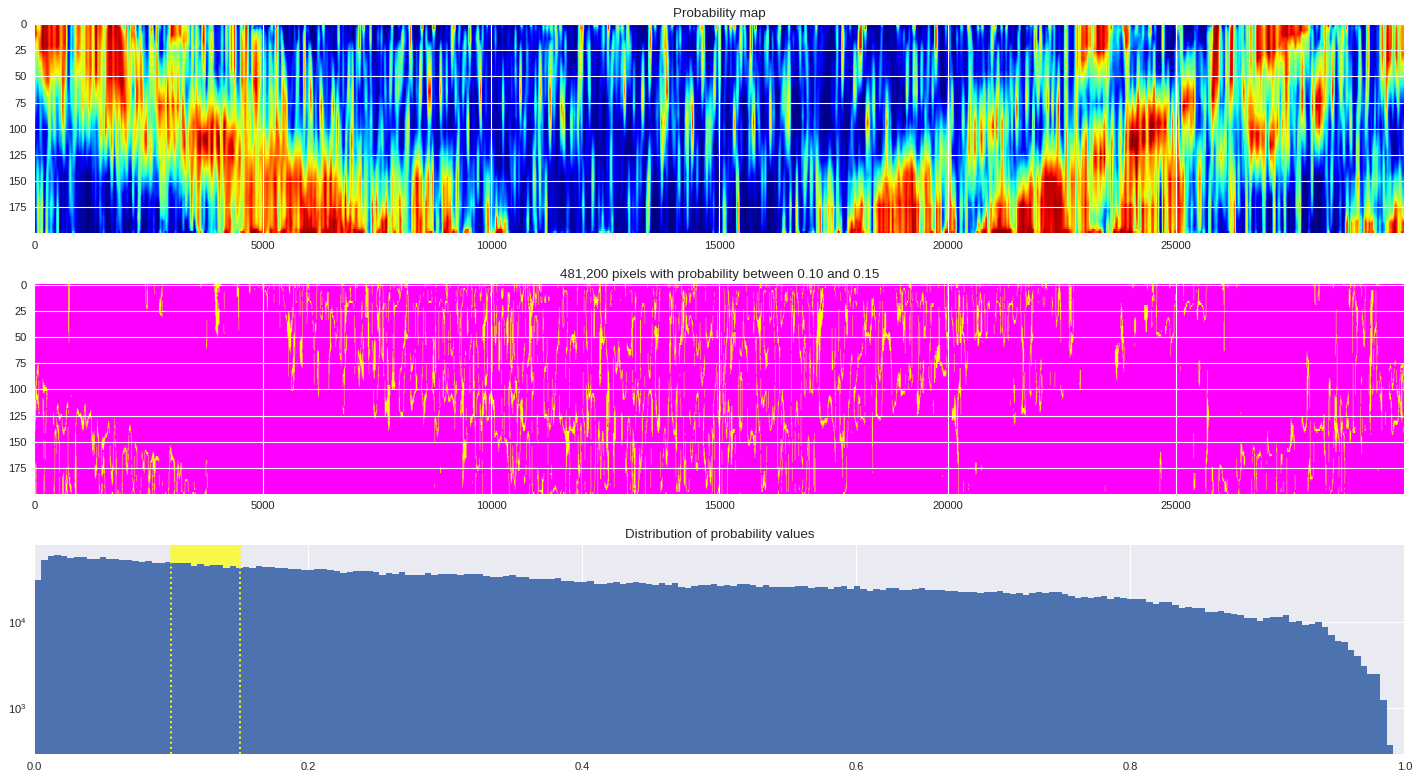
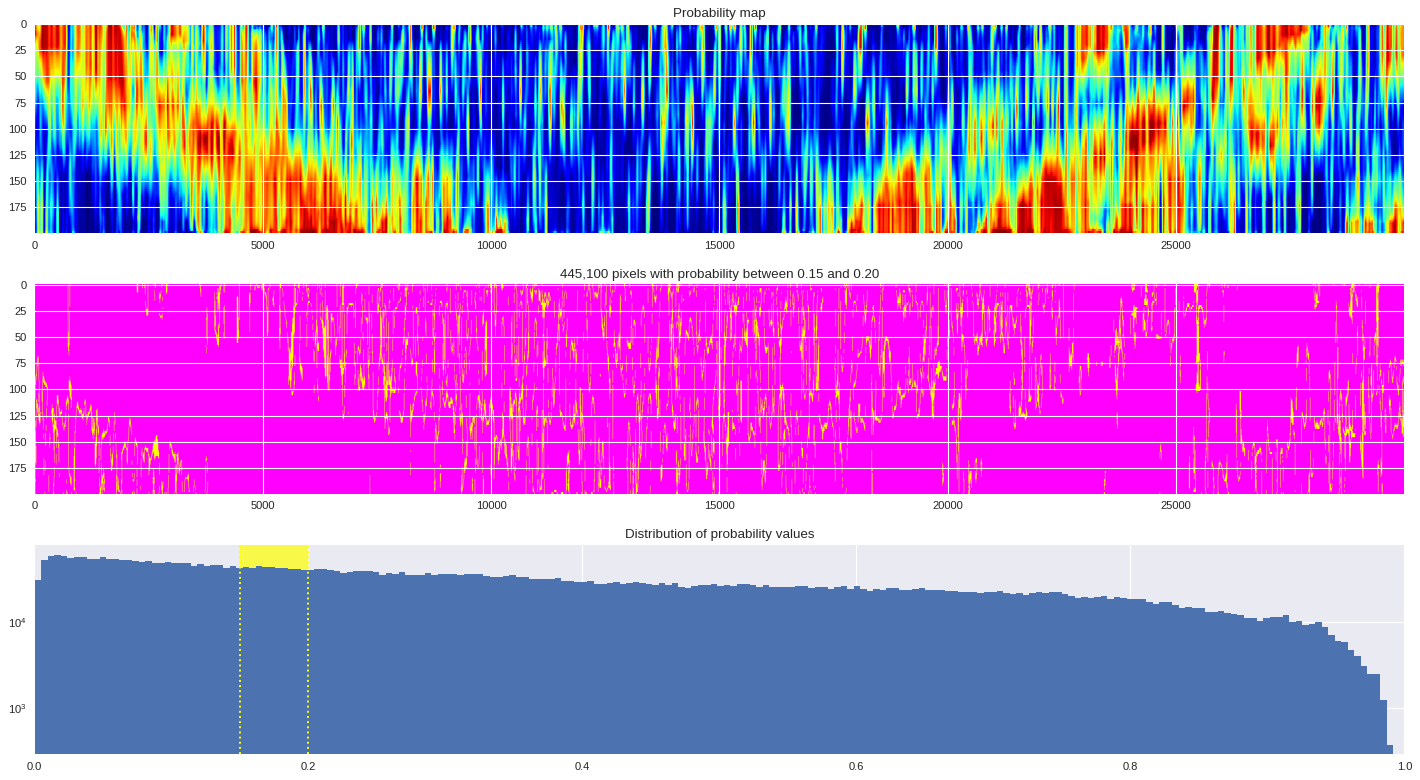
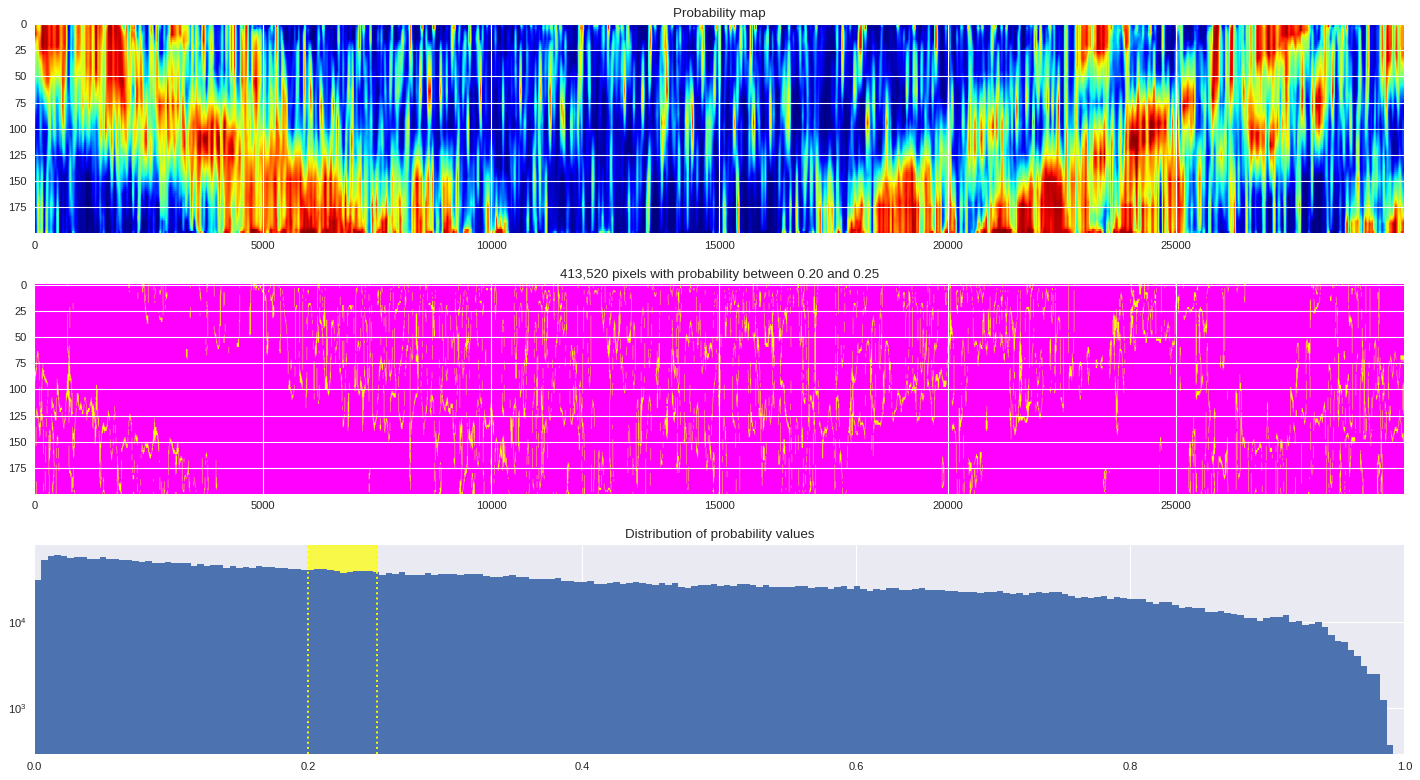
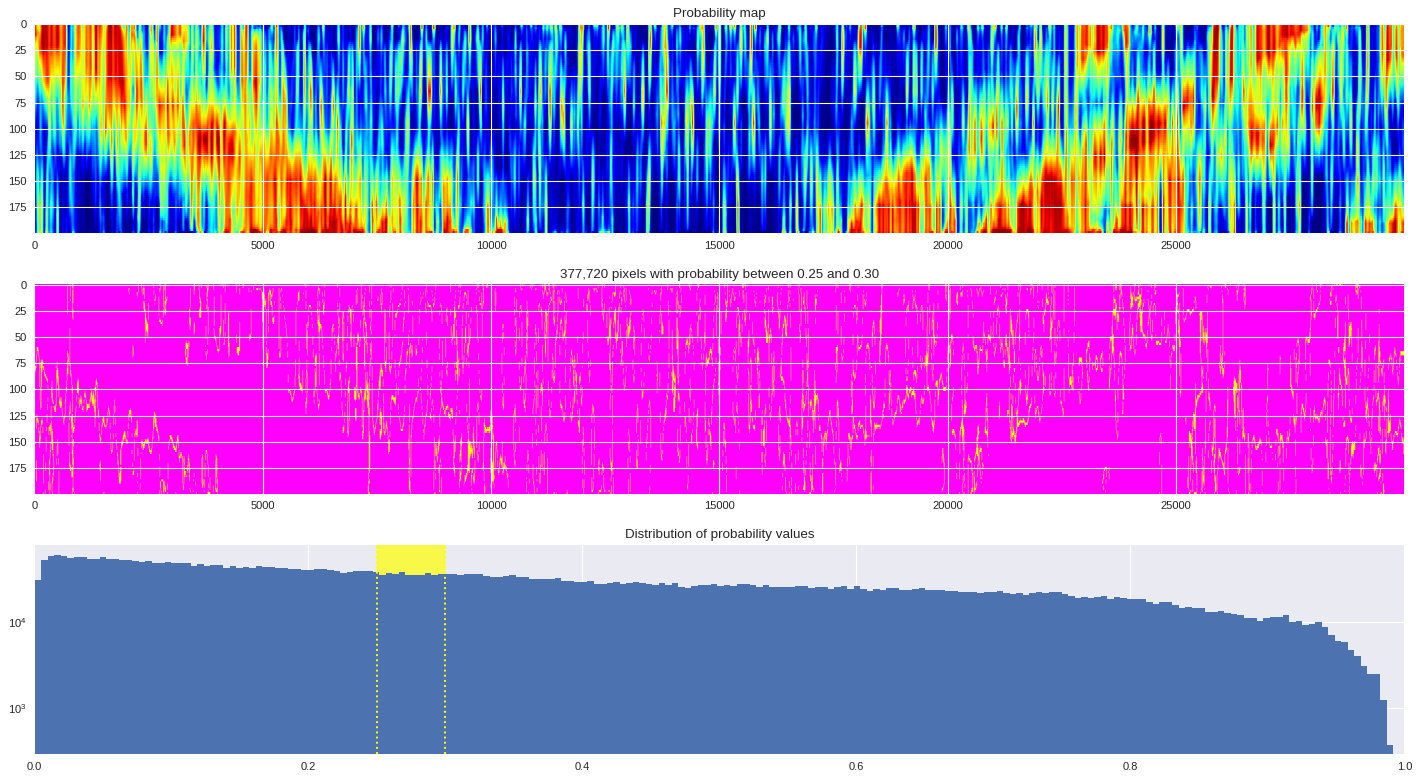
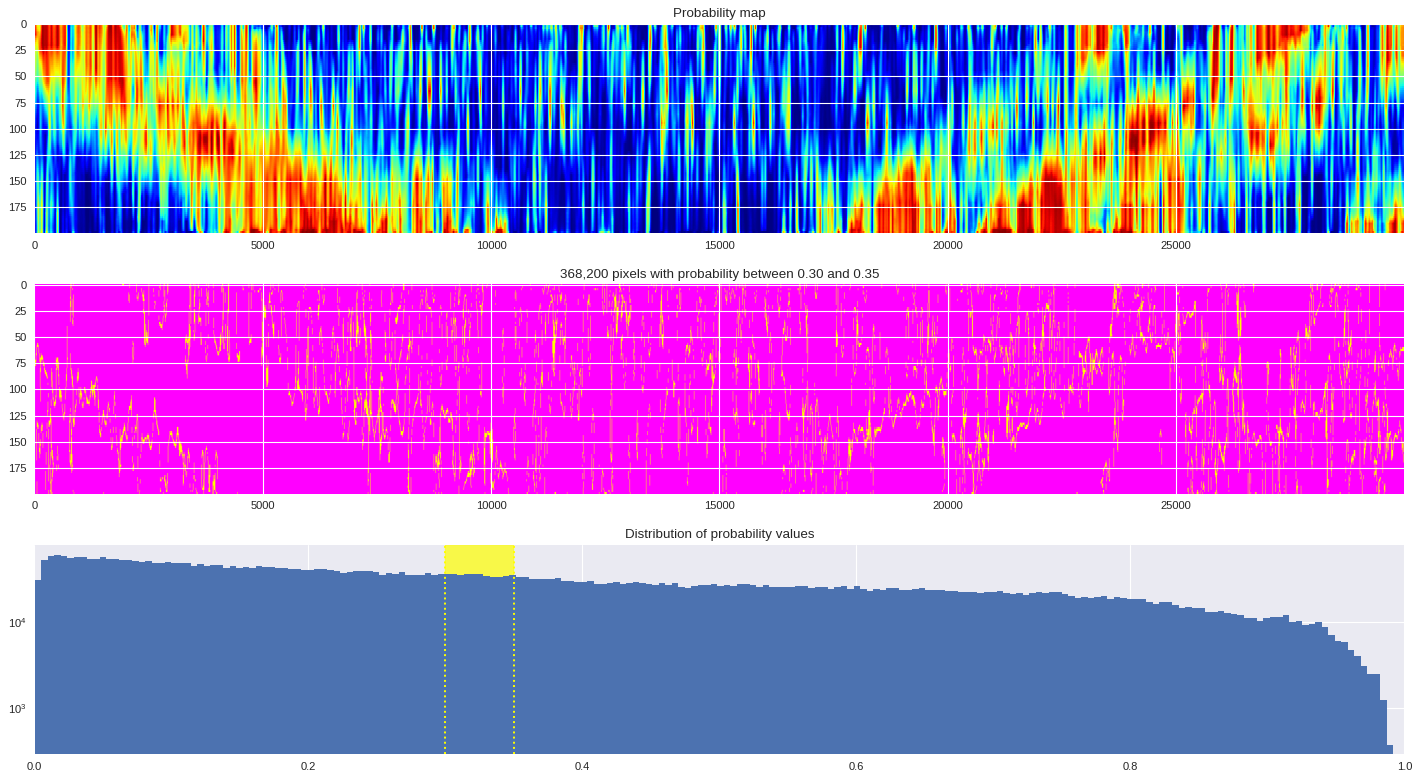
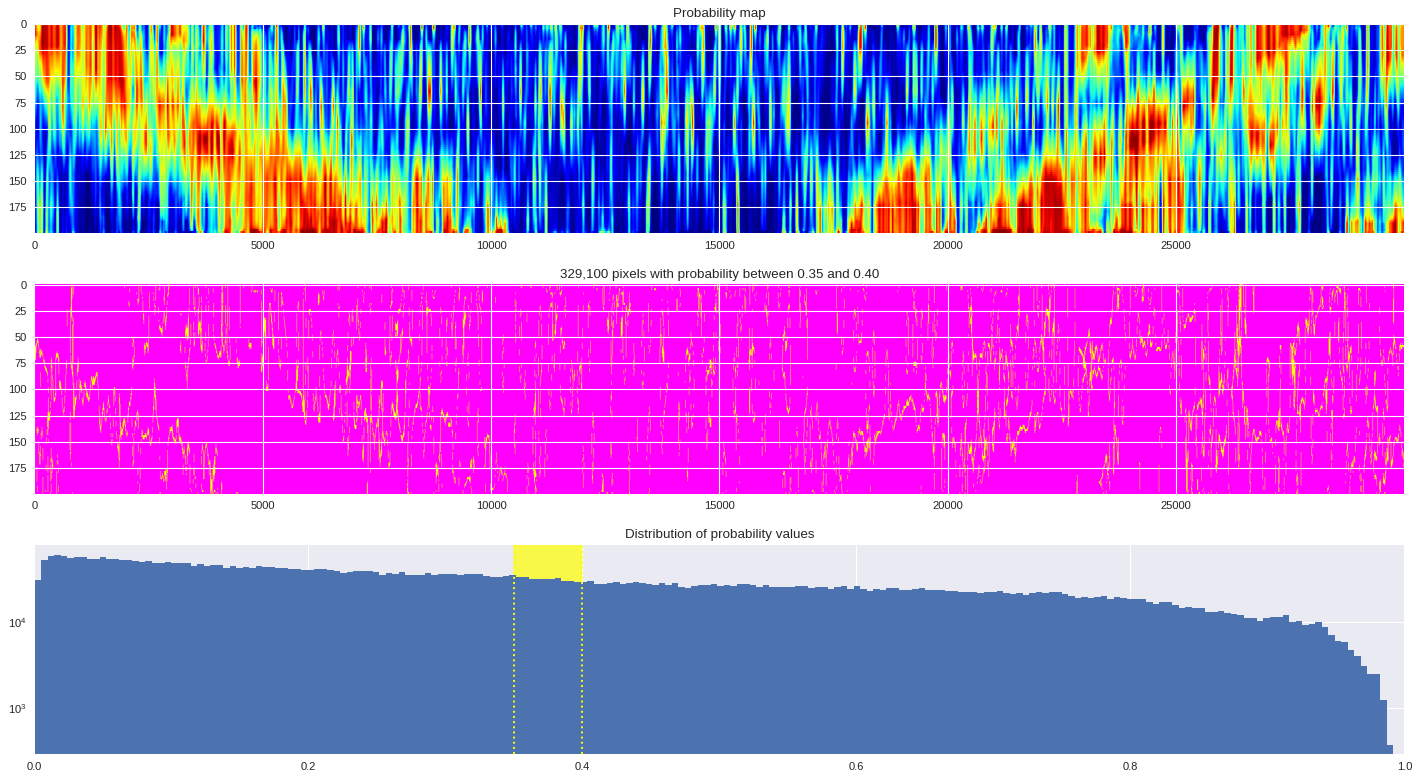
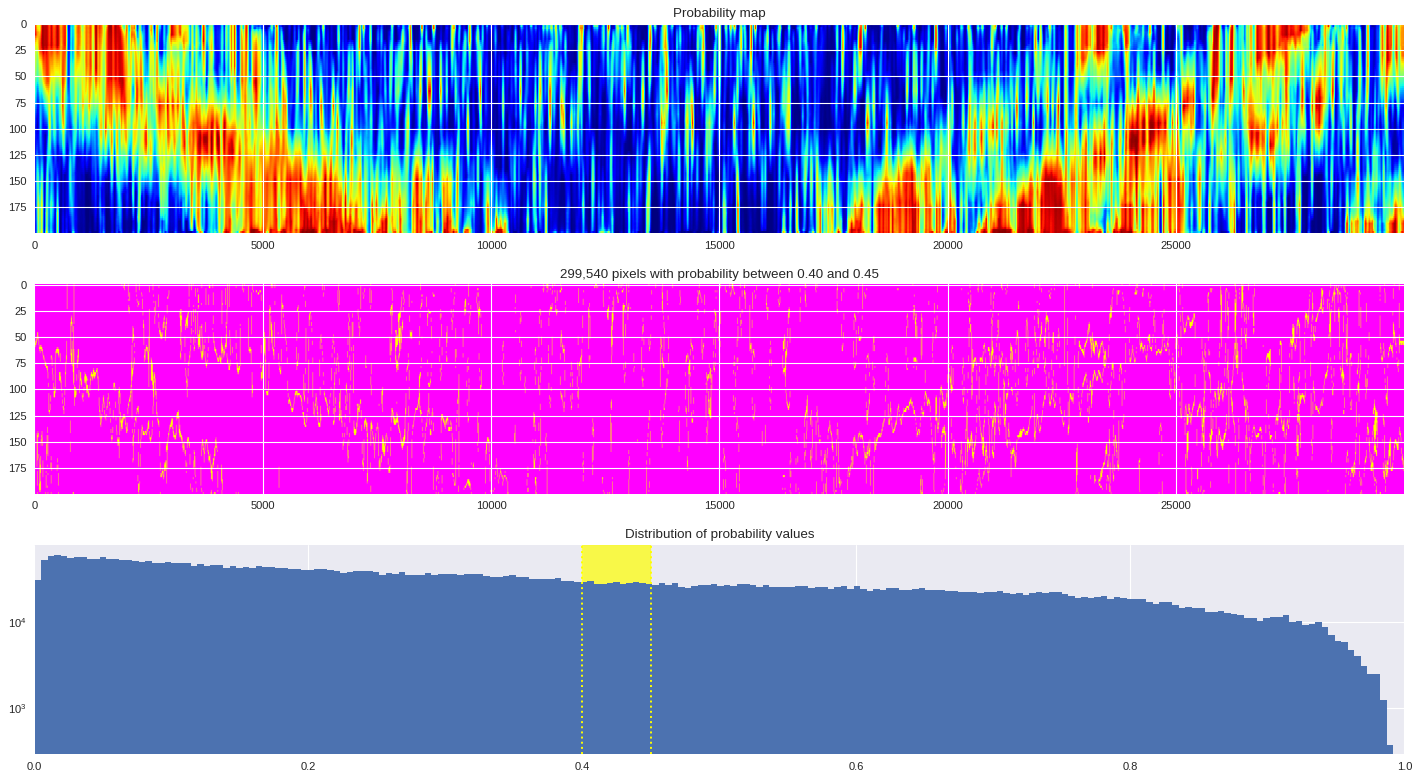
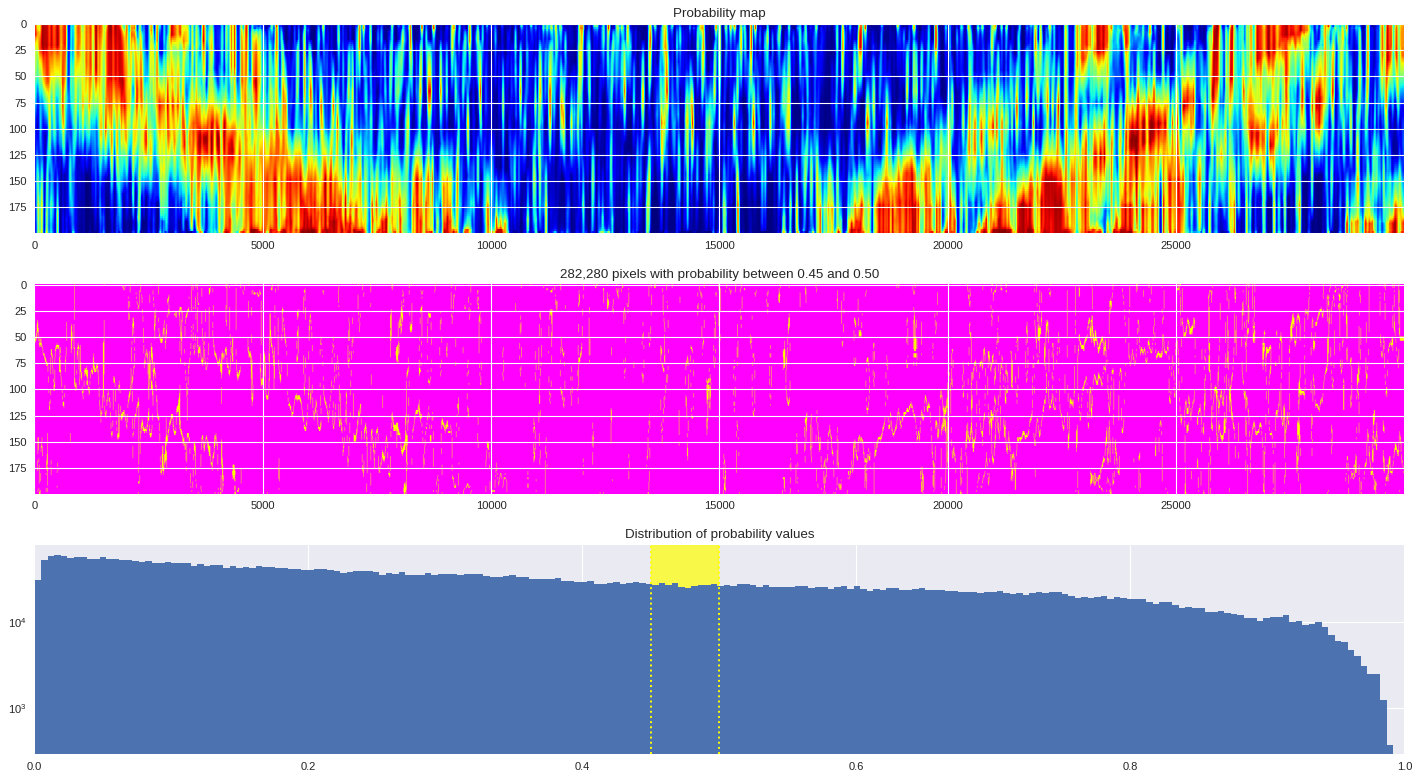
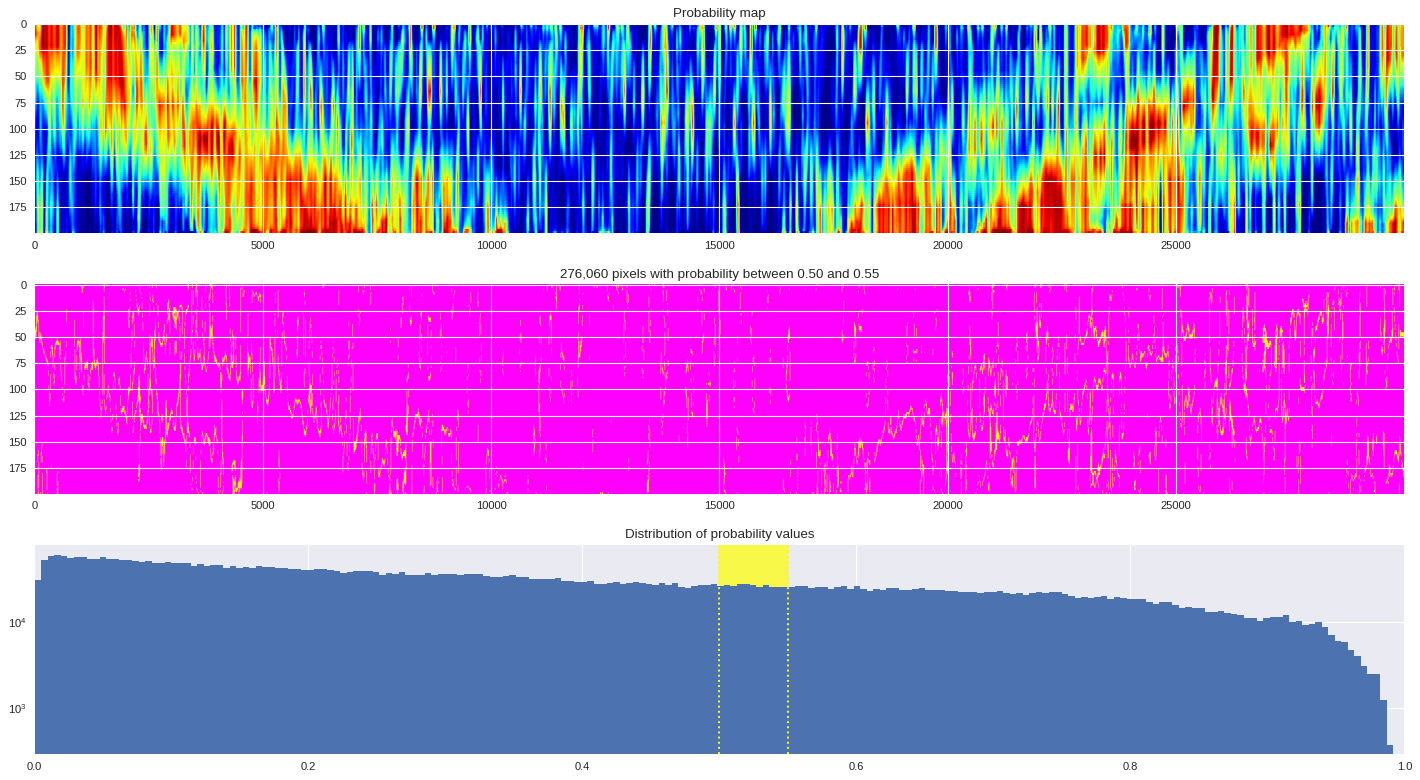
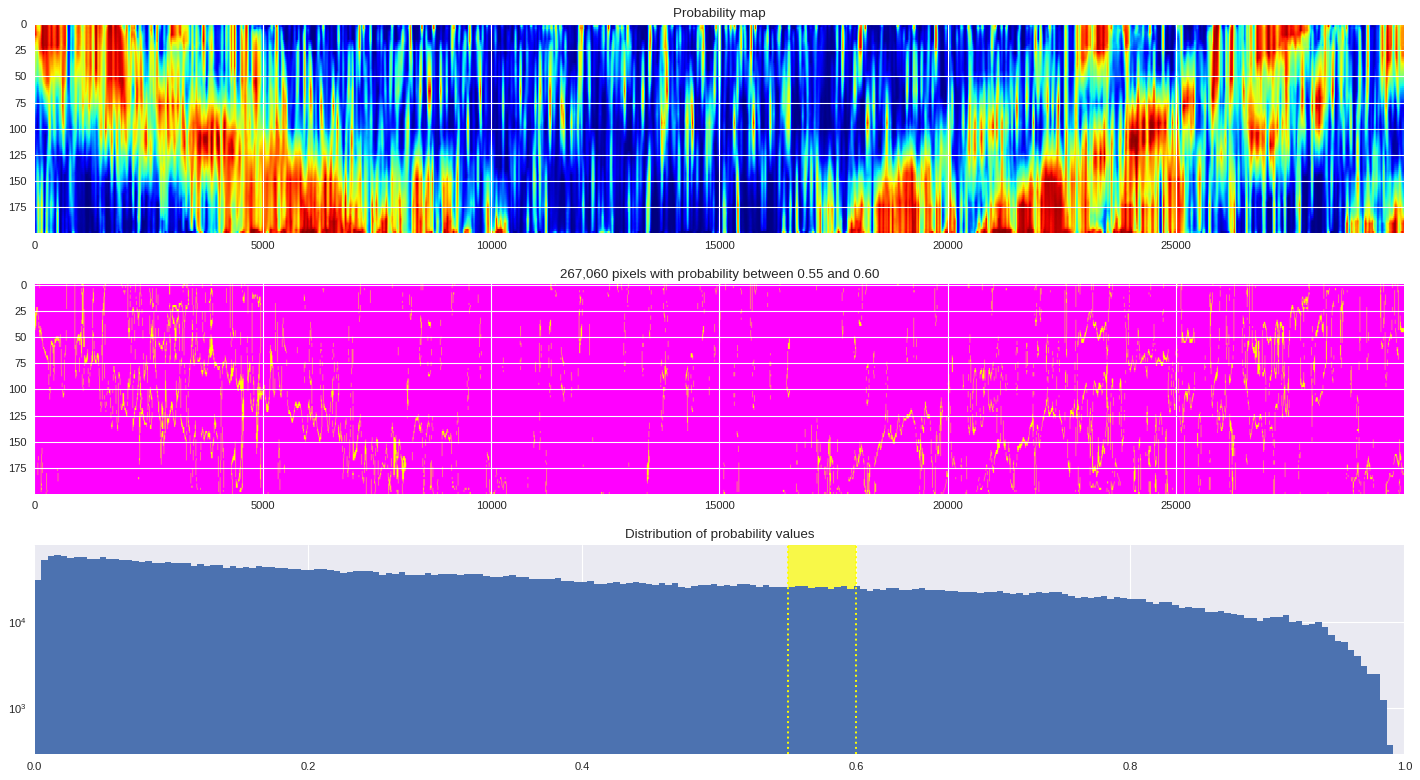
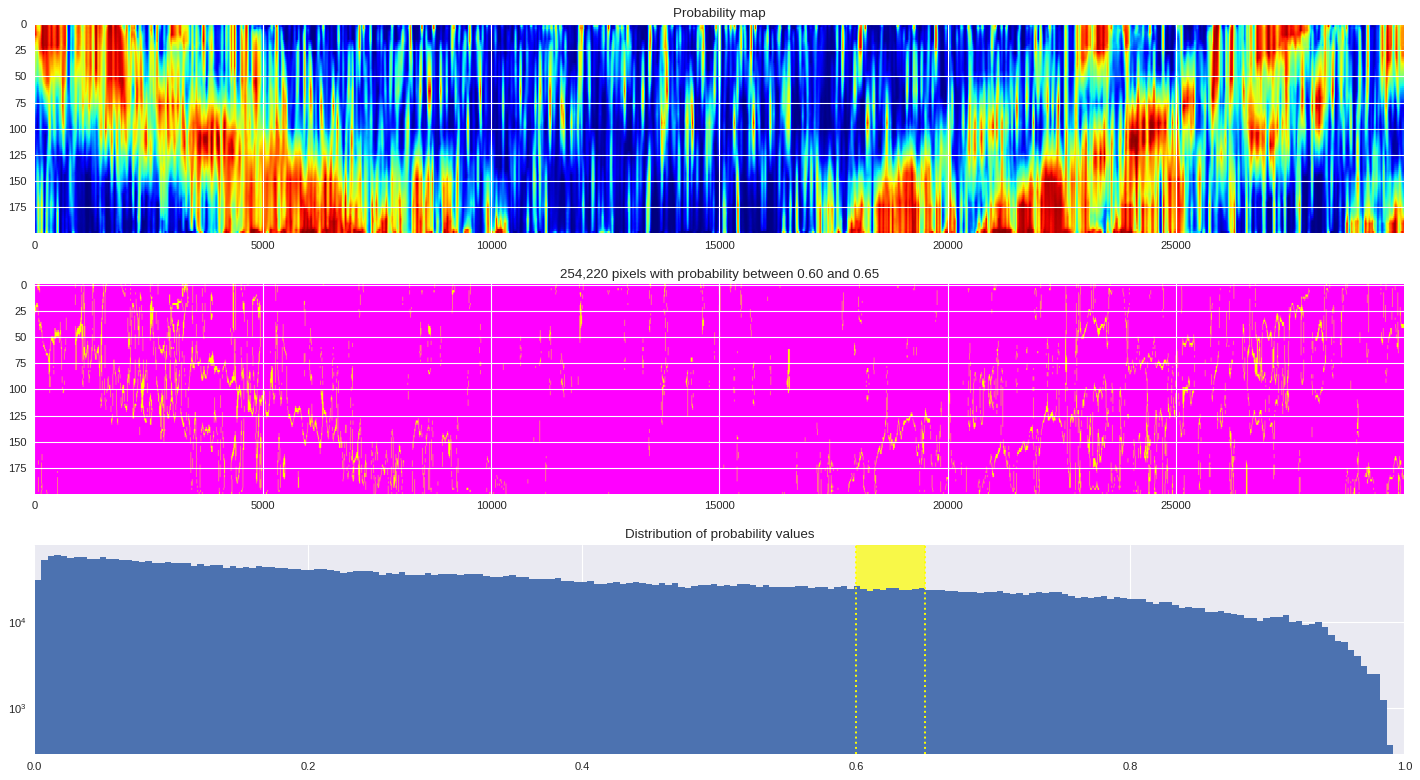
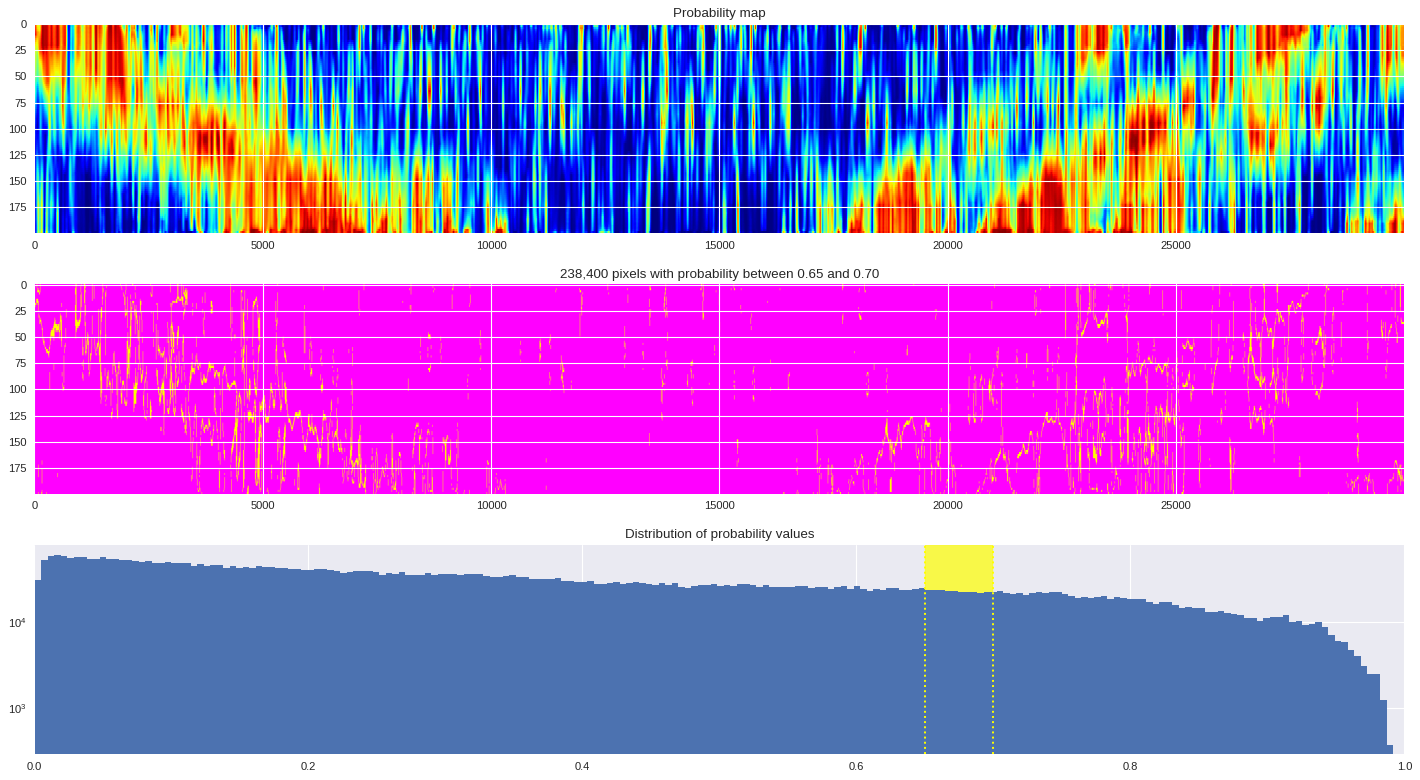
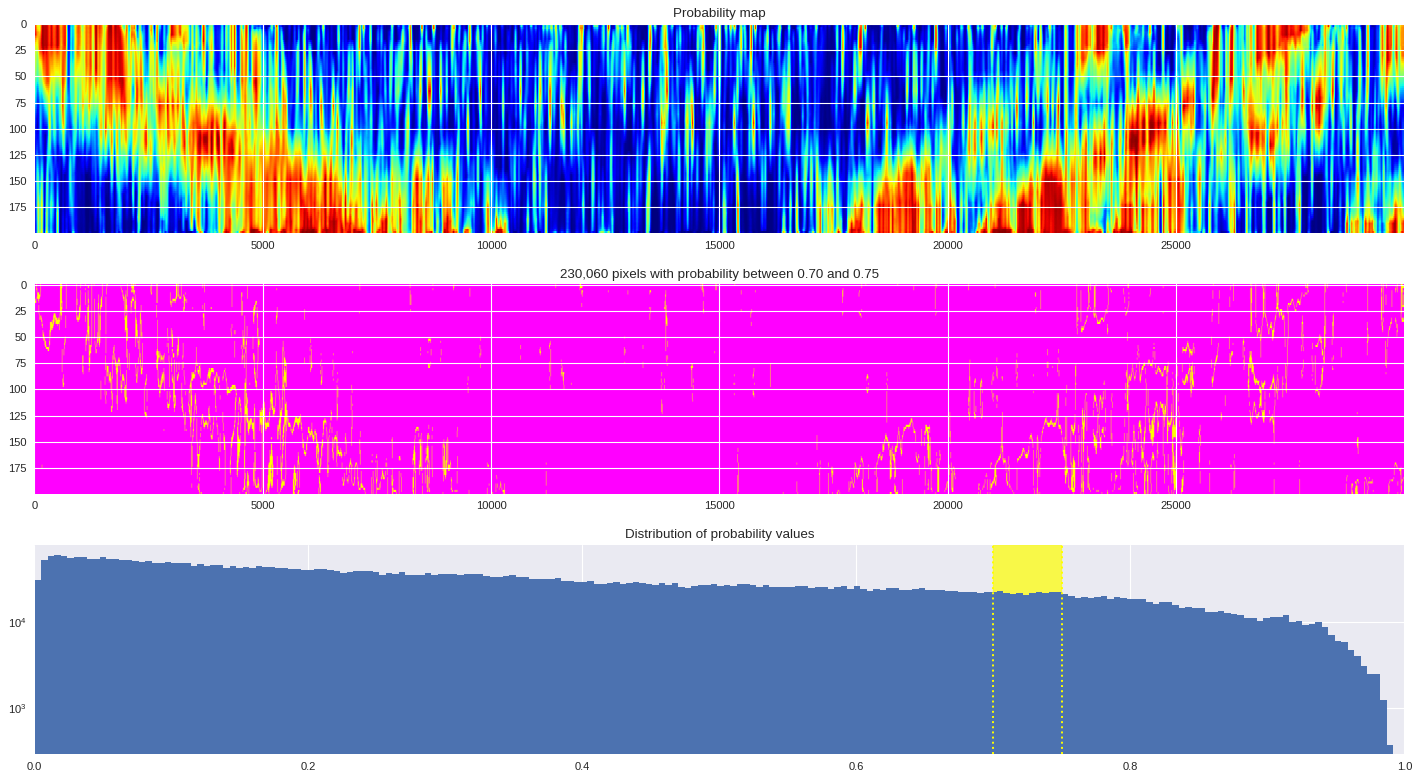
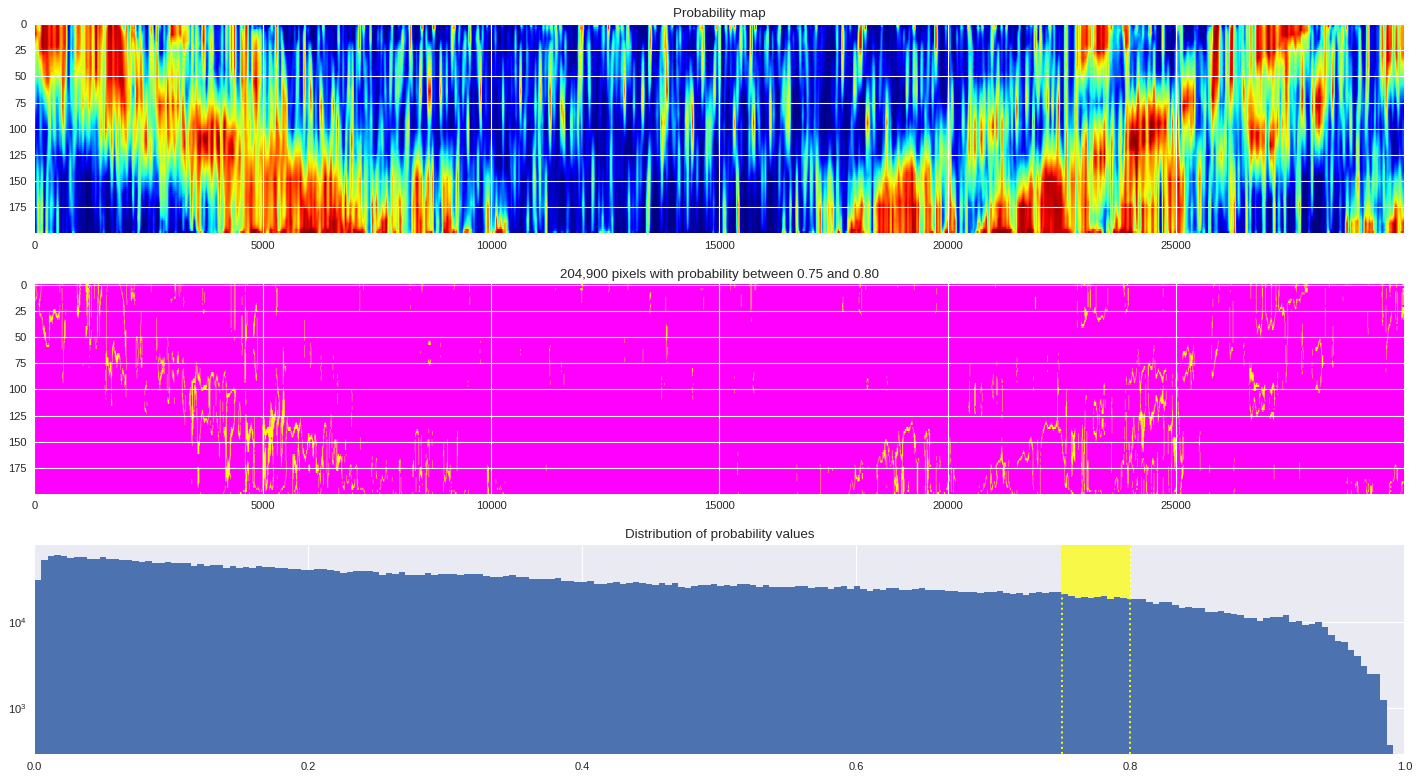
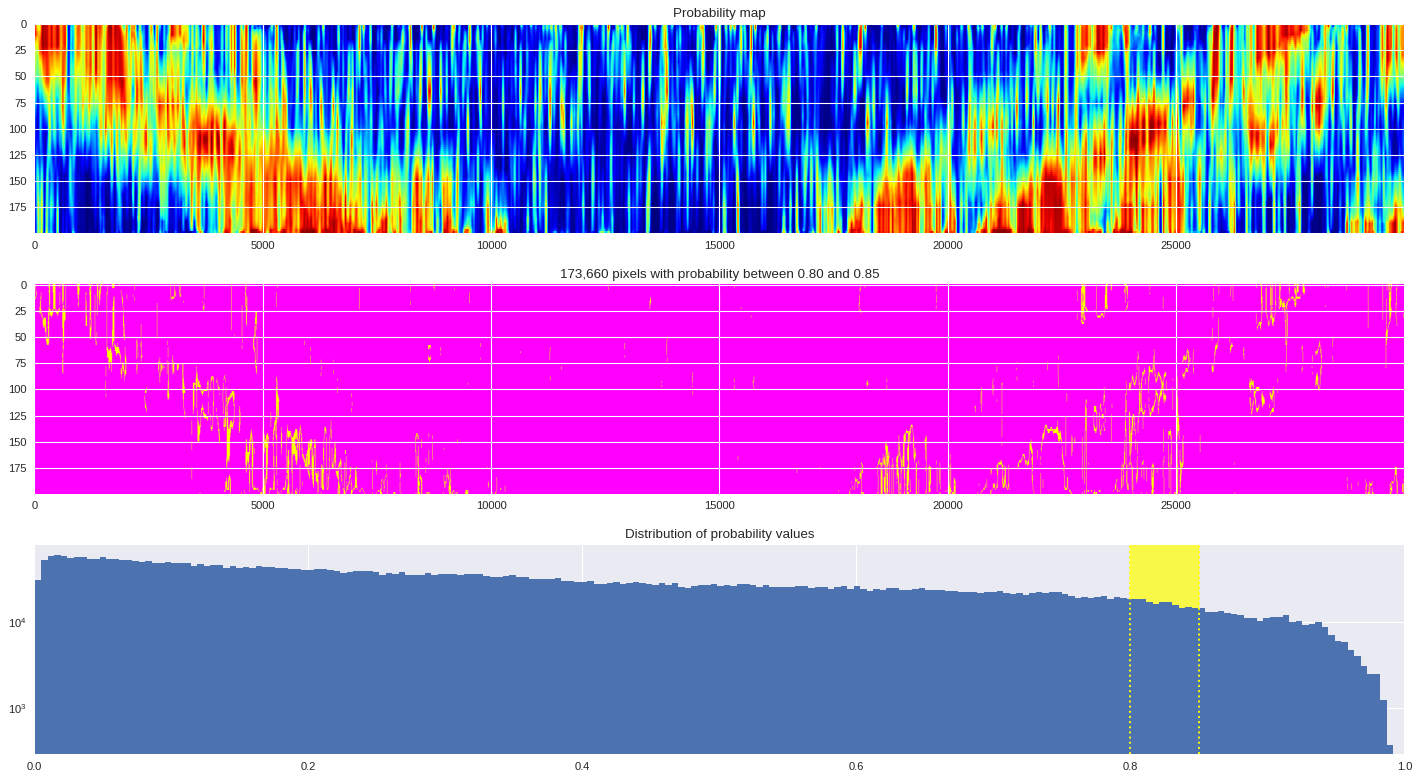
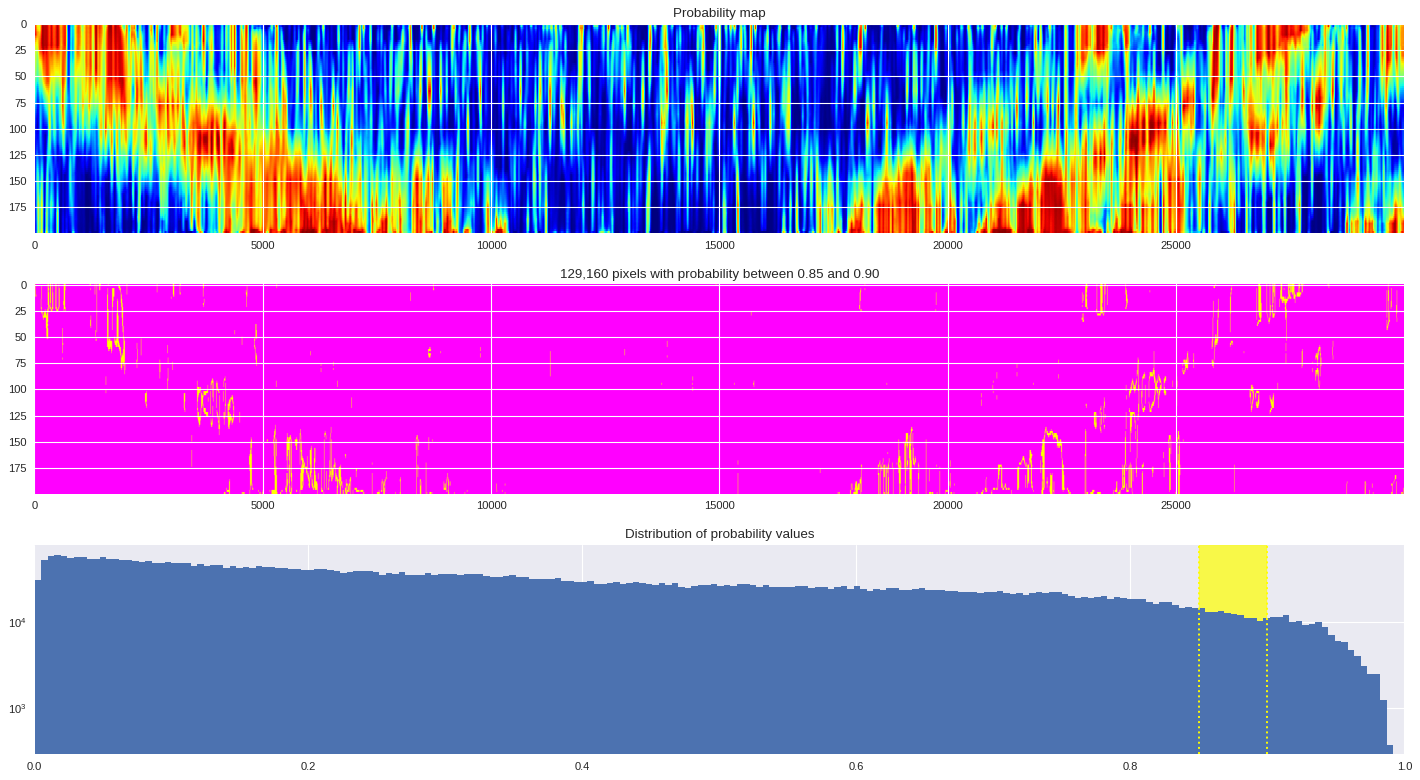
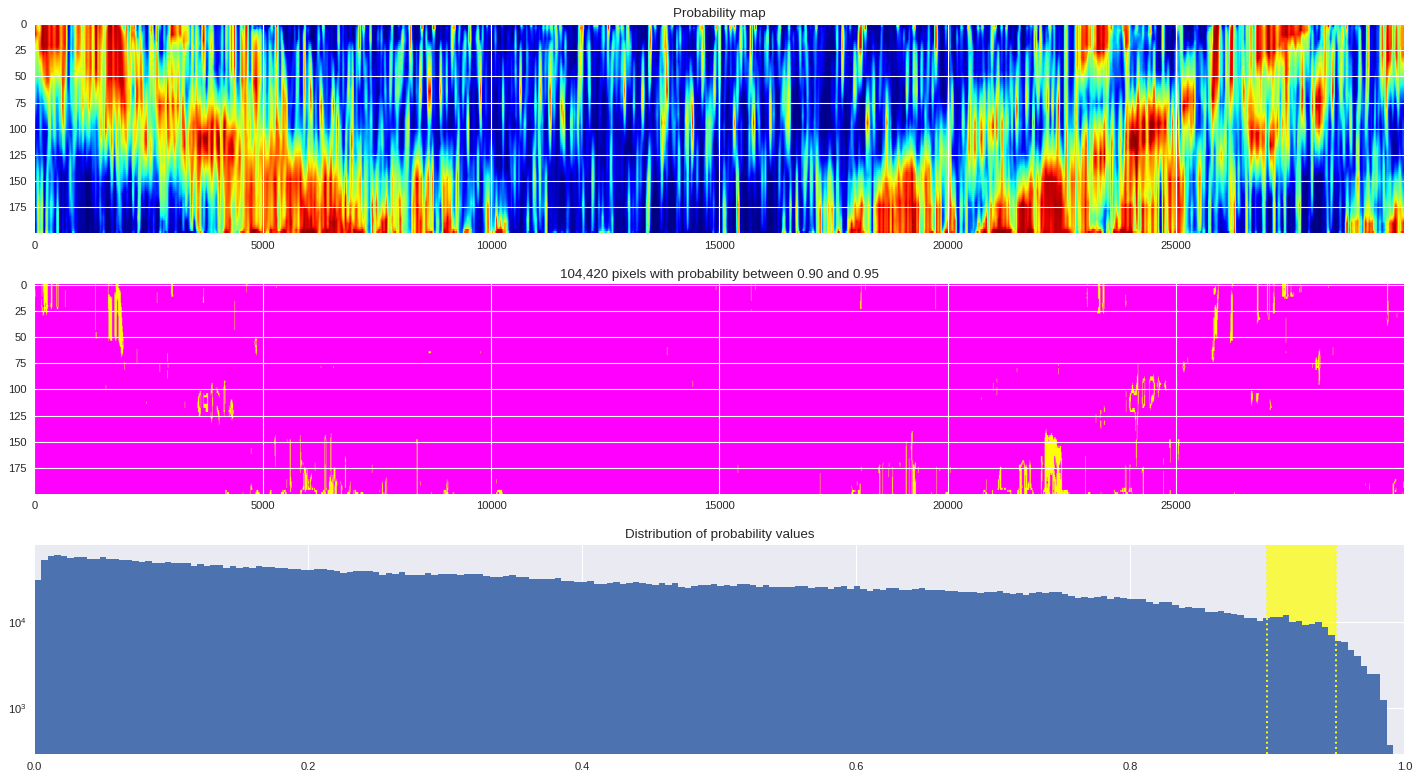
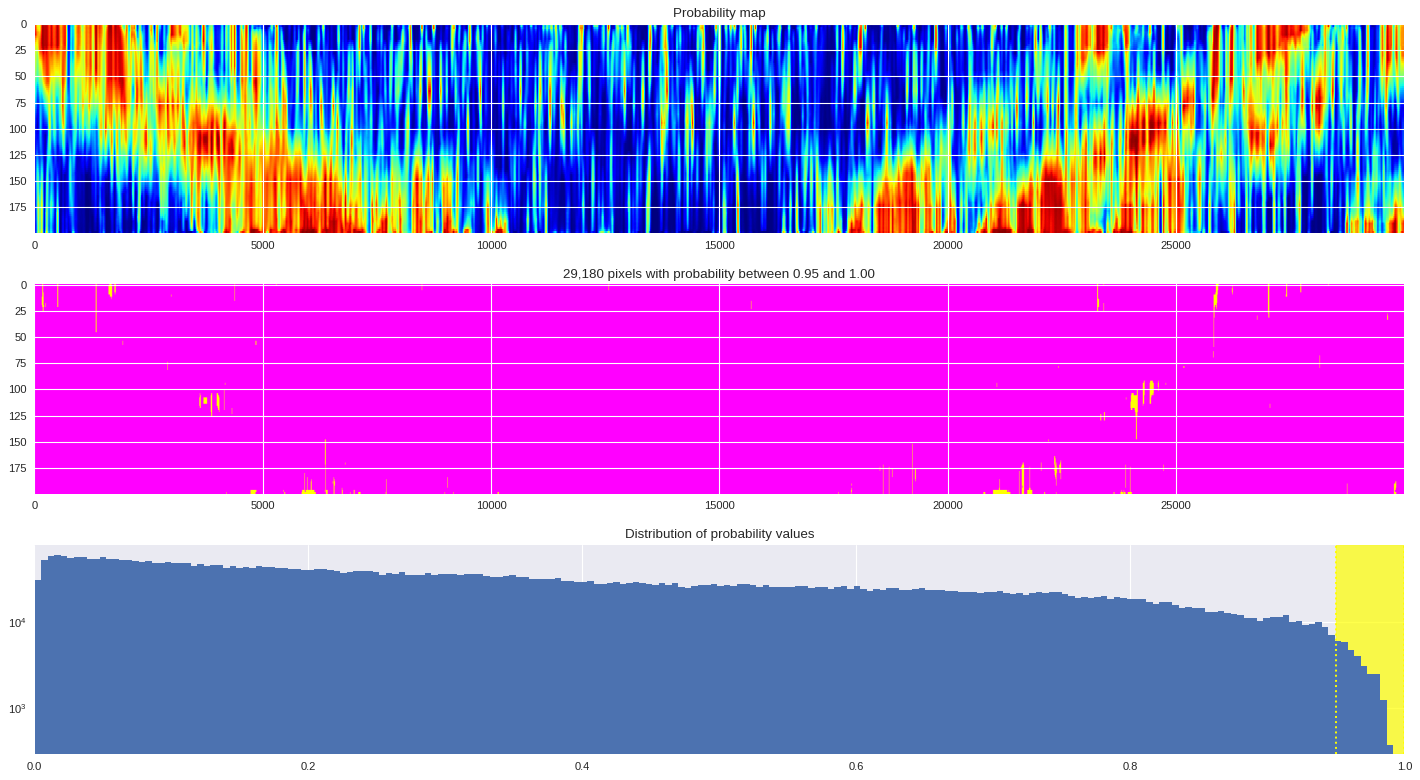
[ ]:
import re,glob,numpy,h5py
import matplotlib.pyplot as plt
from matplotlib.colors import LogNorm
# Initialize the figure
plt.style.use('seaborn')
fig,ax = plt.subplots(1,2,figsize=(18,5),dpi=80,sharex=True,sharey=True)
# Plot original image
imin,imax = 18000,20000
vmin = data[:,imin:imax].min()
vmax = data[:,imin:imax].max()
print(vmin,vmax)
ax[0].imshow(data[:,imin:imax],cmap='seismic',aspect='auto',vmin=vmin,vmax=vmax)
ax[0].set_title('Raw strain measurements')
# Plot probability map
ax[1].imshow(prob_map[:,imin:imax],cmap ='inferno',aspect='auto')
ax[1].set_title('Probability map')
plt.tight_layout()
plt.show()
-2006.0 1730.0
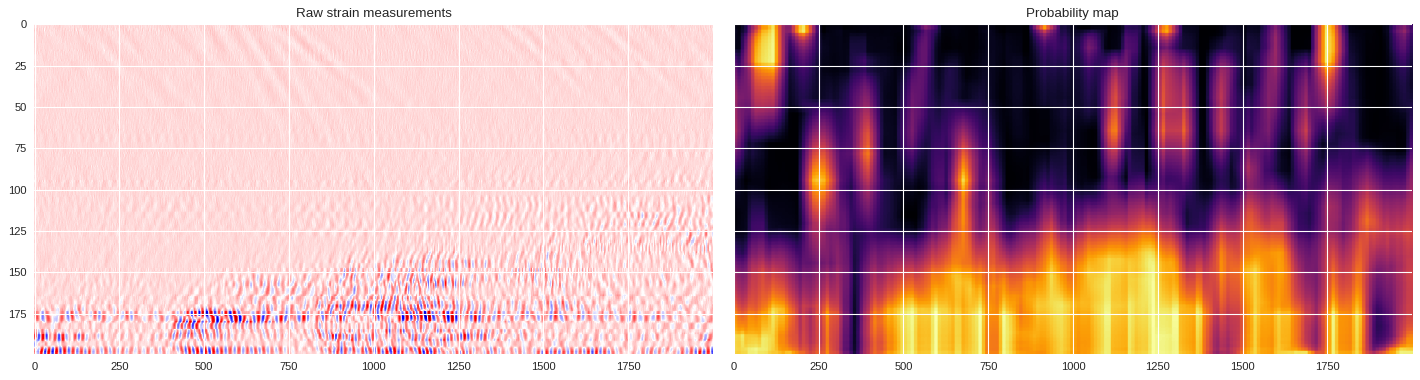
[ ]:
print(prob_map.min(),prob_map.max())
0.026501178741455078 0.9406810641288758
[ ]:
import matplotlib.pyplot as plt
from google.colab import widgets
div = 30
tb = widgets.TabBar([str(i) for i in range(div)])
for k in range(div):
with tb.output_to(k, select=False):
imin,imax = k*data.shape[1]//div,(k+1)*data.shape[1]//div
plt.style.use('seaborn')
plt.figure(figsize=(18,6),dpi=80)
ax1 = plt.subplot(121)
ax1.imshow(data[:,imin:imax],extent=[imin/500,imax/500,200,0],aspect='auto',cmap='seismic')
ax1.set_title('Raw data')
ax1.set_xlabel('Time [second]')
ax1.set_ylabel('Channels')
ax2 = plt.subplot(122,sharex=ax1,sharey=ax1)
print(prob_map[:,imin:imax].min(),prob_map[:,imin:imax].max())
ax2.imshow(prob_map[:,imin:imax],extent=[imin/500,imax/500,200,0],aspect='auto',cmap='jet',vmin=0,vmax=1)
ax2.set_title('Probability map')
ax2.set_xlabel('Time [second]')
plt.tight_layout()
plt.show()
0.026501178741455078 0.9406810641288758
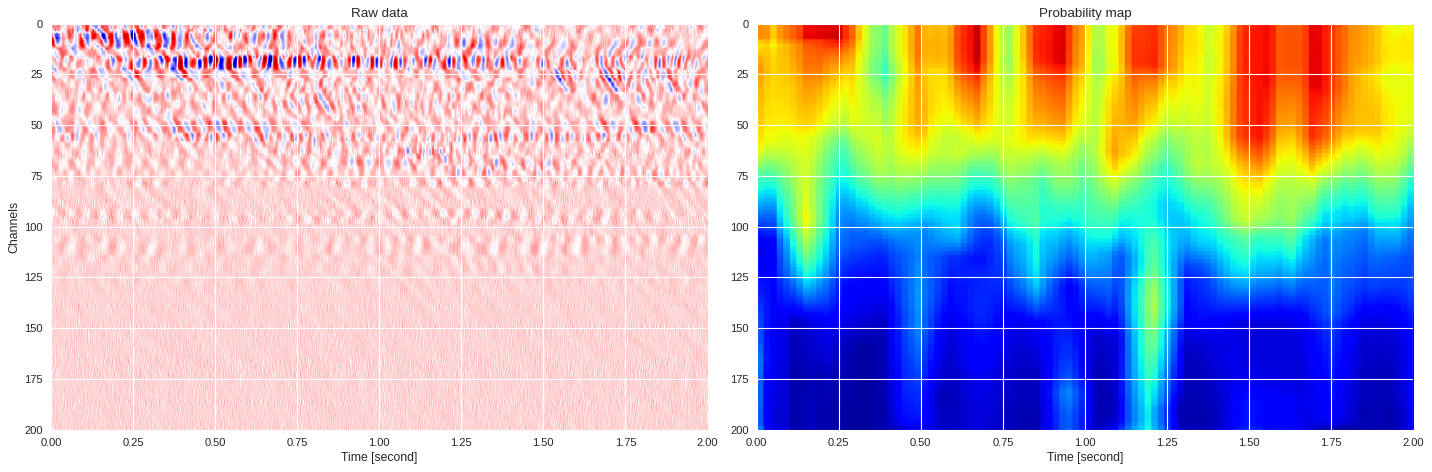
0.04205800096193949 0.9188275518871489
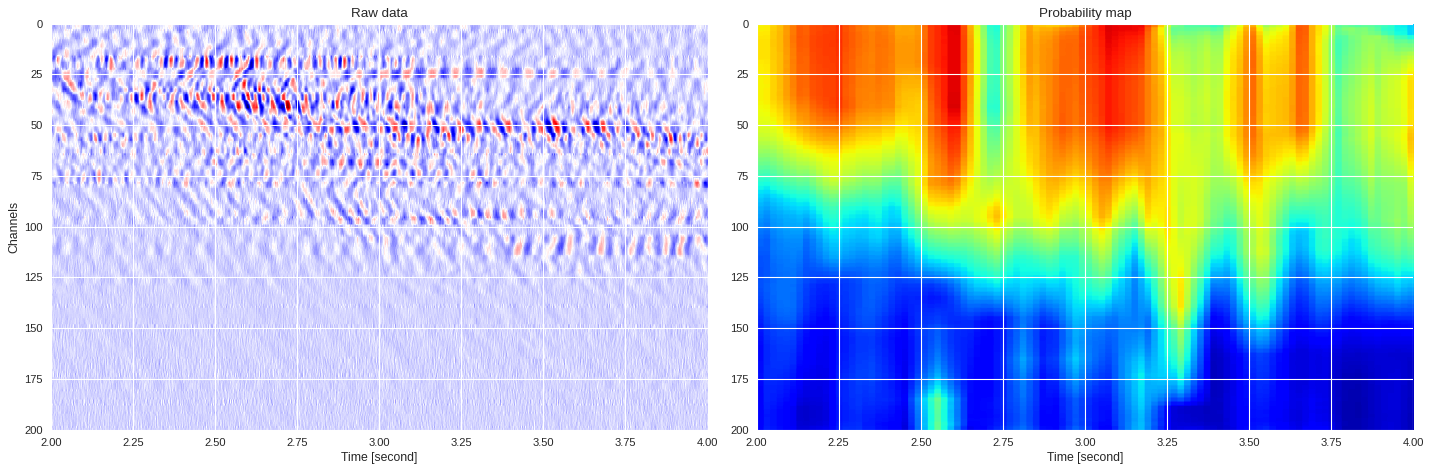
0.036908960342407225 0.8789216980934144
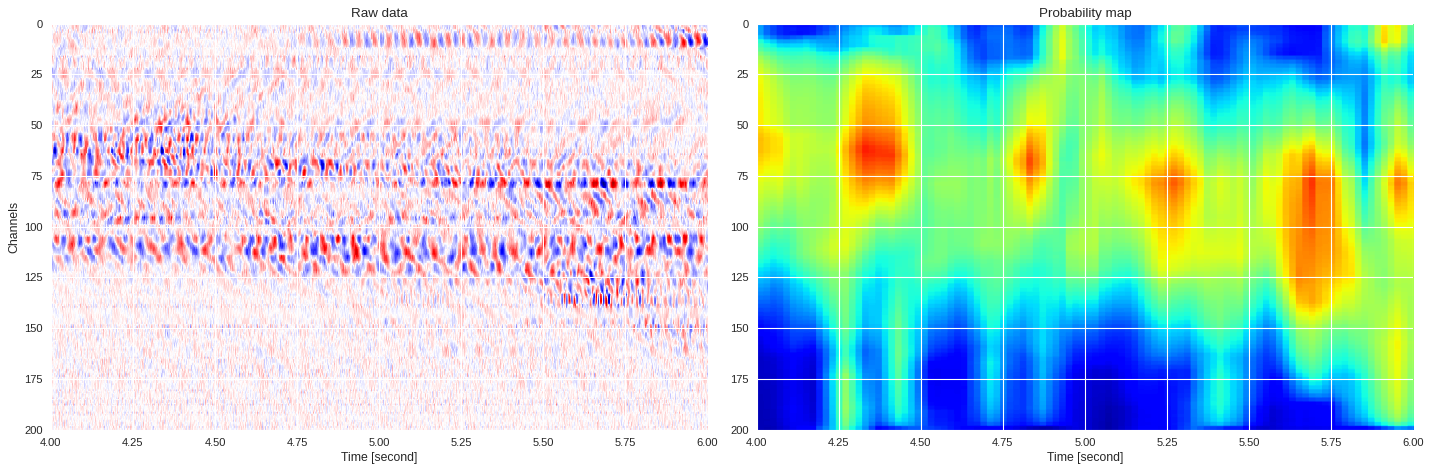
0.12865116596221923 0.9292599287033081
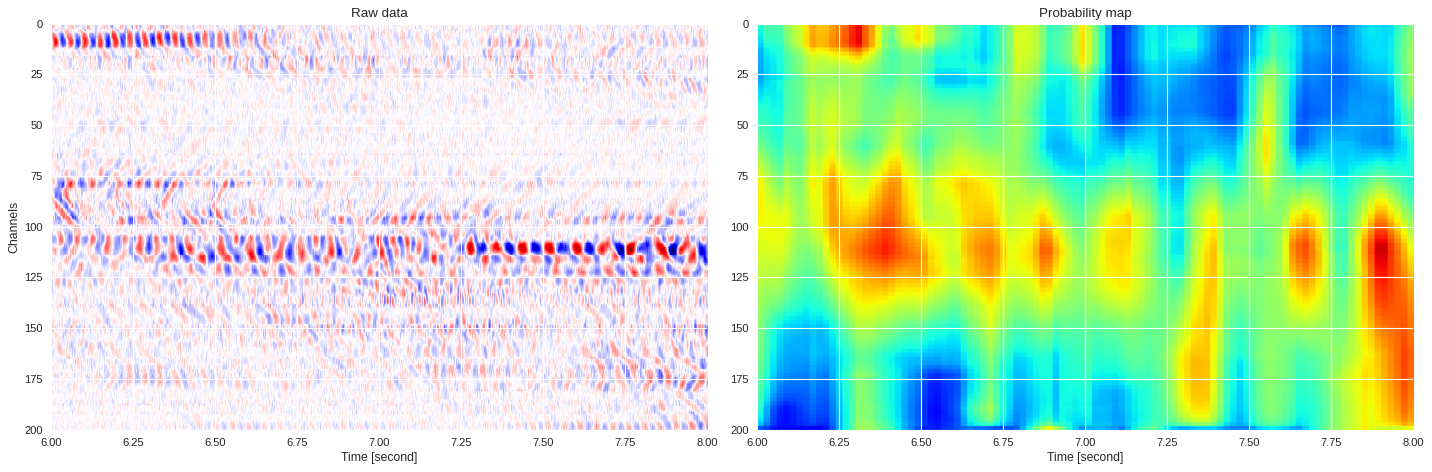
0.11686584949493409 0.8886327028274537
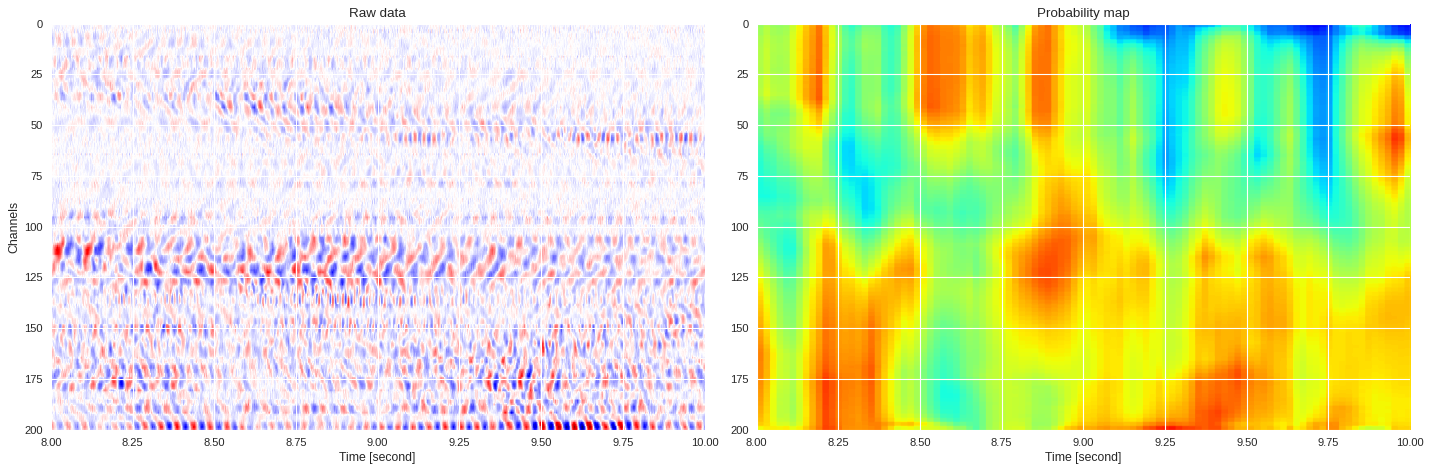
0.06290048360824585 0.8504917621612549
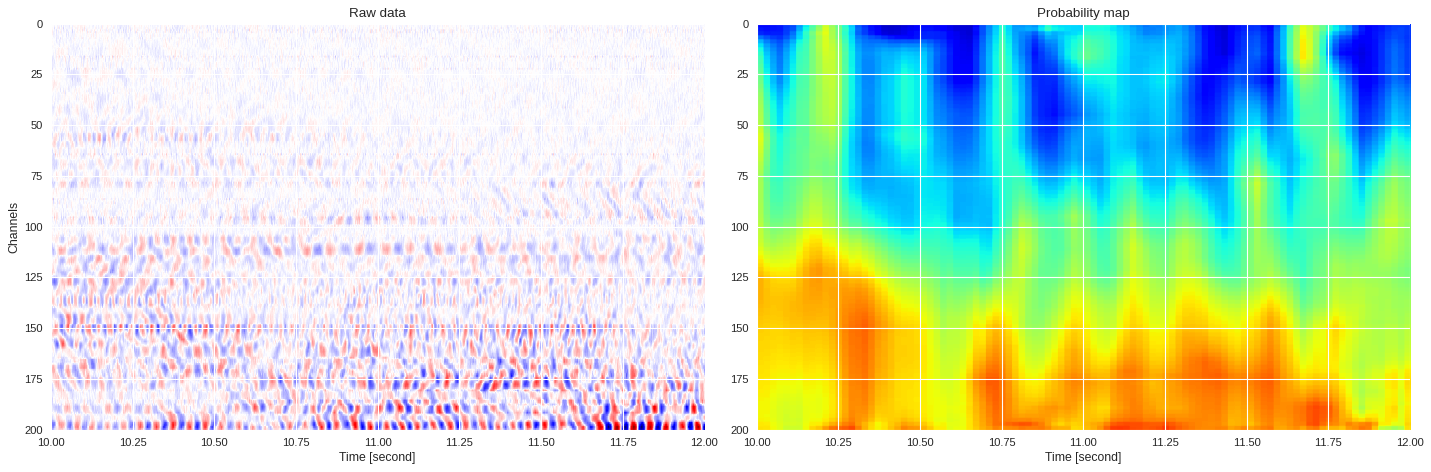
0.05163919925689697 0.9354328870773315
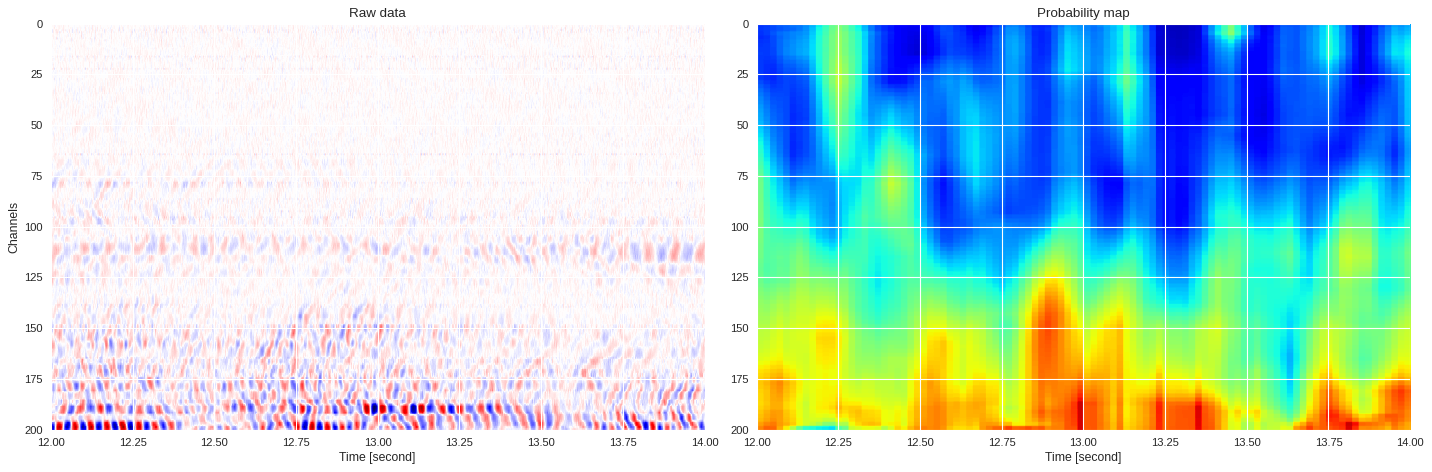
0.042815746579851426 0.8554190802574158
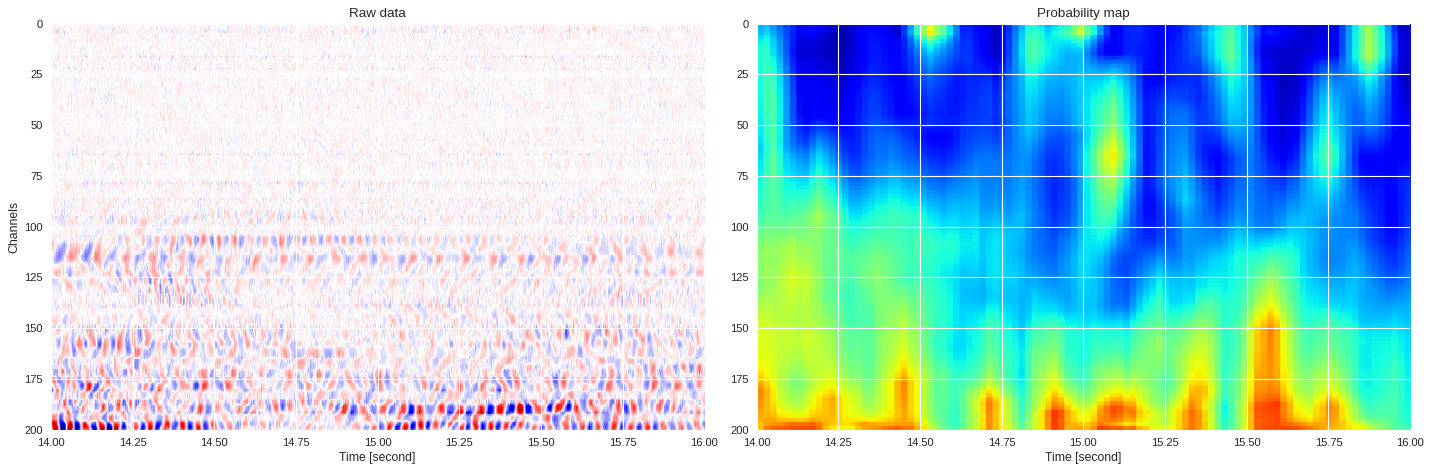
0.053783762454986575 0.7786331137021383
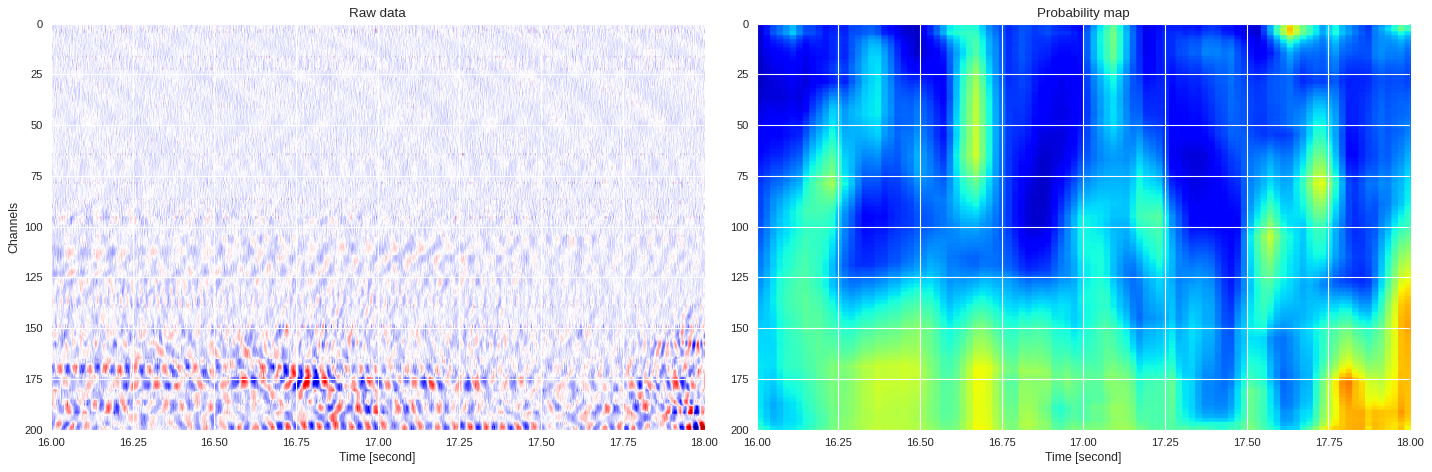
0.04316824674606323 0.7742297887802124
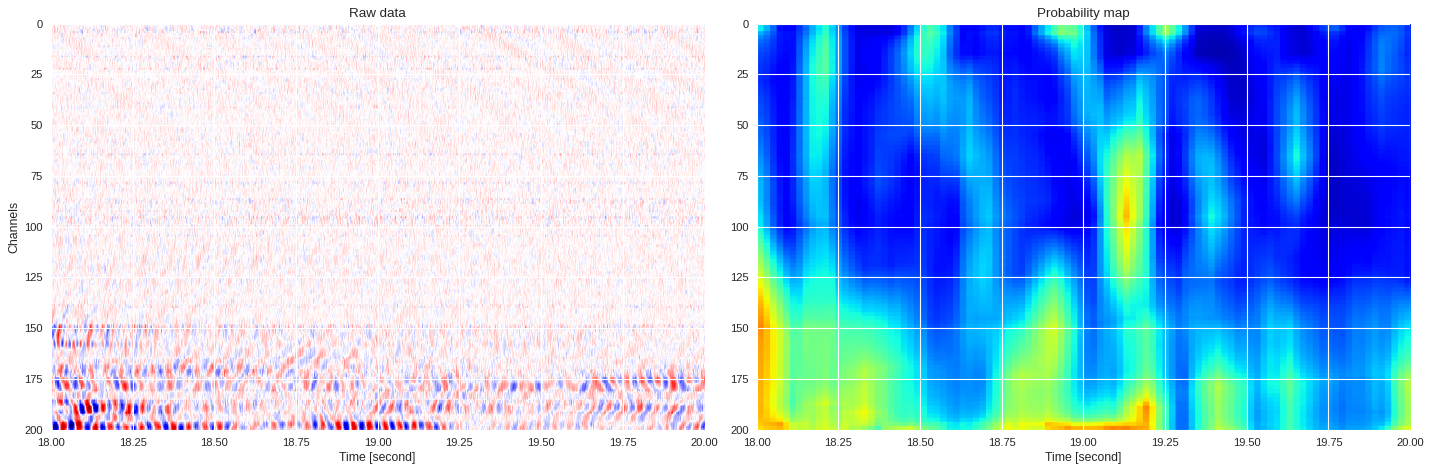
0.058214285850524905 0.7859080448746681
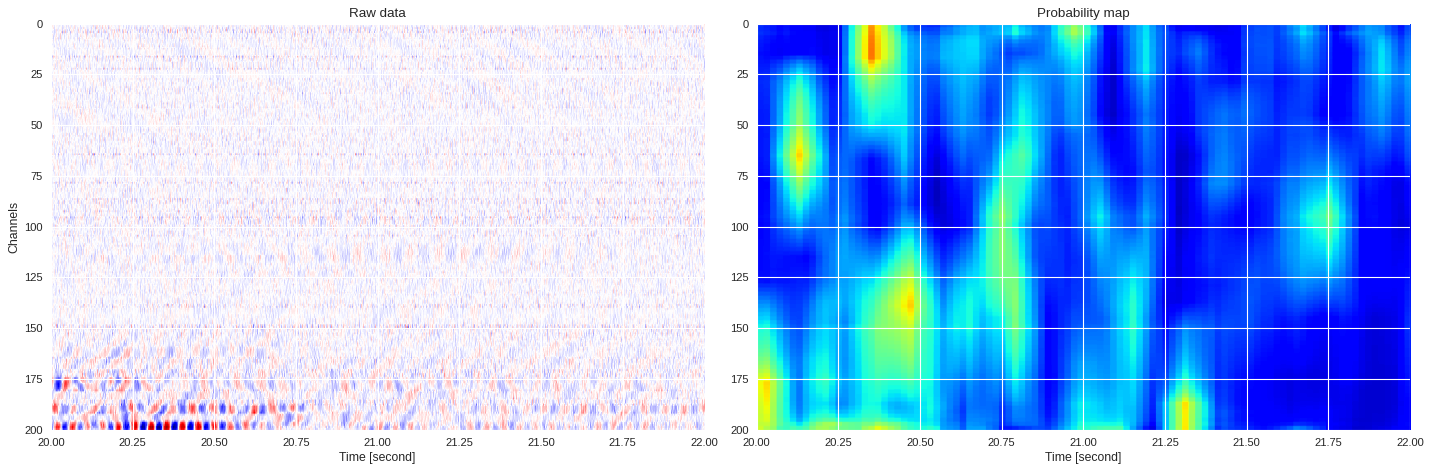
0.036785995960235594 0.6934613747596741
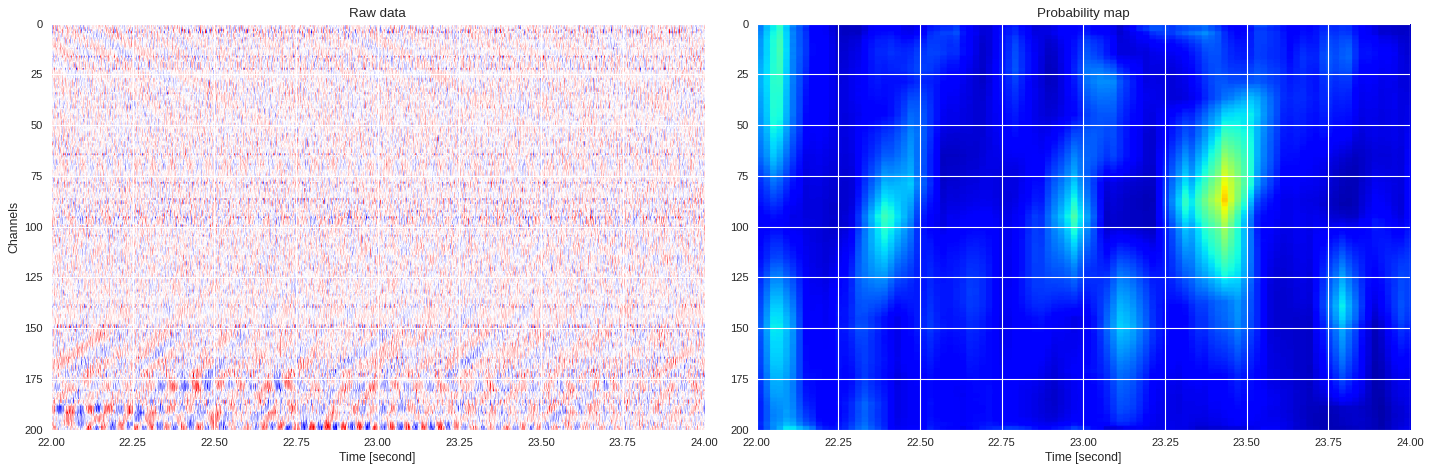
0.037767635451422796 0.6872830629348755
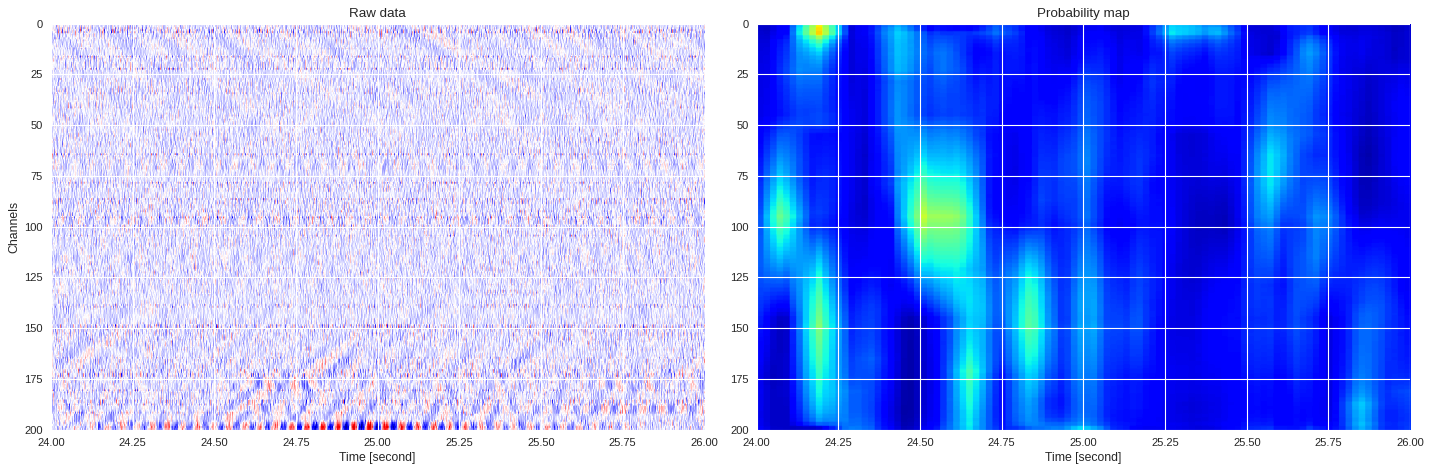
0.028156962394714356 0.5972896148761113
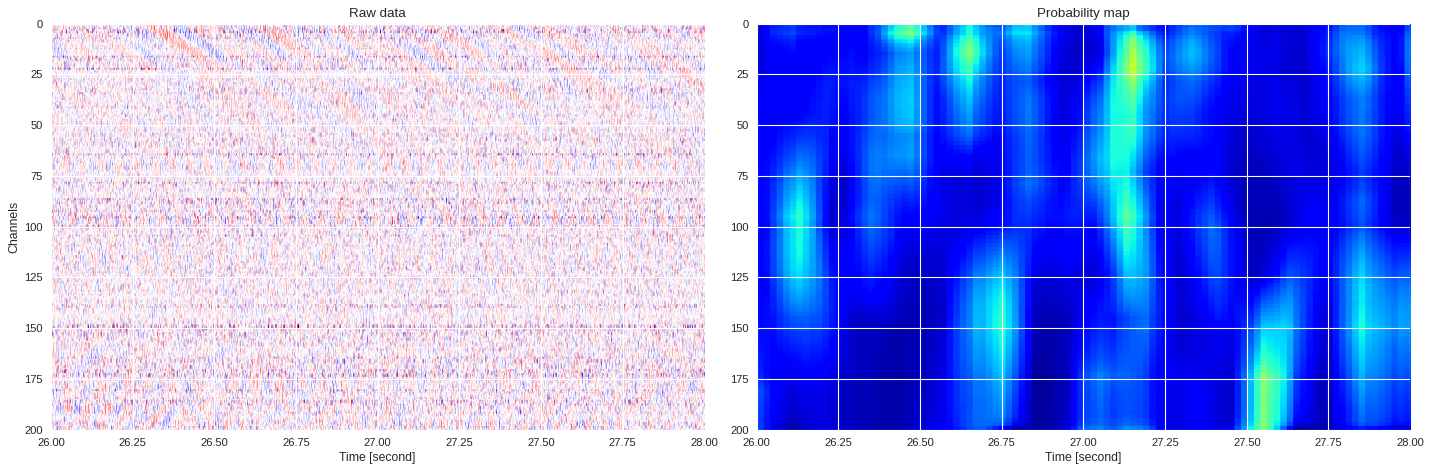
0.046036791801452634 0.7042534132798512
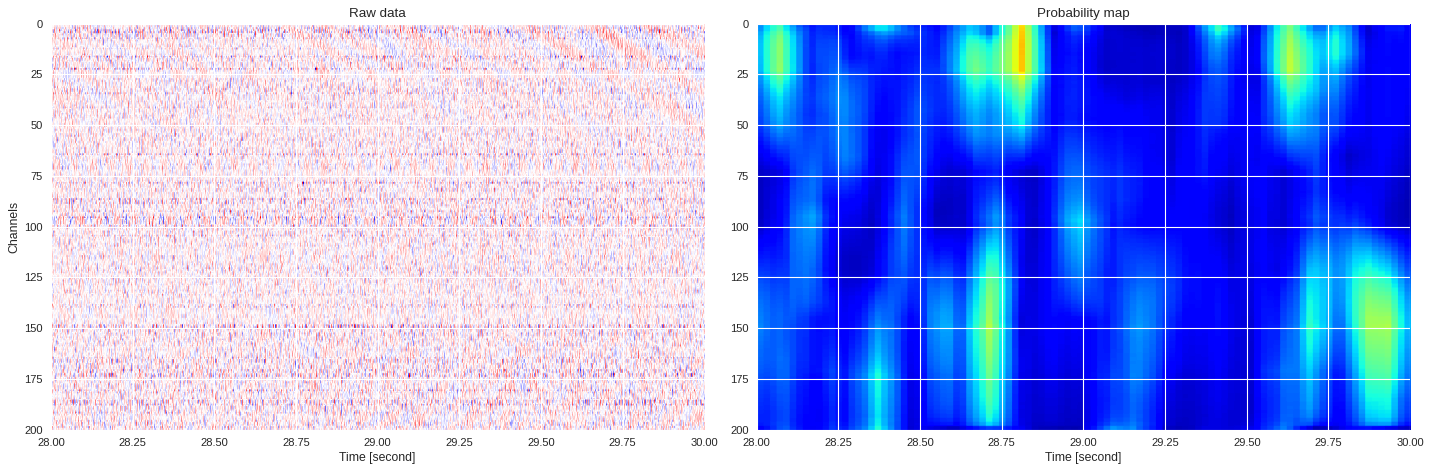
0.03259751796722412 0.7594619592030843
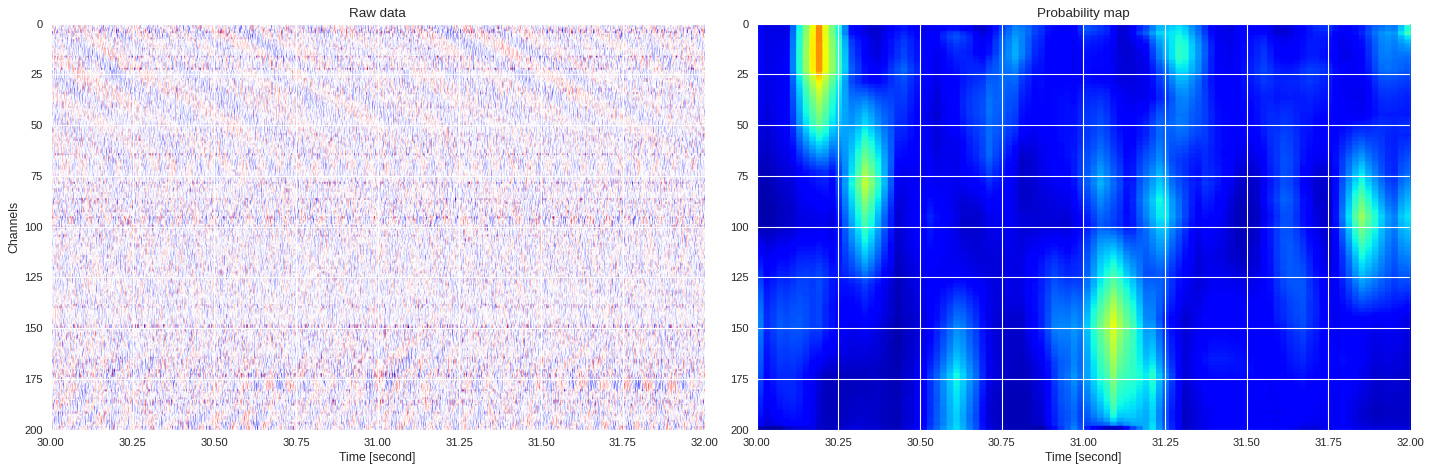
0.029248291969299317 0.6593878712654114
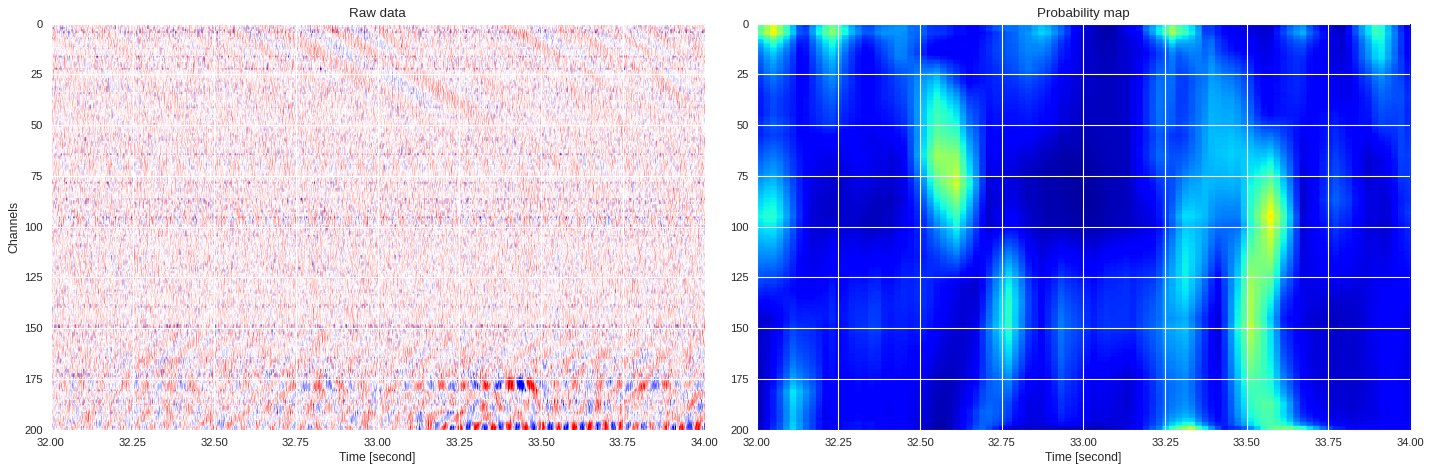
0.04270481765270233 0.7306549668312072
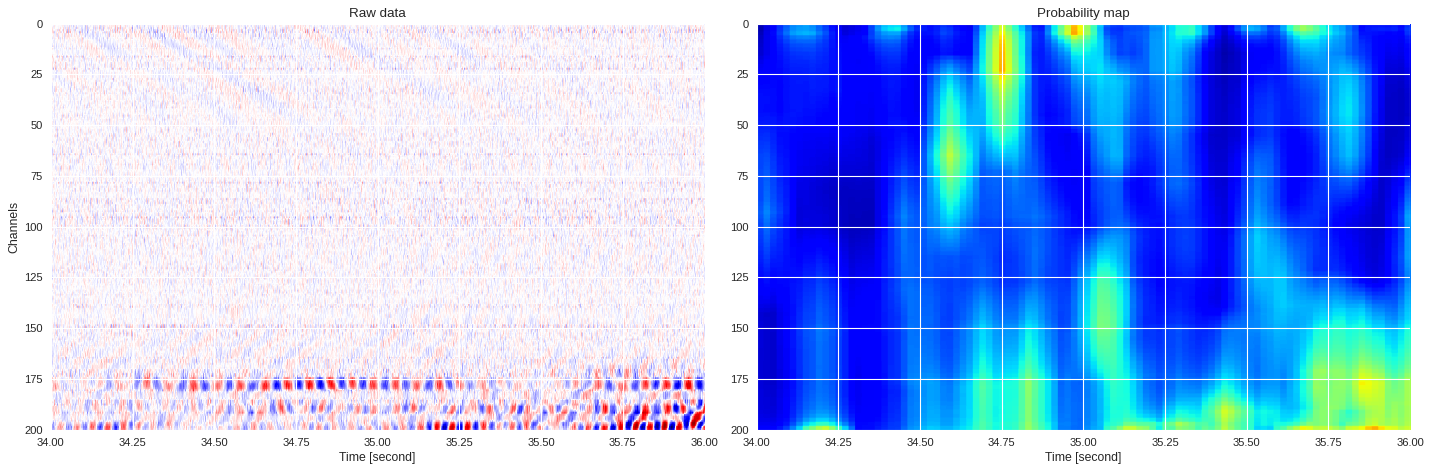
0.04135497093200684 0.8541004647811253
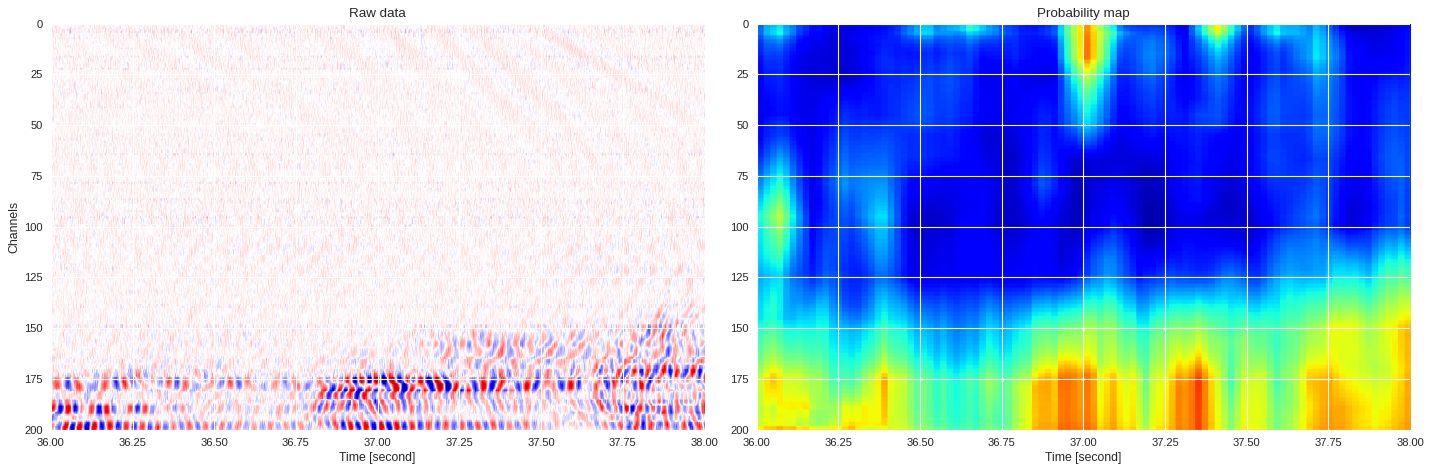
0.05462758541107178 0.9011522311430711
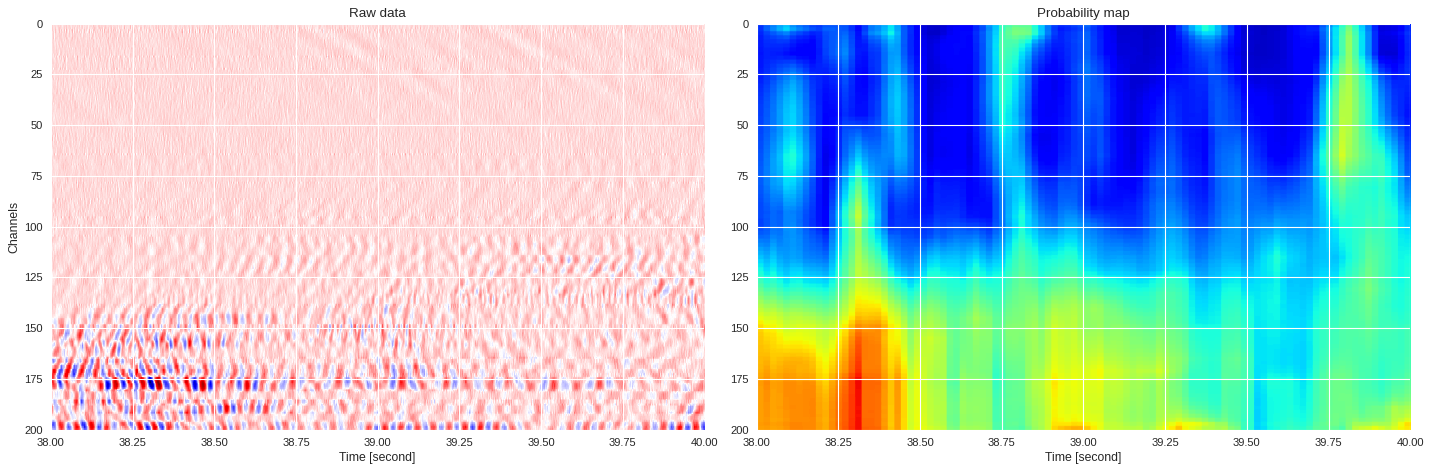
0.03655888438224793 0.885790283203125
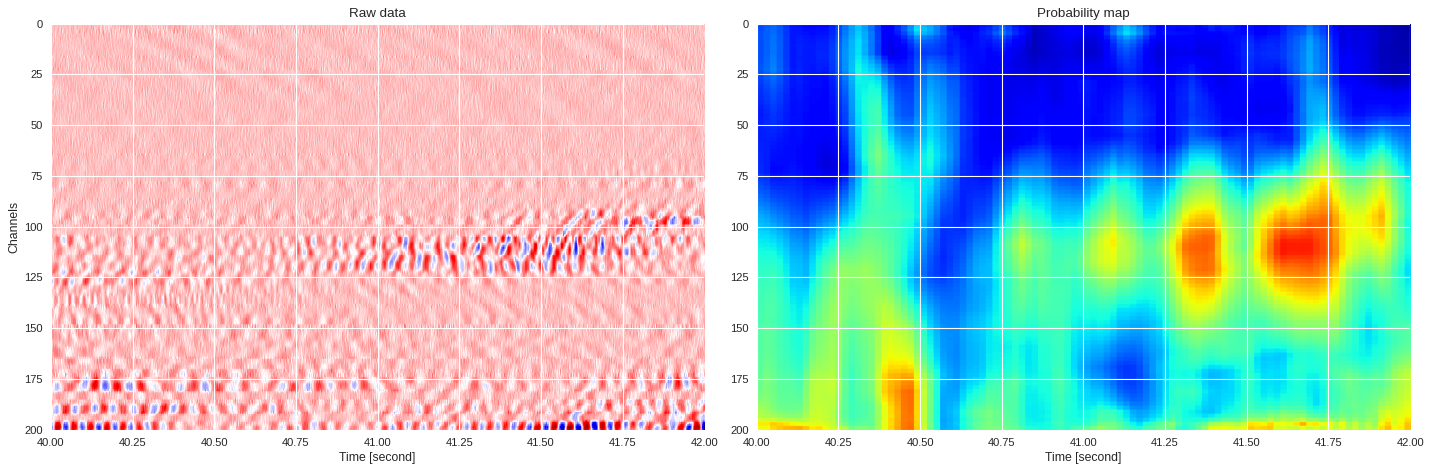
0.034424221515655516 0.9211421196277325
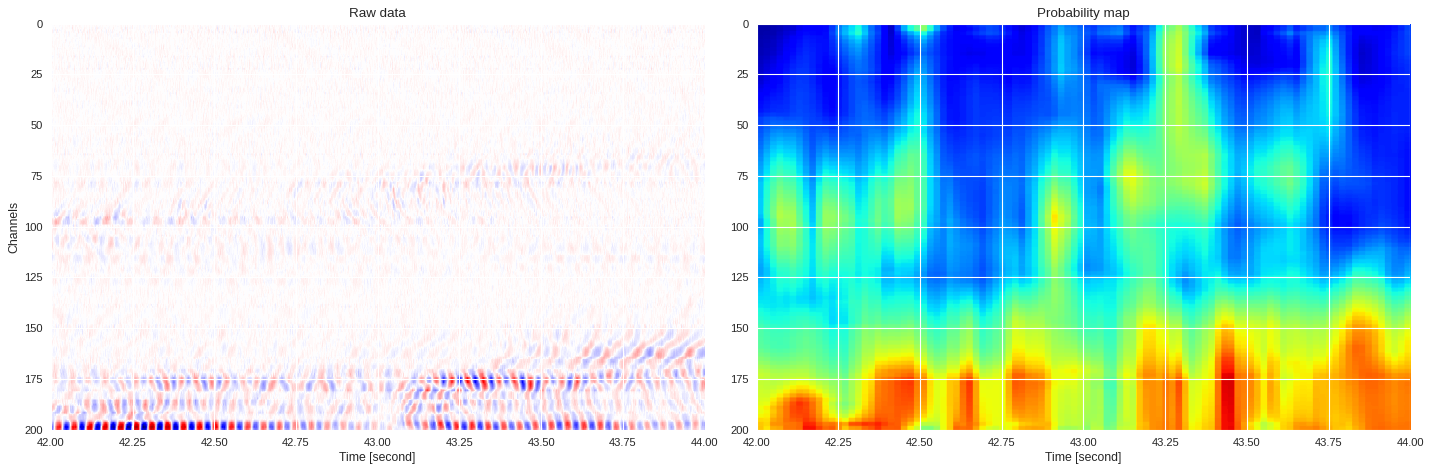
0.10099855661392212 0.8727228283882141
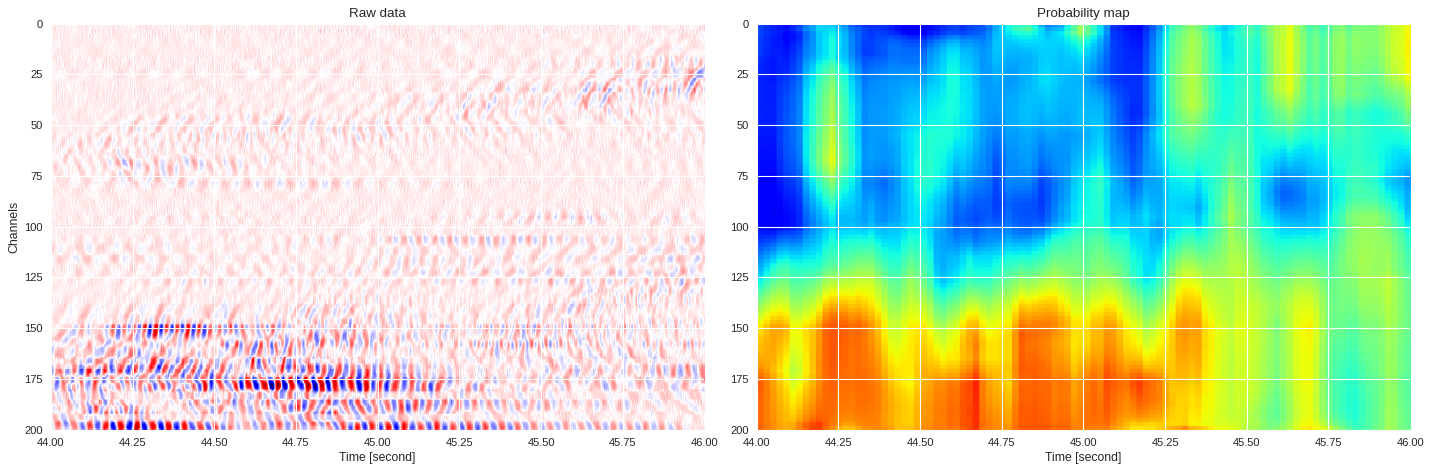
0.1754660539627075 0.9021974219216241
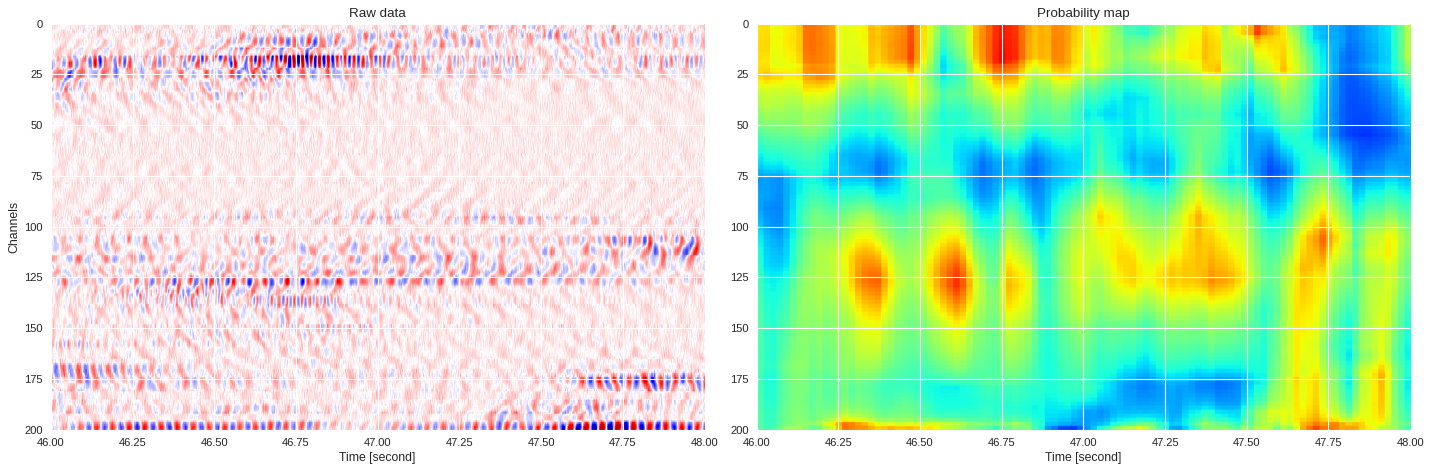
0.10816686898469925 0.929409924030304
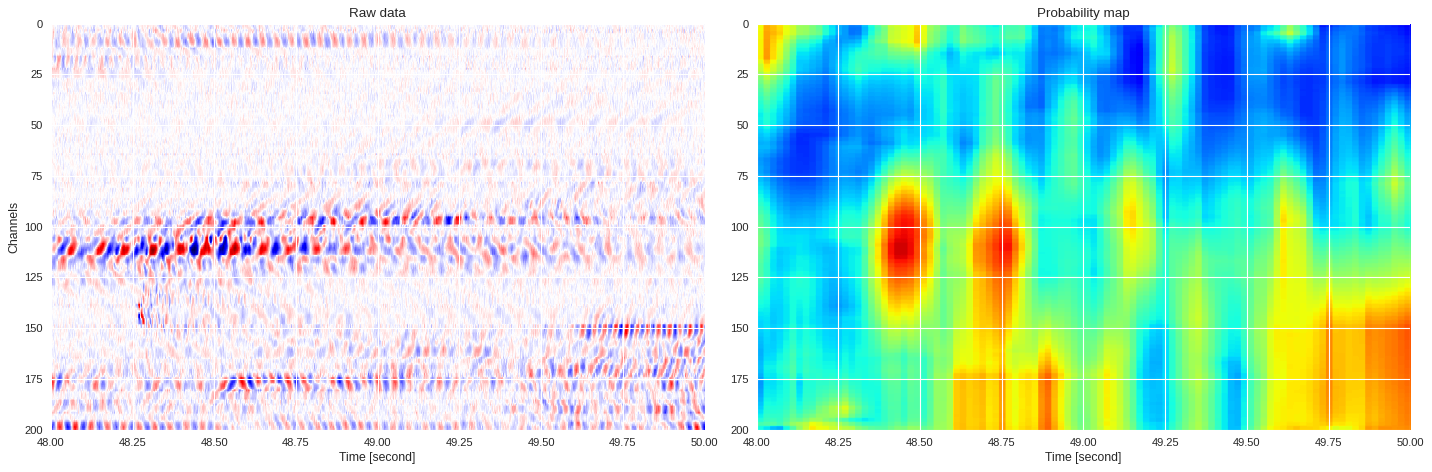
0.05496131181716919 0.8446513619422913
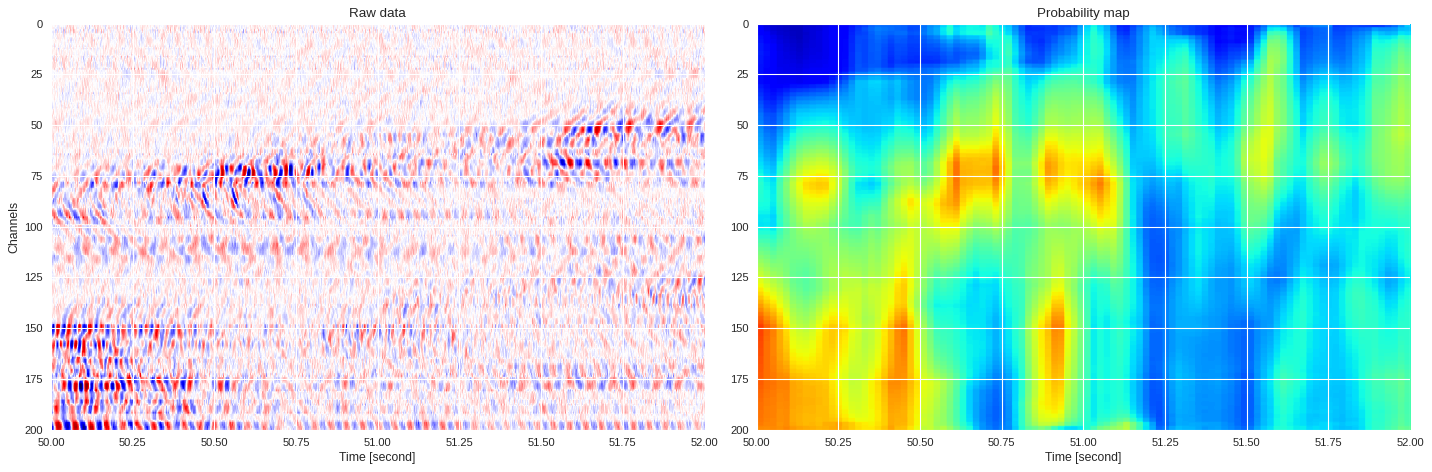
0.043118739128112794 0.8620810013550978
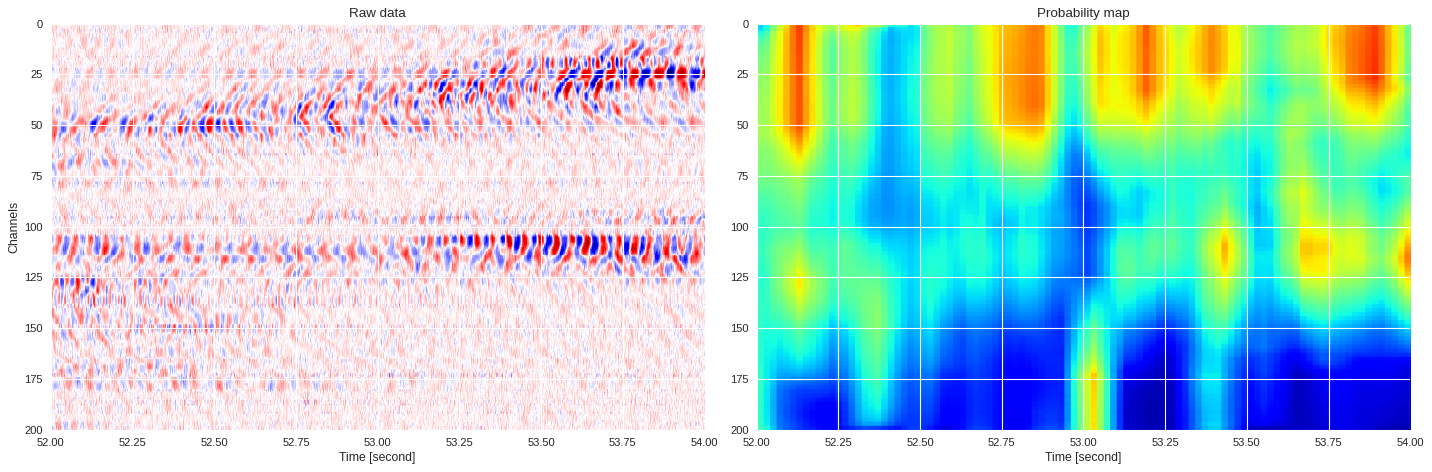
0.04412211179733276 0.8774263100624085
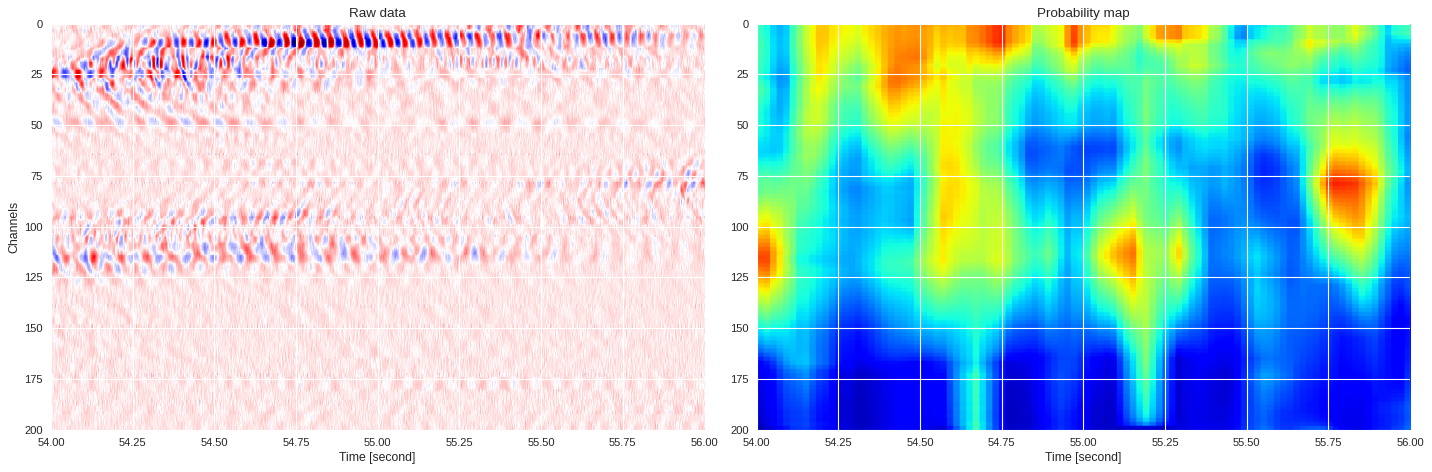
0.06658426523208619 0.8499905009269715
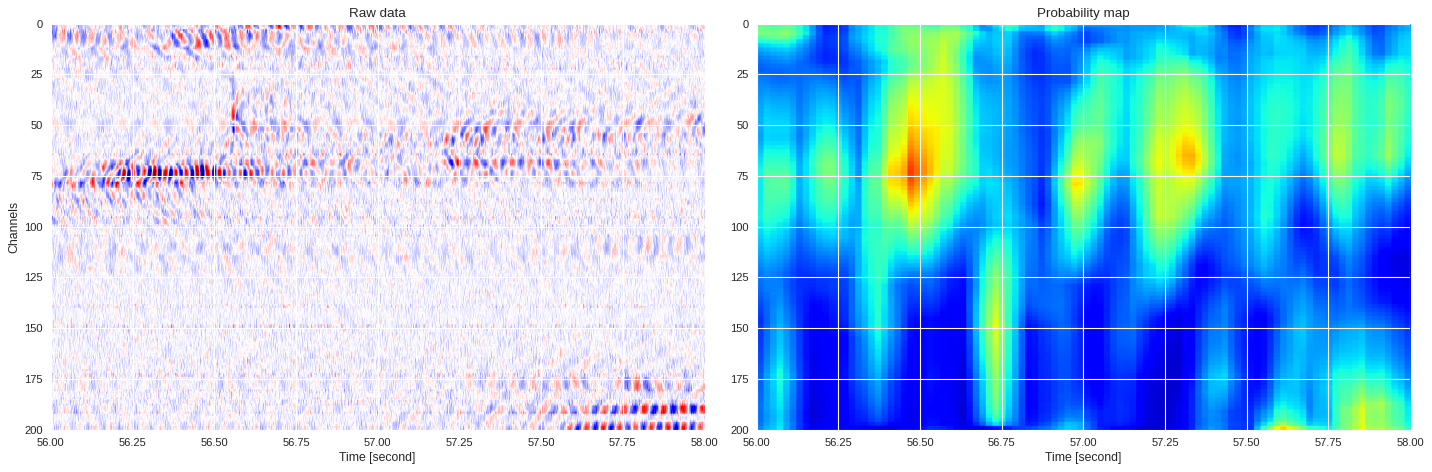
0.07454317951202392 0.8658456007639567
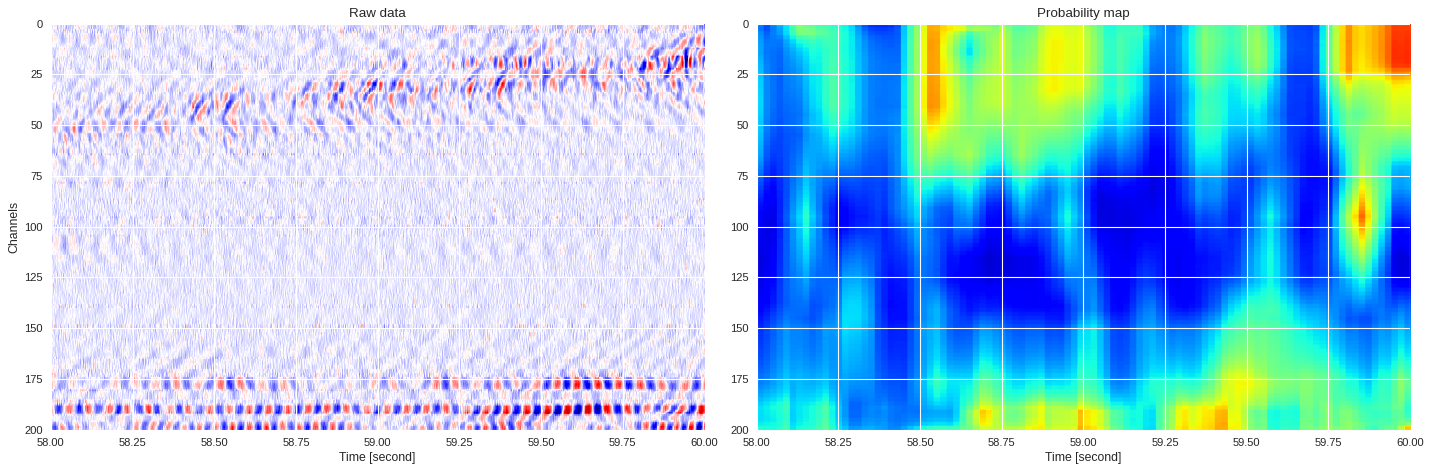
1k 3-class 200x200 dataset ¶
[ ]:
%%capture
!cd /content && tar -zxvf /content/drive/Shared\ drives/ML4DAS/Analysis/Vincent_Dumont/Systematic\ Search/datasets_1k.tar.gz
[ ]:
import torch
from torchvision import transforms, datasets
mytransforms = transforms.Compose([transforms.ToTensor()])
# Load training and testing binary class datasets
trainset = datasets.ImageFolder(root='/content/train',transform=mytransforms)
testset = datasets.ImageFolder(root='/content/test',transform=mytransforms)
# Create data loader for binary class datasets
train_loader = torch.utils.data.DataLoader(trainset,batch_size=100,shuffle=True)
test_loader = torch.utils.data.DataLoader(testset,batch_size=100,shuffle=True)
[ ]:
import h5py,numpy
fname = '/content/drive/Shared drives/ML4DAS/RawData/30min_files_NoTrain/Dsi_30min_170804230056_170804233056_ch5500_6000.mat'
sample_min,sample_max = 330900,331800
channel_min,channel_max = 200,500
f = h5py.File(fname,'r')
data = numpy.array(f[f.get('dsi30/dat')[0,0]][channel_min:channel_max,sample_min:sample_max])
f.close()
Training 1: 8-layer 1-epoch model with 0.001 learning rate ¶
[ ]:
import mldas
model = mldas.ResNet(depth=20,num_classes=3)
criterion = torch.nn.CrossEntropyLoss()
optimizer = torch.optim.SGD(model.parameters(), lr=0.01, momentum=0.9)
loss_hist,models = mldas.suplearn_simple(model,criterion,optimizer,train_loader,test_loader,epochs=10,save_model=True,verbose=True)
Epoch 1/10 | Batch 1/21 | Training loss: 1.05001 | Training accuracy: 30.000 (30/100) | Validation loss: 1.61173 | Validation accuracy: 33.333 (150/450)
Epoch 1/10 | Batch 2/21 | Training loss: 1.03352 | Training accuracy: 53.000 (53/100) | Validation loss: 1.23469 | Validation accuracy: 33.333 (150/450)
Epoch 1/10 | Batch 3/21 | Training loss: 0.97651 | Training accuracy: 52.000 (52/100) | Validation loss: 1.08888 | Validation accuracy: 39.333 (177/450)
Epoch 1/10 | Batch 4/21 | Training loss: 0.91347 | Training accuracy: 50.000 (50/100) | Validation loss: 1.07366 | Validation accuracy: 41.333 (186/450)
Epoch 1/10 | Batch 5/21 | Training loss: 0.80858 | Training accuracy: 62.000 (62/100) | Validation loss: 1.09188 | Validation accuracy: 33.111 (149/450)
Epoch 1/10 | Batch 6/21 | Training loss: 0.70297 | Training accuracy: 74.000 (74/100) | Validation loss: 1.10022 | Validation accuracy: 31.556 (142/450)
Epoch 1/10 | Batch 7/21 | Training loss: 0.65474 | Training accuracy: 77.000 (77/100) | Validation loss: 1.12089 | Validation accuracy: 33.556 (151/450)
Epoch 1/10 | Batch 8/21 | Training loss: 0.55284 | Training accuracy: 83.000 (83/100) | Validation loss: 1.14805 | Validation accuracy: 33.333 (150/450)
Epoch 1/10 | Batch 9/21 | Training loss: 0.52808 | Training accuracy: 88.000 (88/100) | Validation loss: 1.18252 | Validation accuracy: 33.333 (150/450)
Epoch 1/10 | Batch 10/21 | Training loss: 0.51628 | Training accuracy: 83.000 (83/100) | Validation loss: 1.24108 | Validation accuracy: 33.333 (150/450)
Epoch 1/10 | Batch 11/21 | Training loss: 0.46833 | Training accuracy: 83.000 (83/100) | Validation loss: 1.33064 | Validation accuracy: 33.333 (150/450)
Epoch 1/10 | Batch 12/21 | Training loss: 0.42849 | Training accuracy: 88.000 (88/100) | Validation loss: 1.46300 | Validation accuracy: 33.333 (150/450)
Epoch 1/10 | Batch 13/21 | Training loss: 0.41158 | Training accuracy: 88.000 (88/100) | Validation loss: 1.63293 | Validation accuracy: 33.333 (150/450)
Epoch 1/10 | Batch 14/21 | Training loss: 0.38474 | Training accuracy: 89.000 (89/100) | Validation loss: 1.84069 | Validation accuracy: 33.333 (150/450)
Epoch 1/10 | Batch 15/21 | Training loss: 0.35794 | Training accuracy: 90.000 (90/100) | Validation loss: 2.06101 | Validation accuracy: 33.333 (150/450)
Epoch 1/10 | Batch 16/21 | Training loss: 0.34900 | Training accuracy: 86.000 (86/100) | Validation loss: 2.27876 | Validation accuracy: 33.333 (150/450)
Epoch 1/10 | Batch 17/21 | Training loss: 0.25899 | Training accuracy: 93.000 (93/100) | Validation loss: 2.50759 | Validation accuracy: 33.333 (150/450)
Epoch 1/10 | Batch 18/21 | Training loss: 0.23350 | Training accuracy: 95.000 (95/100) | Validation loss: 2.73723 | Validation accuracy: 33.333 (150/450)
Epoch 1/10 | Batch 19/21 | Training loss: 0.30376 | Training accuracy: 88.000 (88/100) | Validation loss: 2.95870 | Validation accuracy: 33.333 (150/450)
Epoch 1/10 | Batch 20/21 | Training loss: 0.30748 | Training accuracy: 87.000 (87/100) | Validation loss: 3.17311 | Validation accuracy: 33.333 (150/450)
Epoch 1/10 | Batch 21/21 | Training loss: 0.25557 | Training accuracy: 93.000 (93/100) | Validation loss: 3.35334 | Validation accuracy: 33.333 (150/450)
Epoch 2/10 | Batch 1/21 | Training loss: 0.20319 | Training accuracy: 91.000 (91/100) | Validation loss: 3.51425 | Validation accuracy: 33.333 (150/450)
Epoch 2/10 | Batch 2/21 | Training loss: 0.16605 | Training accuracy: 96.000 (96/100) | Validation loss: 3.65485 | Validation accuracy: 33.333 (150/450)
Epoch 2/10 | Batch 3/21 | Training loss: 0.18031 | Training accuracy: 91.000 (91/100) | Validation loss: 3.75194 | Validation accuracy: 33.333 (150/450)
Epoch 2/10 | Batch 4/21 | Training loss: 0.25370 | Training accuracy: 88.000 (88/100) | Validation loss: 3.81447 | Validation accuracy: 33.333 (150/450)
Epoch 2/10 | Batch 5/21 | Training loss: 0.27190 | Training accuracy: 87.000 (87/100) | Validation loss: 3.86790 | Validation accuracy: 33.333 (150/450)
Epoch 2/10 | Batch 6/21 | Training loss: 0.18229 | Training accuracy: 90.000 (90/100) | Validation loss: 3.91472 | Validation accuracy: 33.333 (150/450)
Epoch 2/10 | Batch 7/21 | Training loss: 0.13739 | Training accuracy: 95.000 (95/100) | Validation loss: 3.96719 | Validation accuracy: 33.333 (150/450)
Epoch 2/10 | Batch 8/21 | Training loss: 0.13596 | Training accuracy: 96.000 (96/100) | Validation loss: 4.01576 | Validation accuracy: 33.333 (150/450)
Epoch 2/10 | Batch 9/21 | Training loss: 0.18157 | Training accuracy: 92.000 (92/100) | Validation loss: 4.09256 | Validation accuracy: 33.333 (150/450)
Epoch 2/10 | Batch 10/21 | Training loss: 0.24465 | Training accuracy: 91.000 (91/100) | Validation loss: 4.17148 | Validation accuracy: 33.333 (150/450)
Epoch 2/10 | Batch 11/21 | Training loss: 0.15584 | Training accuracy: 94.000 (94/100) | Validation loss: 4.21666 | Validation accuracy: 33.333 (150/450)
Epoch 2/10 | Batch 12/21 | Training loss: 0.13139 | Training accuracy: 94.000 (94/100) | Validation loss: 4.28920 | Validation accuracy: 33.333 (150/450)
Epoch 2/10 | Batch 13/21 | Training loss: 0.10100 | Training accuracy: 96.000 (96/100) | Validation loss: 4.33249 | Validation accuracy: 33.333 (150/450)
Epoch 2/10 | Batch 14/21 | Training loss: 0.11494 | Training accuracy: 93.000 (93/100) | Validation loss: 4.34221 | Validation accuracy: 33.333 (150/450)
Epoch 2/10 | Batch 15/21 | Training loss: 0.20240 | Training accuracy: 91.000 (91/100) | Validation loss: 4.36490 | Validation accuracy: 33.333 (150/450)
Epoch 2/10 | Batch 16/21 | Training loss: 0.10862 | Training accuracy: 96.000 (96/100) | Validation loss: 4.34339 | Validation accuracy: 33.333 (150/450)
Epoch 2/10 | Batch 17/21 | Training loss: 0.17097 | Training accuracy: 94.000 (94/100) | Validation loss: 4.31367 | Validation accuracy: 33.333 (150/450)
Epoch 2/10 | Batch 18/21 | Training loss: 0.11907 | Training accuracy: 96.000 (96/100) | Validation loss: 4.31241 | Validation accuracy: 33.333 (150/450)
Epoch 2/10 | Batch 19/21 | Training loss: 0.17461 | Training accuracy: 97.000 (97/100) | Validation loss: 4.31470 | Validation accuracy: 33.333 (150/450)
Epoch 2/10 | Batch 20/21 | Training loss: 0.11936 | Training accuracy: 95.000 (95/100) | Validation loss: 4.36355 | Validation accuracy: 33.333 (150/450)
Epoch 2/10 | Batch 21/21 | Training loss: 0.16522 | Training accuracy: 93.000 (93/100) | Validation loss: 4.44572 | Validation accuracy: 33.333 (150/450)
Epoch 3/10 | Batch 1/21 | Training loss: 0.15369 | Training accuracy: 96.000 (96/100) | Validation loss: 4.42846 | Validation accuracy: 33.333 (150/450)
Epoch 3/10 | Batch 2/21 | Training loss: 0.07278 | Training accuracy: 98.000 (98/100) | Validation loss: 4.35592 | Validation accuracy: 33.333 (150/450)
Epoch 3/10 | Batch 3/21 | Training loss: 0.13711 | Training accuracy: 94.000 (94/100) | Validation loss: 4.26998 | Validation accuracy: 33.333 (150/450)
Epoch 3/10 | Batch 4/21 | Training loss: 0.11818 | Training accuracy: 94.000 (94/100) | Validation loss: 4.11398 | Validation accuracy: 33.333 (150/450)
Epoch 3/10 | Batch 5/21 | Training loss: 0.20672 | Training accuracy: 91.000 (91/100) | Validation loss: 3.94644 | Validation accuracy: 33.333 (150/450)
Epoch 3/10 | Batch 6/21 | Training loss: 0.19231 | Training accuracy: 91.000 (91/100) | Validation loss: 3.71843 | Validation accuracy: 33.333 (150/450)
Epoch 3/10 | Batch 7/21 | Training loss: 0.24001 | Training accuracy: 94.000 (94/100) | Validation loss: 3.26050 | Validation accuracy: 34.000 (153/450)
Epoch 3/10 | Batch 8/21 | Training loss: 0.20110 | Training accuracy: 92.000 (92/100) | Validation loss: 2.47815 | Validation accuracy: 38.000 (171/450)
Epoch 3/10 | Batch 9/21 | Training loss: 0.15552 | Training accuracy: 95.000 (95/100) | Validation loss: 1.46767 | Validation accuracy: 50.667 (228/450)
Epoch 3/10 | Batch 10/21 | Training loss: 0.24906 | Training accuracy: 91.000 (91/100) | Validation loss: 0.58271 | Validation accuracy: 67.111 (302/450)
Epoch 3/10 | Batch 11/21 | Training loss: 0.13053 | Training accuracy: 95.000 (95/100) | Validation loss: 0.51822 | Validation accuracy: 78.444 (353/450)
Epoch 3/10 | Batch 12/21 | Training loss: 0.07812 | Training accuracy: 99.000 (99/100) | Validation loss: 1.01285 | Validation accuracy: 68.444 (308/450)
Epoch 3/10 | Batch 13/21 | Training loss: 0.08234 | Training accuracy: 98.000 (98/100) | Validation loss: 1.34620 | Validation accuracy: 66.889 (301/450)
Epoch 3/10 | Batch 14/21 | Training loss: 0.16661 | Training accuracy: 92.000 (92/100) | Validation loss: 1.59905 | Validation accuracy: 64.667 (291/450)
Epoch 3/10 | Batch 15/21 | Training loss: 0.14305 | Training accuracy: 96.000 (96/100) | Validation loss: 1.48601 | Validation accuracy: 65.333 (294/450)
Epoch 3/10 | Batch 16/21 | Training loss: 0.07268 | Training accuracy: 99.000 (99/100) | Validation loss: 1.25349 | Validation accuracy: 67.111 (302/450)
Epoch 3/10 | Batch 17/21 | Training loss: 0.15590 | Training accuracy: 95.000 (95/100) | Validation loss: 0.65387 | Validation accuracy: 74.889 (337/450)
Epoch 3/10 | Batch 18/21 | Training loss: 0.16214 | Training accuracy: 96.000 (96/100) | Validation loss: 0.31226 | Validation accuracy: 84.222 (379/450)
Epoch 3/10 | Batch 19/21 | Training loss: 0.05471 | Training accuracy: 99.000 (99/100) | Validation loss: 0.69909 | Validation accuracy: 63.778 (287/450)
Epoch 3/10 | Batch 20/21 | Training loss: 0.10219 | Training accuracy: 97.000 (97/100) | Validation loss: 1.01013 | Validation accuracy: 51.556 (232/450)
Epoch 3/10 | Batch 21/21 | Training loss: 0.13359 | Training accuracy: 93.000 (93/100) | Validation loss: 1.17996 | Validation accuracy: 47.111 (212/450)
Epoch 4/10 | Batch 1/21 | Training loss: 0.08643 | Training accuracy: 96.000 (96/100) | Validation loss: 1.19409 | Validation accuracy: 45.333 (204/450)
Epoch 4/10 | Batch 2/21 | Training loss: 0.04830 | Training accuracy: 100.000 (100/100) | Validation loss: 1.06330 | Validation accuracy: 47.556 (214/450)
Epoch 4/10 | Batch 3/21 | Training loss: 0.11741 | Training accuracy: 95.000 (95/100) | Validation loss: 0.83663 | Validation accuracy: 53.556 (241/450)
Epoch 4/10 | Batch 4/21 | Training loss: 0.10442 | Training accuracy: 96.000 (96/100) | Validation loss: 0.67213 | Validation accuracy: 61.111 (275/450)
Epoch 4/10 | Batch 5/21 | Training loss: 0.14338 | Training accuracy: 94.000 (94/100) | Validation loss: 0.54783 | Validation accuracy: 70.222 (316/450)
Epoch 4/10 | Batch 6/21 | Training loss: 0.10876 | Training accuracy: 97.000 (97/100) | Validation loss: 0.47509 | Validation accuracy: 75.111 (338/450)
Epoch 4/10 | Batch 7/21 | Training loss: 0.05962 | Training accuracy: 99.000 (99/100) | Validation loss: 0.39484 | Validation accuracy: 78.889 (355/450)
Epoch 4/10 | Batch 8/21 | Training loss: 0.09337 | Training accuracy: 96.000 (96/100) | Validation loss: 0.30458 | Validation accuracy: 84.667 (381/450)
Epoch 4/10 | Batch 9/21 | Training loss: 0.11662 | Training accuracy: 95.000 (95/100) | Validation loss: 0.25358 | Validation accuracy: 88.222 (397/450)
Epoch 4/10 | Batch 10/21 | Training loss: 0.10531 | Training accuracy: 96.000 (96/100) | Validation loss: 0.20495 | Validation accuracy: 92.000 (414/450)
Epoch 4/10 | Batch 11/21 | Training loss: 0.12376 | Training accuracy: 95.000 (95/100) | Validation loss: 0.18149 | Validation accuracy: 94.000 (423/450)
Epoch 4/10 | Batch 12/21 | Training loss: 0.07194 | Training accuracy: 98.000 (98/100) | Validation loss: 0.21964 | Validation accuracy: 90.444 (407/450)
Epoch 4/10 | Batch 13/21 | Training loss: 0.14678 | Training accuracy: 94.000 (94/100) | Validation loss: 0.35355 | Validation accuracy: 85.556 (385/450)
Epoch 4/10 | Batch 14/21 | Training loss: 0.07522 | Training accuracy: 99.000 (99/100) | Validation loss: 0.59637 | Validation accuracy: 78.889 (355/450)
Epoch 4/10 | Batch 15/21 | Training loss: 0.10714 | Training accuracy: 95.000 (95/100) | Validation loss: 0.87329 | Validation accuracy: 72.444 (326/450)
Epoch 4/10 | Batch 16/21 | Training loss: 0.11087 | Training accuracy: 96.000 (96/100) | Validation loss: 0.96561 | Validation accuracy: 71.556 (322/450)
Epoch 4/10 | Batch 17/21 | Training loss: 0.14141 | Training accuracy: 97.000 (97/100) | Validation loss: 0.83999 | Validation accuracy: 74.222 (334/450)
Epoch 4/10 | Batch 18/21 | Training loss: 0.09985 | Training accuracy: 95.000 (95/100) | Validation loss: 0.52818 | Validation accuracy: 79.556 (358/450)
Epoch 4/10 | Batch 19/21 | Training loss: 0.04077 | Training accuracy: 100.000 (100/100) | Validation loss: 0.34704 | Validation accuracy: 85.556 (385/450)
Epoch 4/10 | Batch 20/21 | Training loss: 0.05299 | Training accuracy: 100.000 (100/100) | Validation loss: 0.19611 | Validation accuracy: 90.222 (406/450)
Epoch 4/10 | Batch 21/21 | Training loss: 0.07755 | Training accuracy: 99.000 (99/100) | Validation loss: 0.12119 | Validation accuracy: 94.444 (425/450)
Epoch 5/10 | Batch 1/21 | Training loss: 0.10633 | Training accuracy: 96.000 (96/100) | Validation loss: 0.09157 | Validation accuracy: 95.778 (431/450)
Epoch 5/10 | Batch 2/21 | Training loss: 0.05910 | Training accuracy: 98.000 (98/100) | Validation loss: 0.09779 | Validation accuracy: 95.556 (430/450)
Epoch 5/10 | Batch 3/21 | Training loss: 0.07986 | Training accuracy: 97.000 (97/100) | Validation loss: 0.12310 | Validation accuracy: 95.333 (429/450)
Epoch 5/10 | Batch 4/21 | Training loss: 0.14491 | Training accuracy: 93.000 (93/100) | Validation loss: 0.09880 | Validation accuracy: 95.778 (431/450)
Epoch 5/10 | Batch 5/21 | Training loss: 0.04238 | Training accuracy: 99.000 (99/100) | Validation loss: 0.09188 | Validation accuracy: 96.444 (434/450)
Epoch 5/10 | Batch 6/21 | Training loss: 0.06924 | Training accuracy: 98.000 (98/100) | Validation loss: 0.08735 | Validation accuracy: 96.444 (434/450)
Epoch 5/10 | Batch 7/21 | Training loss: 0.04551 | Training accuracy: 100.000 (100/100) | Validation loss: 0.08939 | Validation accuracy: 96.444 (434/450)
Epoch 5/10 | Batch 8/21 | Training loss: 0.04379 | Training accuracy: 100.000 (100/100) | Validation loss: 0.09427 | Validation accuracy: 96.222 (433/450)
Epoch 5/10 | Batch 9/21 | Training loss: 0.08050 | Training accuracy: 97.000 (97/100) | Validation loss: 0.09349 | Validation accuracy: 96.222 (433/450)
Epoch 5/10 | Batch 10/21 | Training loss: 0.10386 | Training accuracy: 96.000 (96/100) | Validation loss: 0.08848 | Validation accuracy: 96.889 (436/450)
Epoch 5/10 | Batch 11/21 | Training loss: 0.11053 | Training accuracy: 96.000 (96/100) | Validation loss: 0.10636 | Validation accuracy: 97.111 (437/450)
Epoch 5/10 | Batch 12/21 | Training loss: 0.02319 | Training accuracy: 100.000 (100/100) | Validation loss: 0.13379 | Validation accuracy: 94.444 (425/450)
Epoch 5/10 | Batch 13/21 | Training loss: 0.05299 | Training accuracy: 99.000 (99/100) | Validation loss: 0.15782 | Validation accuracy: 94.222 (424/450)
Epoch 5/10 | Batch 14/21 | Training loss: 0.08531 | Training accuracy: 97.000 (97/100) | Validation loss: 0.17053 | Validation accuracy: 94.000 (423/450)
Epoch 5/10 | Batch 15/21 | Training loss: 0.07115 | Training accuracy: 98.000 (98/100) | Validation loss: 0.16166 | Validation accuracy: 94.222 (424/450)
Epoch 5/10 | Batch 16/21 | Training loss: 0.08635 | Training accuracy: 98.000 (98/100) | Validation loss: 0.14483 | Validation accuracy: 95.778 (431/450)
Epoch 5/10 | Batch 17/21 | Training loss: 0.06850 | Training accuracy: 97.000 (97/100) | Validation loss: 0.12527 | Validation accuracy: 96.444 (434/450)
Epoch 5/10 | Batch 18/21 | Training loss: 0.07694 | Training accuracy: 99.000 (99/100) | Validation loss: 0.10721 | Validation accuracy: 97.111 (437/450)
Epoch 5/10 | Batch 19/21 | Training loss: 0.07287 | Training accuracy: 99.000 (99/100) | Validation loss: 0.09626 | Validation accuracy: 97.333 (438/450)
Epoch 5/10 | Batch 20/21 | Training loss: 0.04225 | Training accuracy: 99.000 (99/100) | Validation loss: 0.10224 | Validation accuracy: 96.667 (435/450)
Epoch 5/10 | Batch 21/21 | Training loss: 0.03785 | Training accuracy: 99.000 (99/100) | Validation loss: 0.13491 | Validation accuracy: 94.667 (426/450)
Epoch 6/10 | Batch 1/21 | Training loss: 0.05290 | Training accuracy: 99.000 (99/100) | Validation loss: 0.21687 | Validation accuracy: 90.444 (407/450)
Epoch 6/10 | Batch 2/21 | Training loss: 0.06076 | Training accuracy: 98.000 (98/100) | Validation loss: 0.23123 | Validation accuracy: 90.667 (408/450)
Epoch 6/10 | Batch 3/21 | Training loss: 0.04920 | Training accuracy: 98.000 (98/100) | Validation loss: 0.23845 | Validation accuracy: 90.000 (405/450)
Epoch 6/10 | Batch 4/21 | Training loss: 0.05396 | Training accuracy: 98.000 (98/100) | Validation loss: 0.17794 | Validation accuracy: 93.111 (419/450)
Epoch 6/10 | Batch 5/21 | Training loss: 0.14505 | Training accuracy: 94.000 (94/100) | Validation loss: 0.09842 | Validation accuracy: 95.111 (428/450)
Epoch 6/10 | Batch 6/21 | Training loss: 0.07126 | Training accuracy: 97.000 (97/100) | Validation loss: 0.07740 | Validation accuracy: 96.667 (435/450)
Epoch 6/10 | Batch 7/21 | Training loss: 0.05854 | Training accuracy: 99.000 (99/100) | Validation loss: 0.09718 | Validation accuracy: 95.778 (431/450)
Epoch 6/10 | Batch 8/21 | Training loss: 0.04307 | Training accuracy: 99.000 (99/100) | Validation loss: 0.15362 | Validation accuracy: 93.111 (419/450)
Epoch 6/10 | Batch 9/21 | Training loss: 0.05853 | Training accuracy: 96.000 (96/100) | Validation loss: 0.24340 | Validation accuracy: 89.556 (403/450)
Epoch 6/10 | Batch 10/21 | Training loss: 0.08824 | Training accuracy: 96.000 (96/100) | Validation loss: 0.32941 | Validation accuracy: 86.444 (389/450)
Epoch 6/10 | Batch 11/21 | Training loss: 0.07846 | Training accuracy: 98.000 (98/100) | Validation loss: 0.35219 | Validation accuracy: 86.444 (389/450)
Epoch 6/10 | Batch 12/21 | Training loss: 0.04472 | Training accuracy: 100.000 (100/100) | Validation loss: 0.34994 | Validation accuracy: 86.444 (389/450)
Epoch 6/10 | Batch 13/21 | Training loss: 0.05835 | Training accuracy: 99.000 (99/100) | Validation loss: 0.25559 | Validation accuracy: 89.111 (401/450)
Epoch 6/10 | Batch 14/21 | Training loss: 0.05513 | Training accuracy: 99.000 (99/100) | Validation loss: 0.17645 | Validation accuracy: 91.556 (412/450)
Epoch 6/10 | Batch 15/21 | Training loss: 0.03175 | Training accuracy: 100.000 (100/100) | Validation loss: 0.13478 | Validation accuracy: 95.111 (428/450)
Epoch 6/10 | Batch 16/21 | Training loss: 0.06764 | Training accuracy: 98.000 (98/100) | Validation loss: 0.09718 | Validation accuracy: 96.444 (434/450)
Epoch 6/10 | Batch 17/21 | Training loss: 0.08075 | Training accuracy: 97.000 (97/100) | Validation loss: 0.07449 | Validation accuracy: 97.333 (438/450)
Epoch 6/10 | Batch 18/21 | Training loss: 0.07808 | Training accuracy: 97.000 (97/100) | Validation loss: 0.07476 | Validation accuracy: 98.000 (441/450)
Epoch 6/10 | Batch 19/21 | Training loss: 0.04719 | Training accuracy: 98.000 (98/100) | Validation loss: 0.08524 | Validation accuracy: 97.778 (440/450)
Epoch 6/10 | Batch 20/21 | Training loss: 0.03112 | Training accuracy: 99.000 (99/100) | Validation loss: 0.10769 | Validation accuracy: 97.111 (437/450)
Epoch 6/10 | Batch 21/21 | Training loss: 0.03213 | Training accuracy: 100.000 (100/100) | Validation loss: 0.13814 | Validation accuracy: 96.222 (433/450)
Epoch 7/10 | Batch 1/21 | Training loss: 0.09038 | Training accuracy: 97.000 (97/100) | Validation loss: 0.15323 | Validation accuracy: 95.111 (428/450)
Epoch 7/10 | Batch 2/21 | Training loss: 0.04865 | Training accuracy: 98.000 (98/100) | Validation loss: 0.17422 | Validation accuracy: 94.667 (426/450)
Epoch 7/10 | Batch 3/21 | Training loss: 0.02917 | Training accuracy: 100.000 (100/100) | Validation loss: 0.17830 | Validation accuracy: 95.111 (428/450)
Epoch 7/10 | Batch 4/21 | Training loss: 0.05211 | Training accuracy: 98.000 (98/100) | Validation loss: 0.15007 | Validation accuracy: 95.333 (429/450)
Epoch 7/10 | Batch 5/21 | Training loss: 0.01630 | Training accuracy: 100.000 (100/100) | Validation loss: 0.12819 | Validation accuracy: 96.222 (433/450)
Epoch 7/10 | Batch 6/21 | Training loss: 0.03814 | Training accuracy: 100.000 (100/100) | Validation loss: 0.10907 | Validation accuracy: 96.889 (436/450)
Epoch 7/10 | Batch 7/21 | Training loss: 0.02314 | Training accuracy: 100.000 (100/100) | Validation loss: 0.10038 | Validation accuracy: 97.333 (438/450)
Epoch 7/10 | Batch 8/21 | Training loss: 0.06989 | Training accuracy: 97.000 (97/100) | Validation loss: 0.07322 | Validation accuracy: 98.000 (441/450)
Epoch 7/10 | Batch 9/21 | Training loss: 0.01815 | Training accuracy: 100.000 (100/100) | Validation loss: 0.06074 | Validation accuracy: 98.667 (444/450)
Epoch 7/10 | Batch 10/21 | Training loss: 0.06389 | Training accuracy: 97.000 (97/100) | Validation loss: 0.05578 | Validation accuracy: 98.444 (443/450)
Epoch 7/10 | Batch 11/21 | Training loss: 0.04755 | Training accuracy: 98.000 (98/100) | Validation loss: 0.05046 | Validation accuracy: 98.444 (443/450)
Epoch 7/10 | Batch 12/21 | Training loss: 0.02764 | Training accuracy: 100.000 (100/100) | Validation loss: 0.04752 | Validation accuracy: 98.667 (444/450)
Epoch 7/10 | Batch 13/21 | Training loss: 0.06118 | Training accuracy: 98.000 (98/100) | Validation loss: 0.04617 | Validation accuracy: 98.444 (443/450)
Epoch 7/10 | Batch 14/21 | Training loss: 0.03103 | Training accuracy: 100.000 (100/100) | Validation loss: 0.04685 | Validation accuracy: 98.444 (443/450)
Epoch 7/10 | Batch 15/21 | Training loss: 0.02417 | Training accuracy: 99.000 (99/100) | Validation loss: 0.04880 | Validation accuracy: 98.444 (443/450)
Epoch 7/10 | Batch 16/21 | Training loss: 0.09778 | Training accuracy: 96.000 (96/100) | Validation loss: 0.06111 | Validation accuracy: 98.444 (443/450)
Epoch 7/10 | Batch 17/21 | Training loss: 0.02377 | Training accuracy: 100.000 (100/100) | Validation loss: 0.07891 | Validation accuracy: 97.778 (440/450)
Epoch 7/10 | Batch 18/21 | Training loss: 0.10074 | Training accuracy: 96.000 (96/100) | Validation loss: 0.09429 | Validation accuracy: 96.889 (436/450)
Epoch 7/10 | Batch 19/21 | Training loss: 0.03243 | Training accuracy: 100.000 (100/100) | Validation loss: 0.11968 | Validation accuracy: 95.778 (431/450)
Epoch 7/10 | Batch 20/21 | Training loss: 0.02868 | Training accuracy: 99.000 (99/100) | Validation loss: 0.12417 | Validation accuracy: 96.000 (432/450)
Epoch 7/10 | Batch 21/21 | Training loss: 0.04346 | Training accuracy: 98.000 (98/100) | Validation loss: 0.13386 | Validation accuracy: 95.111 (428/450)
Epoch 8/10 | Batch 1/21 | Training loss: 0.02331 | Training accuracy: 100.000 (100/100) | Validation loss: 0.13658 | Validation accuracy: 94.889 (427/450)
Epoch 8/10 | Batch 2/21 | Training loss: 0.05075 | Training accuracy: 99.000 (99/100) | Validation loss: 0.11358 | Validation accuracy: 96.000 (432/450)
Epoch 8/10 | Batch 3/21 | Training loss: 0.04165 | Training accuracy: 99.000 (99/100) | Validation loss: 0.08547 | Validation accuracy: 97.333 (438/450)
Epoch 8/10 | Batch 4/21 | Training loss: 0.05006 | Training accuracy: 100.000 (100/100) | Validation loss: 0.05856 | Validation accuracy: 98.222 (442/450)
Epoch 8/10 | Batch 5/21 | Training loss: 0.03628 | Training accuracy: 99.000 (99/100) | Validation loss: 0.04663 | Validation accuracy: 98.222 (442/450)
Epoch 8/10 | Batch 6/21 | Training loss: 0.04146 | Training accuracy: 99.000 (99/100) | Validation loss: 0.04082 | Validation accuracy: 98.667 (444/450)
Epoch 8/10 | Batch 7/21 | Training loss: 0.01949 | Training accuracy: 100.000 (100/100) | Validation loss: 0.03878 | Validation accuracy: 98.889 (445/450)
Epoch 8/10 | Batch 8/21 | Training loss: 0.04185 | Training accuracy: 98.000 (98/100) | Validation loss: 0.04098 | Validation accuracy: 99.111 (446/450)
Epoch 8/10 | Batch 9/21 | Training loss: 0.02544 | Training accuracy: 99.000 (99/100) | Validation loss: 0.04473 | Validation accuracy: 98.444 (443/450)
Epoch 8/10 | Batch 10/21 | Training loss: 0.04576 | Training accuracy: 99.000 (99/100) | Validation loss: 0.05099 | Validation accuracy: 98.000 (441/450)
Epoch 8/10 | Batch 11/21 | Training loss: 0.05790 | Training accuracy: 98.000 (98/100) | Validation loss: 0.05231 | Validation accuracy: 97.778 (440/450)
Epoch 8/10 | Batch 12/21 | Training loss: 0.04275 | Training accuracy: 99.000 (99/100) | Validation loss: 0.04728 | Validation accuracy: 98.000 (441/450)
Epoch 8/10 | Batch 13/21 | Training loss: 0.03034 | Training accuracy: 100.000 (100/100) | Validation loss: 0.04622 | Validation accuracy: 98.222 (442/450)
Epoch 8/10 | Batch 14/21 | Training loss: 0.03758 | Training accuracy: 100.000 (100/100) | Validation loss: 0.04642 | Validation accuracy: 98.667 (444/450)
Epoch 8/10 | Batch 15/21 | Training loss: 0.02943 | Training accuracy: 100.000 (100/100) | Validation loss: 0.04798 | Validation accuracy: 98.667 (444/450)
Epoch 8/10 | Batch 16/21 | Training loss: 0.02830 | Training accuracy: 100.000 (100/100) | Validation loss: 0.05570 | Validation accuracy: 98.889 (445/450)
Epoch 8/10 | Batch 17/21 | Training loss: 0.03170 | Training accuracy: 99.000 (99/100) | Validation loss: 0.05793 | Validation accuracy: 98.889 (445/450)
Epoch 8/10 | Batch 18/21 | Training loss: 0.04270 | Training accuracy: 98.000 (98/100) | Validation loss: 0.06020 | Validation accuracy: 98.667 (444/450)
Epoch 8/10 | Batch 19/21 | Training loss: 0.04128 | Training accuracy: 99.000 (99/100) | Validation loss: 0.07046 | Validation accuracy: 98.222 (442/450)
Epoch 8/10 | Batch 20/21 | Training loss: 0.01787 | Training accuracy: 100.000 (100/100) | Validation loss: 0.08150 | Validation accuracy: 98.444 (443/450)
Epoch 8/10 | Batch 21/21 | Training loss: 0.03848 | Training accuracy: 98.000 (98/100) | Validation loss: 0.07562 | Validation accuracy: 98.222 (442/450)
Epoch 9/10 | Batch 1/21 | Training loss: 0.03319 | Training accuracy: 99.000 (99/100) | Validation loss: 0.07593 | Validation accuracy: 97.556 (439/450)
Epoch 9/10 | Batch 2/21 | Training loss: 0.03476 | Training accuracy: 99.000 (99/100) | Validation loss: 0.07758 | Validation accuracy: 97.556 (439/450)
Epoch 9/10 | Batch 3/21 | Training loss: 0.03588 | Training accuracy: 99.000 (99/100) | Validation loss: 0.07127 | Validation accuracy: 97.333 (438/450)
Epoch 9/10 | Batch 4/21 | Training loss: 0.03245 | Training accuracy: 98.000 (98/100) | Validation loss: 0.06334 | Validation accuracy: 97.778 (440/450)
Epoch 9/10 | Batch 5/21 | Training loss: 0.03510 | Training accuracy: 98.000 (98/100) | Validation loss: 0.06755 | Validation accuracy: 97.778 (440/450)
Epoch 9/10 | Batch 6/21 | Training loss: 0.01814 | Training accuracy: 100.000 (100/100) | Validation loss: 0.07027 | Validation accuracy: 97.556 (439/450)
Epoch 9/10 | Batch 7/21 | Training loss: 0.02037 | Training accuracy: 99.000 (99/100) | Validation loss: 0.06983 | Validation accuracy: 97.778 (440/450)
Epoch 9/10 | Batch 8/21 | Training loss: 0.00945 | Training accuracy: 100.000 (100/100) | Validation loss: 0.07015 | Validation accuracy: 97.778 (440/450)
Epoch 9/10 | Batch 9/21 | Training loss: 0.02191 | Training accuracy: 100.000 (100/100) | Validation loss: 0.06437 | Validation accuracy: 97.778 (440/450)
Epoch 9/10 | Batch 10/21 | Training loss: 0.01997 | Training accuracy: 99.000 (99/100) | Validation loss: 0.06272 | Validation accuracy: 98.222 (442/450)
Epoch 9/10 | Batch 11/21 | Training loss: 0.03322 | Training accuracy: 99.000 (99/100) | Validation loss: 0.06209 | Validation accuracy: 98.000 (441/450)
Epoch 9/10 | Batch 12/21 | Training loss: 0.01531 | Training accuracy: 100.000 (100/100) | Validation loss: 0.06439 | Validation accuracy: 97.778 (440/450)
Epoch 9/10 | Batch 13/21 | Training loss: 0.02848 | Training accuracy: 100.000 (100/100) | Validation loss: 0.05970 | Validation accuracy: 98.000 (441/450)
Epoch 9/10 | Batch 14/21 | Training loss: 0.01864 | Training accuracy: 100.000 (100/100) | Validation loss: 0.05753 | Validation accuracy: 98.222 (442/450)
Epoch 9/10 | Batch 15/21 | Training loss: 0.04902 | Training accuracy: 98.000 (98/100) | Validation loss: 0.05225 | Validation accuracy: 98.444 (443/450)
Epoch 9/10 | Batch 16/21 | Training loss: 0.04097 | Training accuracy: 98.000 (98/100) | Validation loss: 0.05491 | Validation accuracy: 98.444 (443/450)
Epoch 9/10 | Batch 17/21 | Training loss: 0.04109 | Training accuracy: 98.000 (98/100) | Validation loss: 0.04434 | Validation accuracy: 98.222 (442/450)
Epoch 9/10 | Batch 18/21 | Training loss: 0.01771 | Training accuracy: 100.000 (100/100) | Validation loss: 0.03892 | Validation accuracy: 98.444 (443/450)
Epoch 9/10 | Batch 19/21 | Training loss: 0.01994 | Training accuracy: 99.000 (99/100) | Validation loss: 0.04335 | Validation accuracy: 99.111 (446/450)
Epoch 9/10 | Batch 20/21 | Training loss: 0.04036 | Training accuracy: 98.000 (98/100) | Validation loss: 0.07136 | Validation accuracy: 97.778 (440/450)
Epoch 9/10 | Batch 21/21 | Training loss: 0.04511 | Training accuracy: 97.000 (97/100) | Validation loss: 0.15905 | Validation accuracy: 95.111 (428/450)
Epoch 10/10 | Batch 1/21 | Training loss: 0.02407 | Training accuracy: 100.000 (100/100) | Validation loss: 0.27306 | Validation accuracy: 91.778 (413/450)
Epoch 10/10 | Batch 2/21 | Training loss: 0.03877 | Training accuracy: 98.000 (98/100) | Validation loss: 0.40449 | Validation accuracy: 86.889 (391/450)
Epoch 10/10 | Batch 3/21 | Training loss: 0.01332 | Training accuracy: 100.000 (100/100) | Validation loss: 0.45434 | Validation accuracy: 86.667 (390/450)
Epoch 10/10 | Batch 4/21 | Training loss: 0.03847 | Training accuracy: 98.000 (98/100) | Validation loss: 0.42221 | Validation accuracy: 88.000 (396/450)
Epoch 10/10 | Batch 5/21 | Training loss: 0.02881 | Training accuracy: 100.000 (100/100) | Validation loss: 0.37317 | Validation accuracy: 88.667 (399/450)
Epoch 10/10 | Batch 6/21 | Training loss: 0.05366 | Training accuracy: 98.000 (98/100) | Validation loss: 0.28053 | Validation accuracy: 91.556 (412/450)
Epoch 10/10 | Batch 7/21 | Training loss: 0.03761 | Training accuracy: 99.000 (99/100) | Validation loss: 0.14130 | Validation accuracy: 96.000 (432/450)
Epoch 10/10 | Batch 8/21 | Training loss: 0.02558 | Training accuracy: 100.000 (100/100) | Validation loss: 0.07446 | Validation accuracy: 98.000 (441/450)
Epoch 10/10 | Batch 9/21 | Training loss: 0.07650 | Training accuracy: 97.000 (97/100) | Validation loss: 0.03196 | Validation accuracy: 98.889 (445/450)
Epoch 10/10 | Batch 10/21 | Training loss: 0.08384 | Training accuracy: 95.000 (95/100) | Validation loss: 0.08477 | Validation accuracy: 96.444 (434/450)
Epoch 10/10 | Batch 11/21 | Training loss: 0.01402 | Training accuracy: 100.000 (100/100) | Validation loss: 0.25974 | Validation accuracy: 89.333 (402/450)
Epoch 10/10 | Batch 12/21 | Training loss: 0.01082 | Training accuracy: 100.000 (100/100) | Validation loss: 0.45990 | Validation accuracy: 86.444 (389/450)
Epoch 10/10 | Batch 13/21 | Training loss: 0.03264 | Training accuracy: 100.000 (100/100) | Validation loss: 0.54508 | Validation accuracy: 84.889 (382/450)
Epoch 10/10 | Batch 14/21 | Training loss: 0.02575 | Training accuracy: 99.000 (99/100) | Validation loss: 0.53777 | Validation accuracy: 84.889 (382/450)
Epoch 10/10 | Batch 15/21 | Training loss: 0.01770 | Training accuracy: 100.000 (100/100) | Validation loss: 0.48025 | Validation accuracy: 86.000 (387/450)
Epoch 10/10 | Batch 16/21 | Training loss: 0.03500 | Training accuracy: 98.000 (98/100) | Validation loss: 0.34861 | Validation accuracy: 87.778 (395/450)
Epoch 10/10 | Batch 17/21 | Training loss: 0.02895 | Training accuracy: 100.000 (100/100) | Validation loss: 0.18074 | Validation accuracy: 92.444 (416/450)
Epoch 10/10 | Batch 18/21 | Training loss: 0.04354 | Training accuracy: 98.000 (98/100) | Validation loss: 0.06676 | Validation accuracy: 97.333 (438/450)
Epoch 10/10 | Batch 19/21 | Training loss: 0.02365 | Training accuracy: 99.000 (99/100) | Validation loss: 0.04519 | Validation accuracy: 98.444 (443/450)
Epoch 10/10 | Batch 20/21 | Training loss: 0.01875 | Training accuracy: 99.000 (99/100) | Validation loss: 0.06532 | Validation accuracy: 97.556 (439/450)
Epoch 10/10 | Batch 21/21 | Training loss: 0.04241 | Training accuracy: 99.000 (99/100) | Validation loss: 0.09753 | Validation accuracy: 96.222 (433/450)
[ ]:
import matplotlib.pyplot as plt
plt.style.use('seaborn')
fig,ax = plt.subplots(1,2,figsize=(18,5),dpi=80,sharex=True)
ax[0].plot(loss_hist[:,3]/loss_hist[:,2],label='Training loss')
ax[0].plot(loss_hist[:,6]/loss_hist[:,5],label='Validation loss')
ax[0].set_xlabel('Batch Iterations')
ax[0].set_ylabel('Loss')
ax[0].legend(frameon=False)
ax[1].plot(100*loss_hist[:,4]/loss_hist[:,2],label='Training accuracy')
ax[1].plot(100*loss_hist[:,7]/loss_hist[:,5],label='Validation accuracy')
ax[1].set_xlabel('Batch Iterations')
ax[1].set_ylabel('Accuracy')
ax[1].legend(frameon=False)
plt.tight_layout()
plt.show()
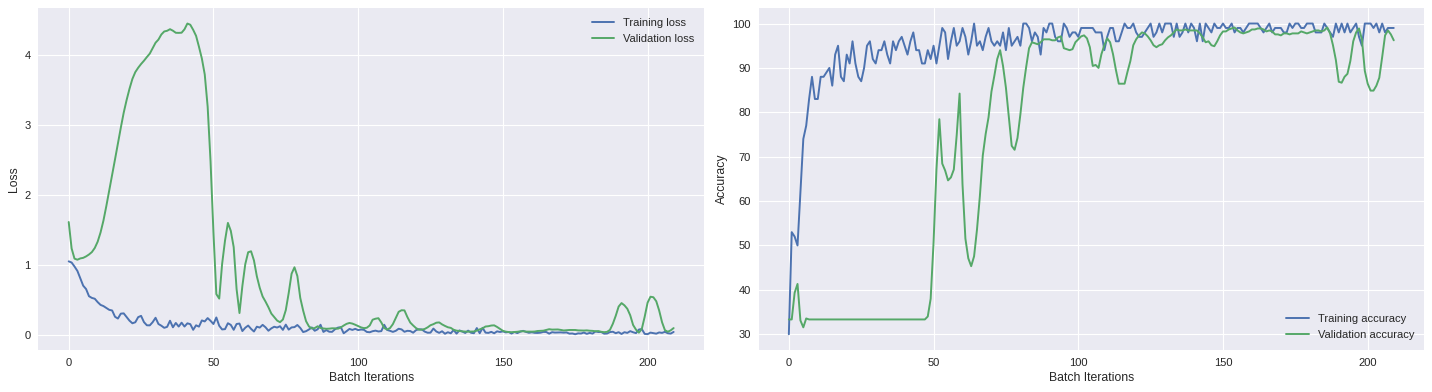
[ ]:
import os
os.system('mkdir -p training5/models/')
numpy.save('training5/loss_hist',loss_hist)
for key in models.keys():
torch.save(models[key].state_dict(),'training5/models/model%03i.pt'%key)
No train DAS file ¶
[ ]:
import h5py
fname = '/content/drive/Shared drives/ML4DAS/RawData/30min_files_NoTrain/Dsi_30min_170804230056_170804233056_ch5500_6000.mat'
f = h5py.File(fname,'r')
data = numpy.array(f[f.get('dsi30/dat')[0,0]][100:500,330000:333000])
f.close()
[ ]:
import h5py,numpy,torch
from PIL import Image
from matplotlib import cm
import matplotlib.pyplot as plt
from torchvision import transforms
def prob_map(data,model,img_size=200,channel_stride=200,sample_stride=200,binary=False,inverse=False):
# Calculate probability map
model.eval() # Set model to evalutation mode
prob_array = numpy.zeros((2,*data.shape)) # Initialize probability map
idxs = numpy.array([[[i,j] for j in range(0,data.shape[1]-img_size+1,sample_stride)] for i in range(0,data.shape[0]-img_size+1,channel_stride)])
idxs = idxs.reshape(idxs.shape[0]*idxs.shape[1],2)
for k,(i,j) in enumerate(idxs):
im = data[i:i+img_size,j:j+img_size].copy() # Create copy of square data window
im = (im-im.min())/(im.max()-im.min()) # Normalize data
im = Image.fromarray(numpy.uint8(cm.gist_earth(im)*255)).convert("RGB") # Convert data to RGB image
image = transforms.ToTensor()(im).float().unsqueeze(0) # Convert image to tensor and use first channel
output = model(image) # Run trained model to image
prob = torch.nn.functional.softmax(output,dim=1).topk(3) # Get probability for each class
assert int(prob[1][0,0]) in [0,1,2],"Maximum probability class has an unknown label..." # Check if label not found
wave_prob = float(prob[0][numpy.where(prob.indices==2)])
prob_array[0,i:i+img_size,j:j+img_size]+=wave_prob # Increment probability to map
prob_array[1,i:i+img_size,j:j+img_size]+=1 # Increment scanning index to map
prob_map = prob_array[0]/prob_array[1]
return prob_map
[ ]:
model = mldas.ResNet(depth=20,num_classes=3)
for k in range(len(loss_hist)):
state_dict = torch.load('training5/models/model%03i.pt'%k)
model.load_state_dict(state_dict)
model.eval()
probmap = prob_map(data,model)
plt.style.use('seaborn')
fig,ax = plt.subplots(2,2,figsize=(18,10),dpi=80)
ax[0][0].plot(loss_hist[:,3]/loss_hist[:,2],label='Training loss')
ax[0][0].plot(loss_hist[:,6]/loss_hist[:,5],label='Validation loss')
ax[0][0].axvline(k,color='black',lw=0.8)
ax[0][0].scatter([k,k],[loss_hist[k,3]/loss_hist[k,2],loss_hist[k,6]/loss_hist[k,5]],color='black',zorder=3)
ax[0][0].set_xlabel('Batch Iterations')
ax[0][0].set_ylabel('Loss')
ax[0][0].legend(frameon=False)
ax[0][1].plot(100*loss_hist[:,4]/loss_hist[:,2],label='Training accuracy')
ax[0][1].plot(100*loss_hist[:,7]/loss_hist[:,5],label='Validation accuracy')
ax[0][1].axvline(k,color='black',lw=0.8)
ax[0][1].scatter([k,k],[100*loss_hist[k,4]/loss_hist[k,2],100*loss_hist[k,7]/loss_hist[k,5]],color='black',zorder=3)
ax[0][1].set_xlabel('Batch Iterations')
ax[0][1].set_ylabel('Accuracy')
ax[0][1].legend(frameon=False)
ax[1][0].imshow(data,aspect='auto',cmap='seismic')
ax[1][0].set_title('Raw strain measurements')
ax[1][0].set_xlabel('Samples')
ax[1][0].set_ylabel('Channels')
ax[1][1].imshow(probmap,aspect='auto',cmap='jet',vmin=0,vmax=1)
ax[1][1].set_title('Probability map')
ax[1][1].set_xlabel('Samples')
plt.tight_layout()
plt.savefig('training5/maps/model%02i.png'%k,dpi=200)
plt.close()
[ ]:
%%capture
!ffmpeg -i training5/maps/model%02d.png training5/video.mp4
[ ]:
from IPython.display import HTML
from base64 import b64encode
mp4 = open('training5/video.mp4','rb').read()
data_url = "data:video/mp4;base64," + b64encode(mp4).decode()
HTML("""<video width=100%% controls loop><source src="%s" type="video/mp4"></video>""" % data_url)
[ ]:
new_model = models[209]
probmap = prob_map(data,new_model,channel_stride=1)
plt.style.use('seaborn')
fig,ax = plt.subplots(1,2,figsize=(18,6),dpi=80,sharex=True,sharey=True)
ax[0].imshow(data,aspect='auto',cmap='seismic')
ax[0].set_title('Raw data')
ax[0].set_xlabel('Time [second]')
ax[0].set_ylabel('Channels')
im = ax[1].imshow(probmap,aspect='auto',cmap='inferno',vmin=0,vmax=1)
ax[1].set_title('Probability map')
ax[1].set_xlabel('Time [second]')
plt.colorbar(im,ax=ax[1])
plt.tight_layout()
plt.show()
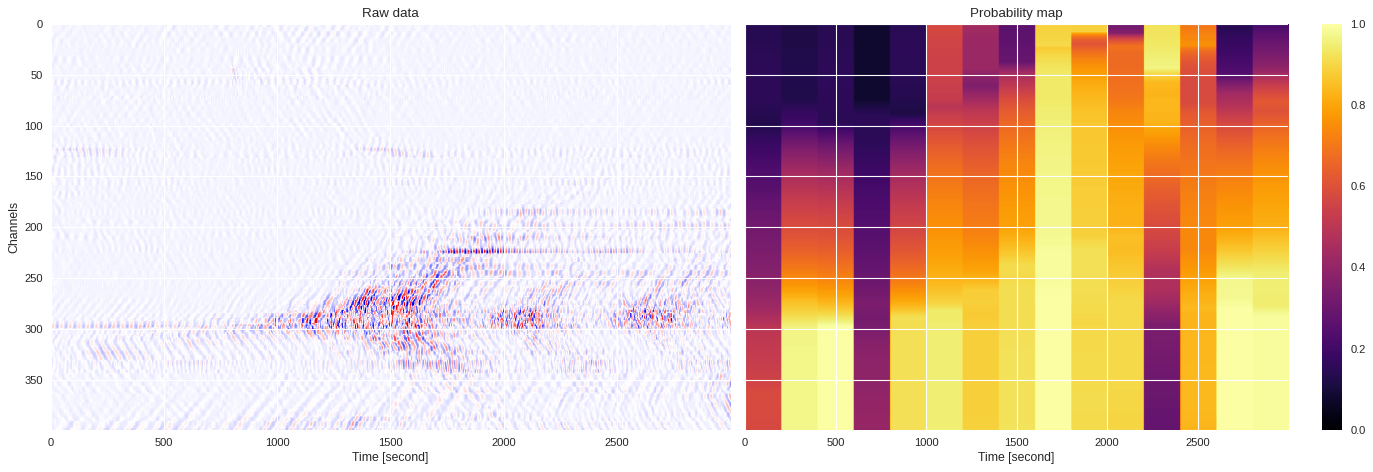
1-minute DAS file ¶
[ ]:
f = h5py.File('/content/drive/Shared drives/ML4DAS/RawData/1min_ch4650_4850/westSac_180112235258_ch4650_4850.mat','r')
data = numpy.array(f[f.get('variable/dat')[0,0]][:200])
f.close()
[ ]:
# new_model = models[209]
import mldas
new_model = mldas.ResNet(depth=20,num_classes=3)
new_model.load_state_dict(torch.load('training5/models/model209.pt'))
probmap = prob_map(data,new_model)
[ ]:
import matplotlib.pyplot as plt
from matplotlib.colors import LogNorm
plt.style.use('seaborn')
fig,ax = plt.subplots(1,2,figsize=(18,6),dpi=80,sharex=True,sharey=True)
ax[0].imshow(abs(data),cmap='plasma',aspect='auto',norm=LogNorm())
ax[0].set_title('Raw data')
ax[0].set_xlabel('Time [second]')
ax[0].set_ylabel('Channels')
im = ax[1].imshow(probmap,aspect='auto',cmap='inferno',vmin=0,vmax=1)
ax[1].set_title('Probability map')
ax[1].set_xlabel('Time [second]')
plt.colorbar(im,ax=ax[1])
plt.tight_layout()
plt.show()
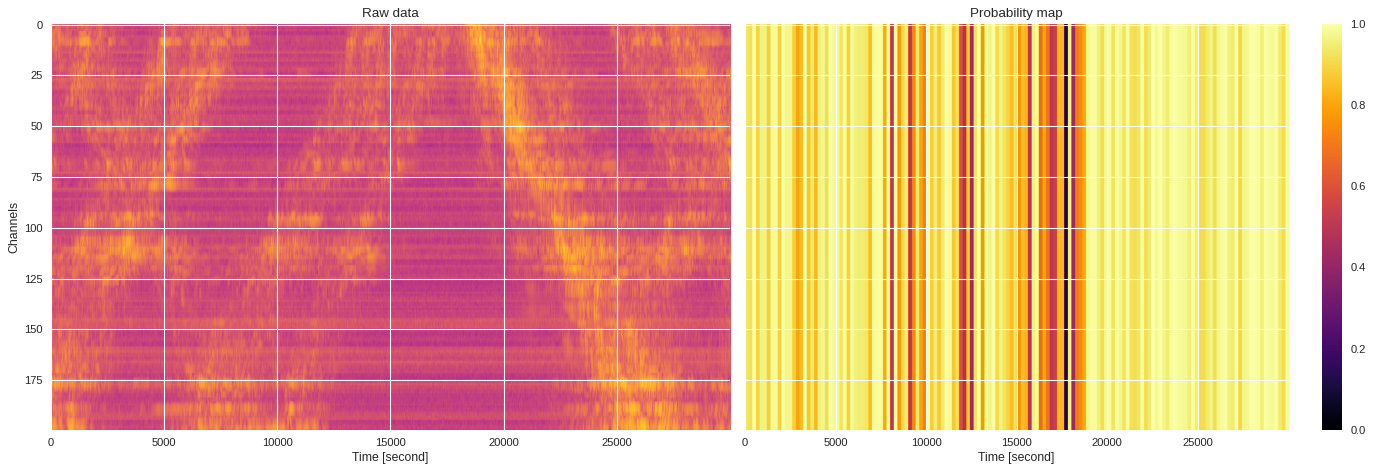
[ ]:
import matplotlib.pyplot as plt
from google.colab import widgets
div = 30
tb = widgets.TabBar([str(i) for i in range(div)])
for k in range(div):
with tb.output_to(k, select=False):
imin,imax = k*data.shape[1]//div,(k+1)*data.shape[1]//div
# Initialize figure
plt.style.use('seaborn')
fig = plt.figure(figsize=(17,8))
# Plot surface wave probability map
ax = fig.add_axes([0.1,0.1,0.85,0.8])
im = ax.imshow(probmap[:,imin:imax],extent=[imin,imax,200,0],aspect='auto',cmap='jet',vmin=0,vmax=1)
ax.set_xlabel('Time [second]')
ax.set_ylabel('Channels')
# Overlay original DAS data
newax = fig.add_axes(ax.get_position())
newax.imshow(data[:,imin:imax],aspect='auto',alpha=0.8)
newax.axis('off')
# Plot colorbar
cax = fig.add_axes([0.1,0.91,0.85,0.05])
cb = fig.colorbar(im,cax=cax,orientation="horizontal")
cax.xaxis.tick_top()
cax.set_title('Probability of wave signal',pad=10)
plt.show()
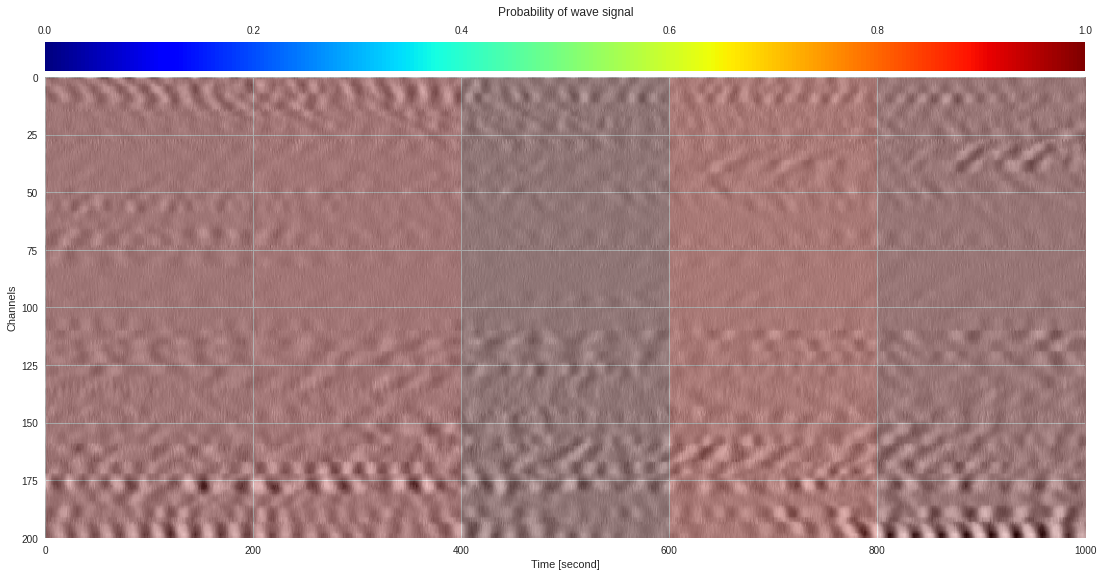
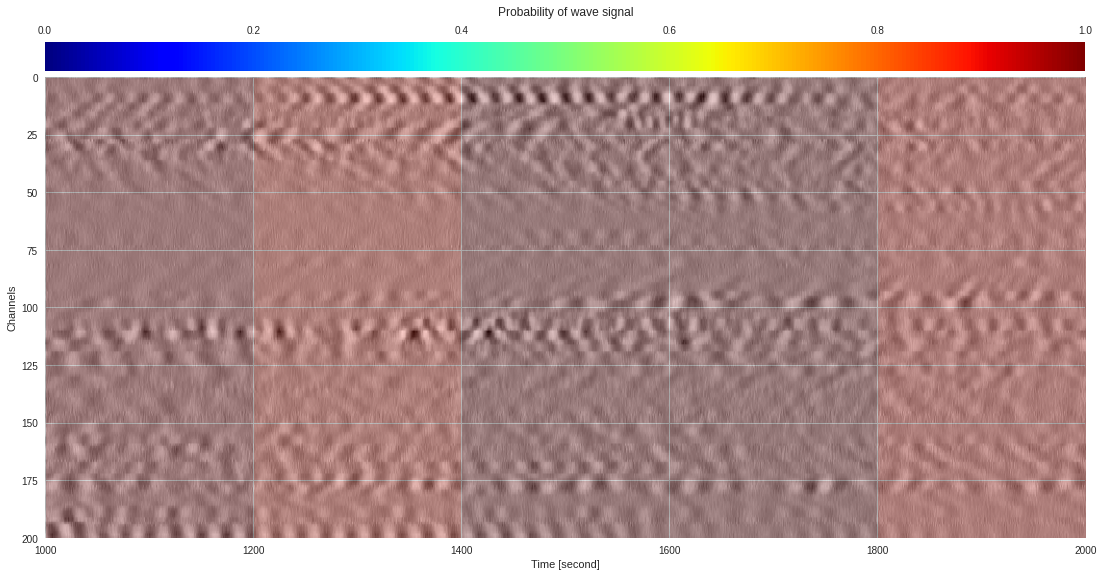
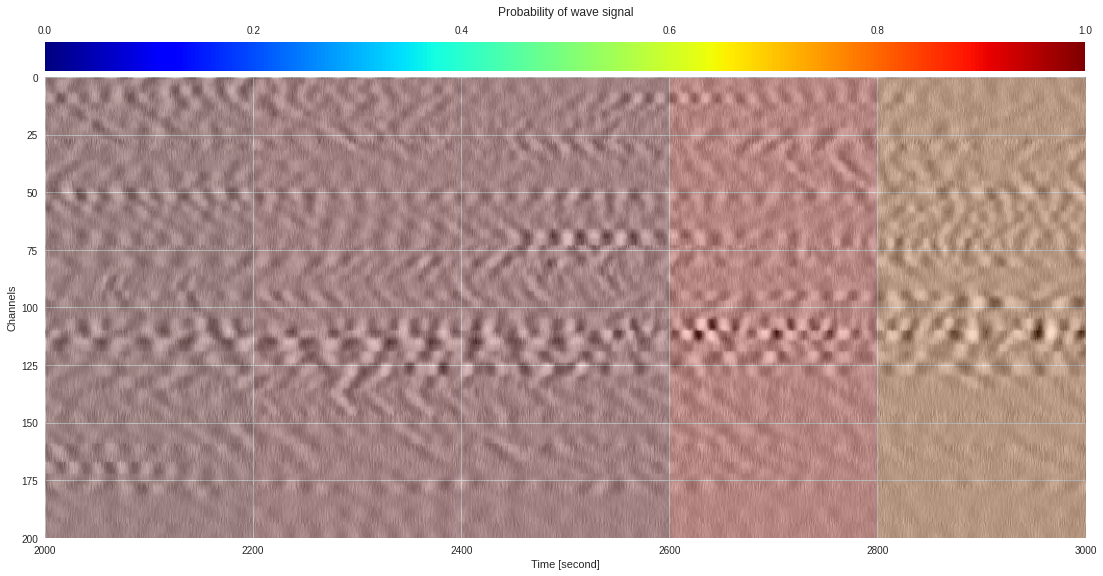
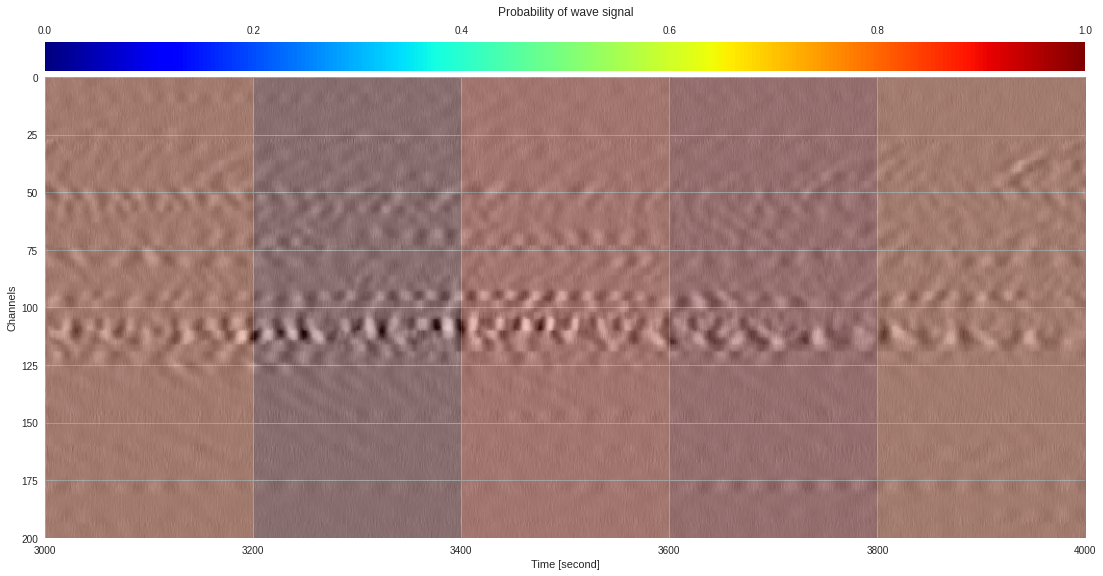
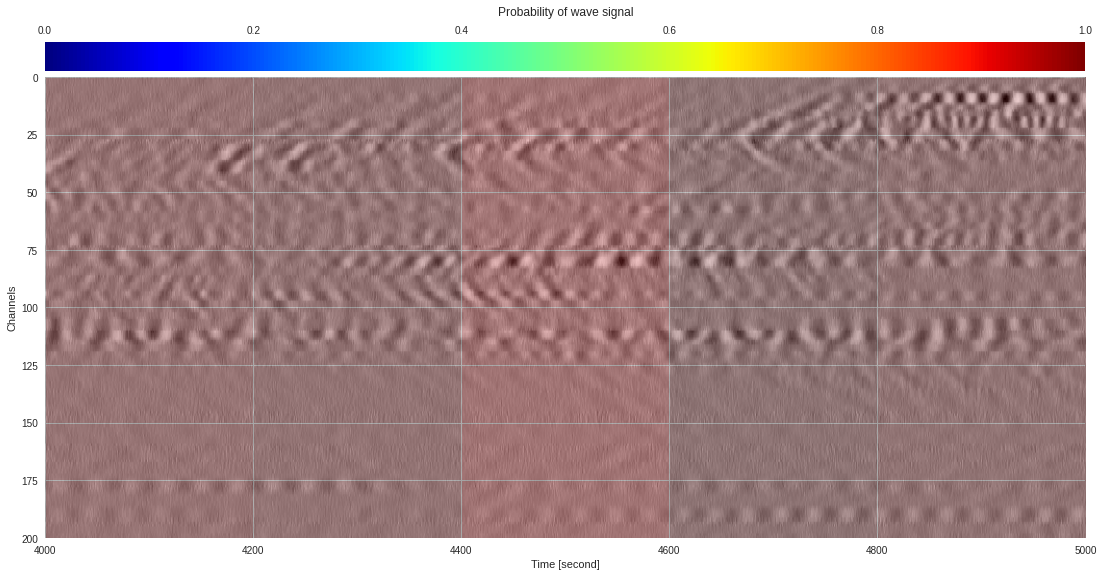
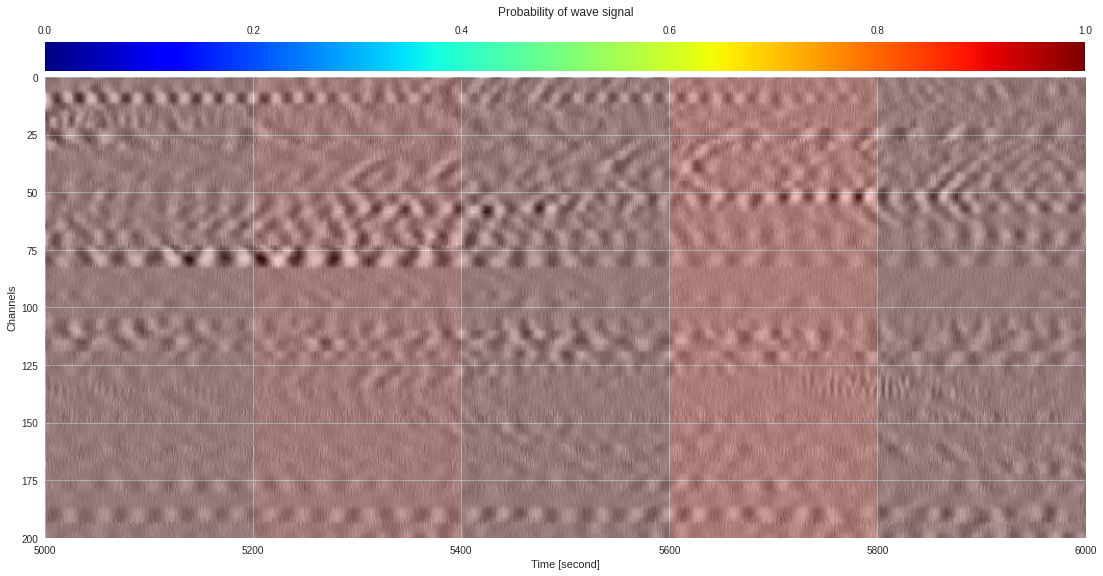
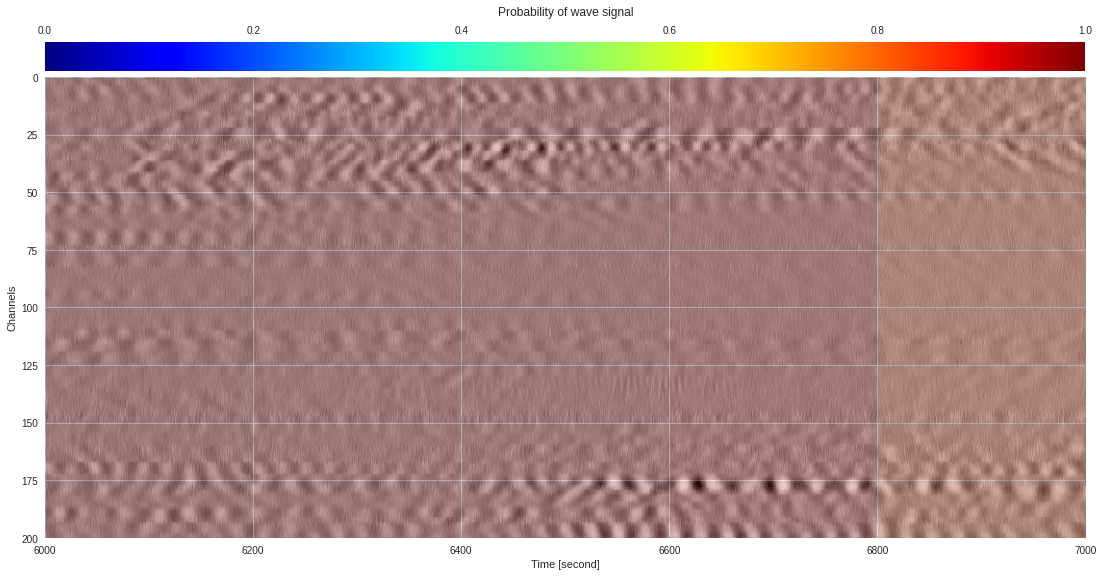
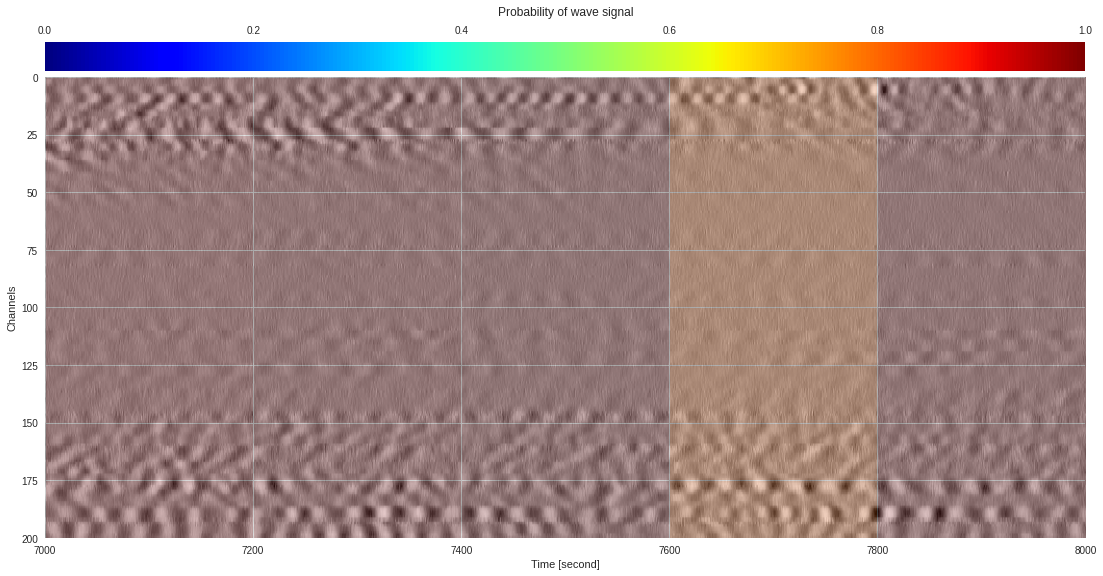
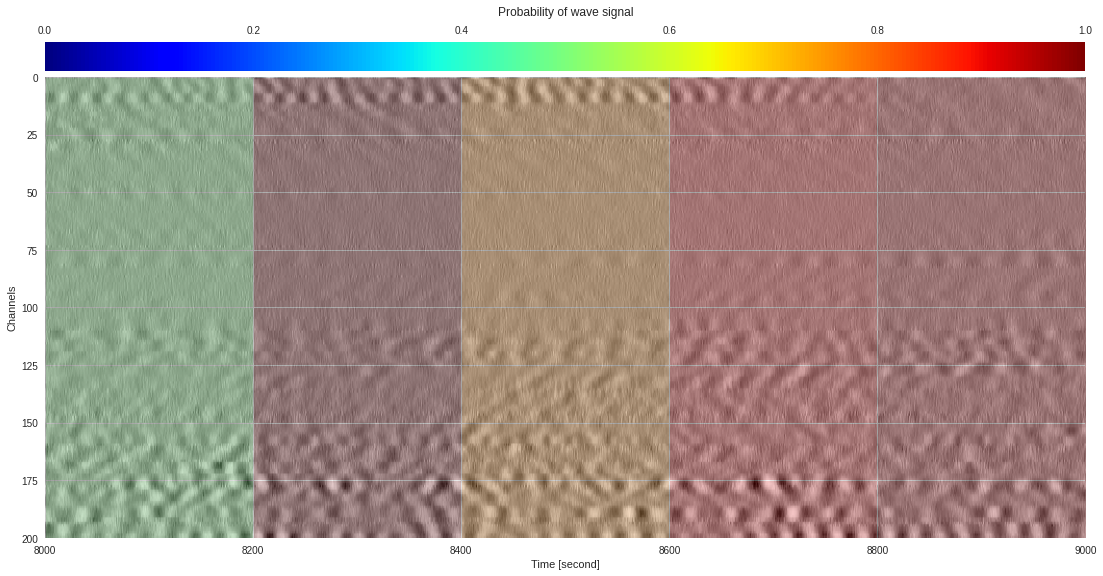
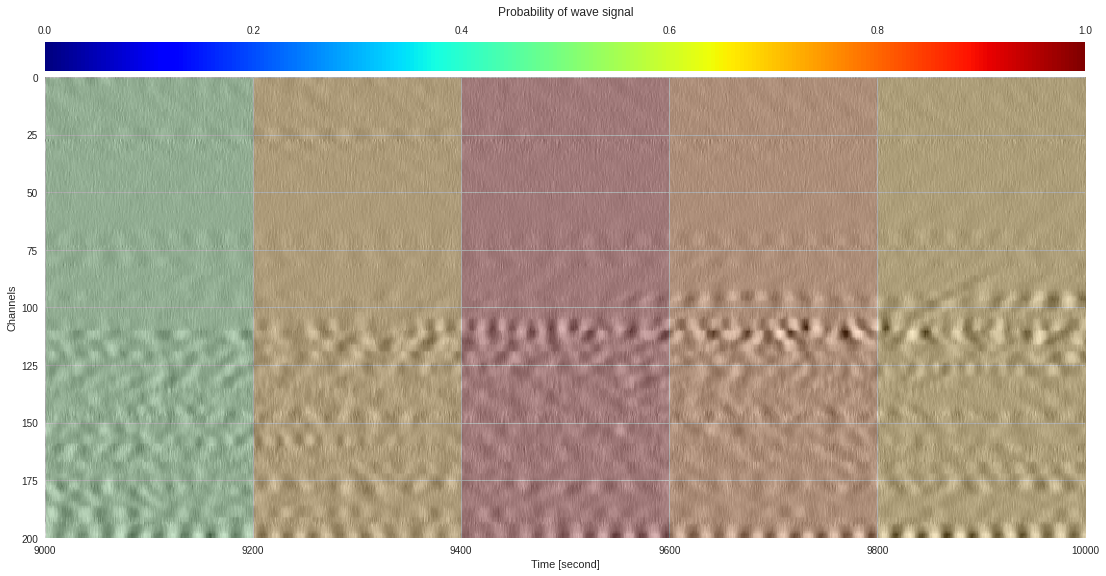
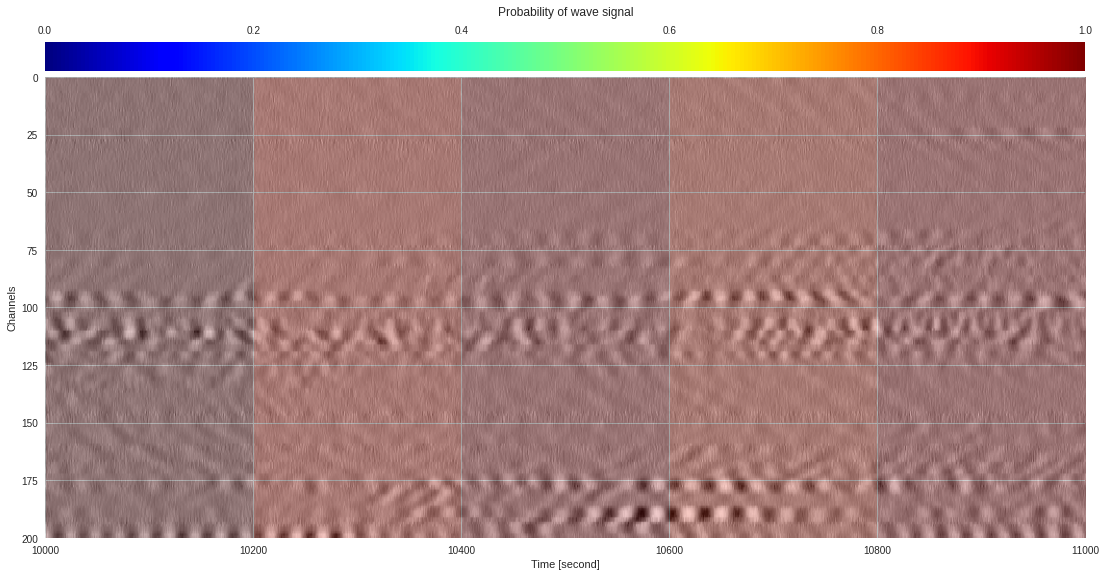
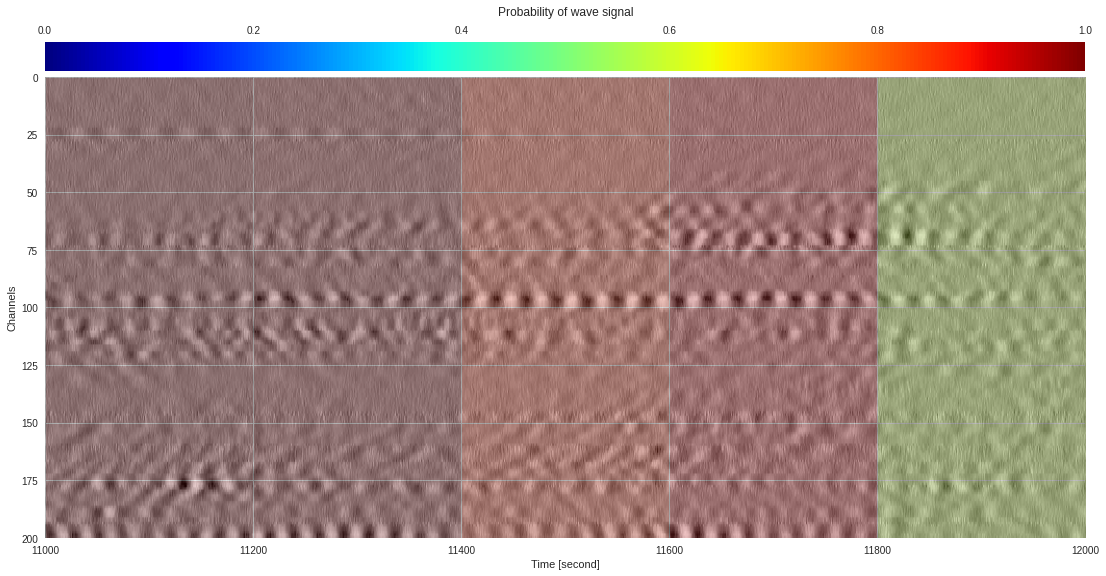
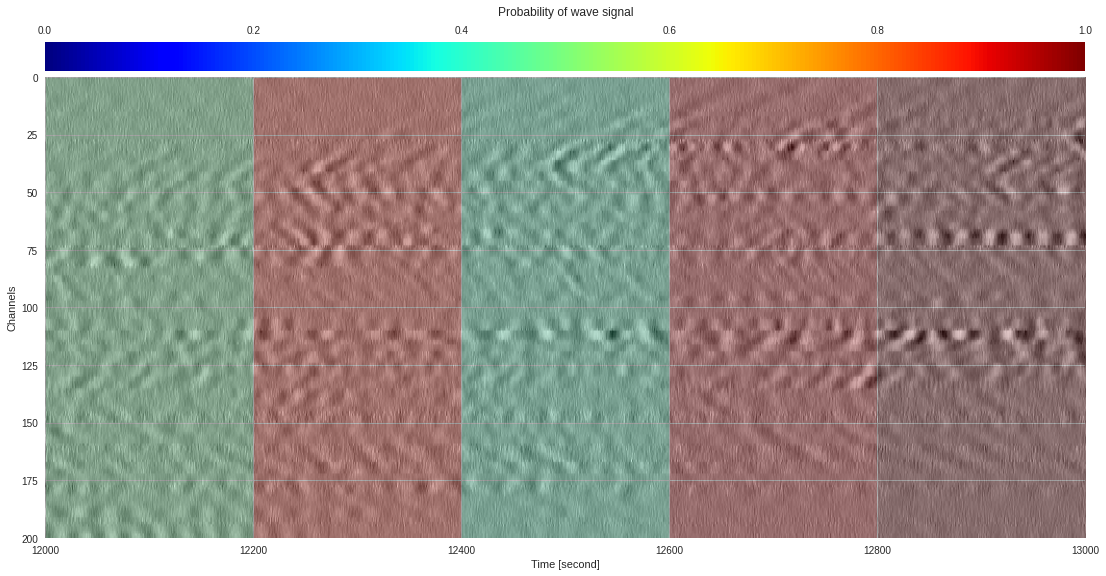
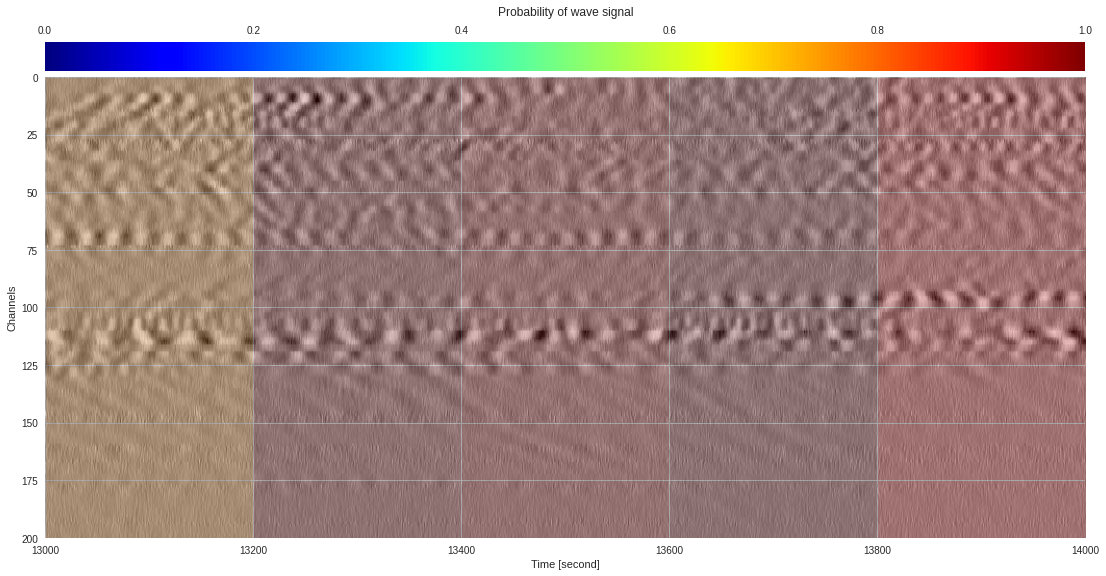
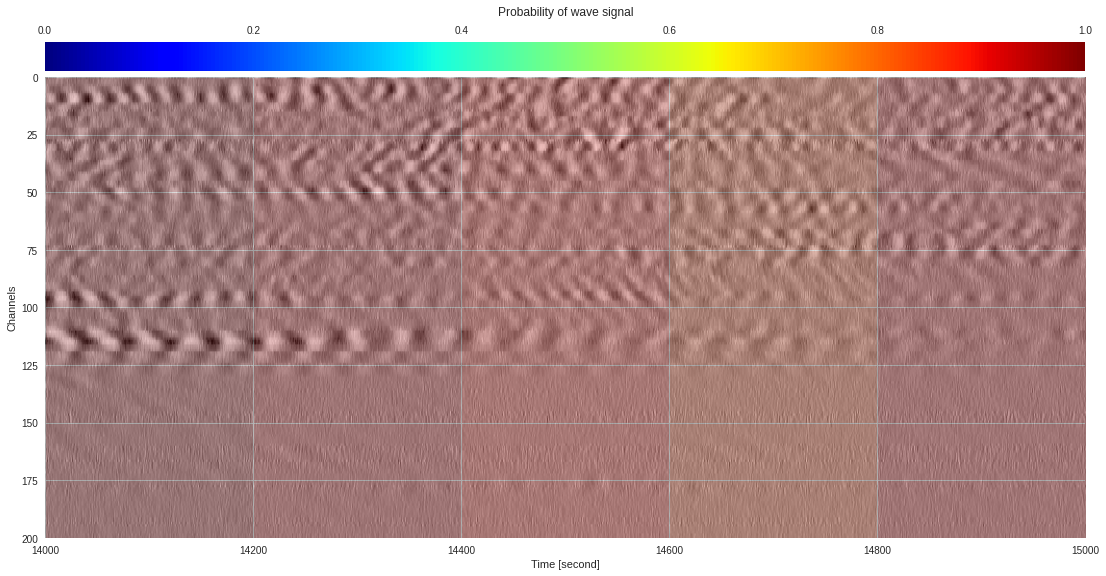
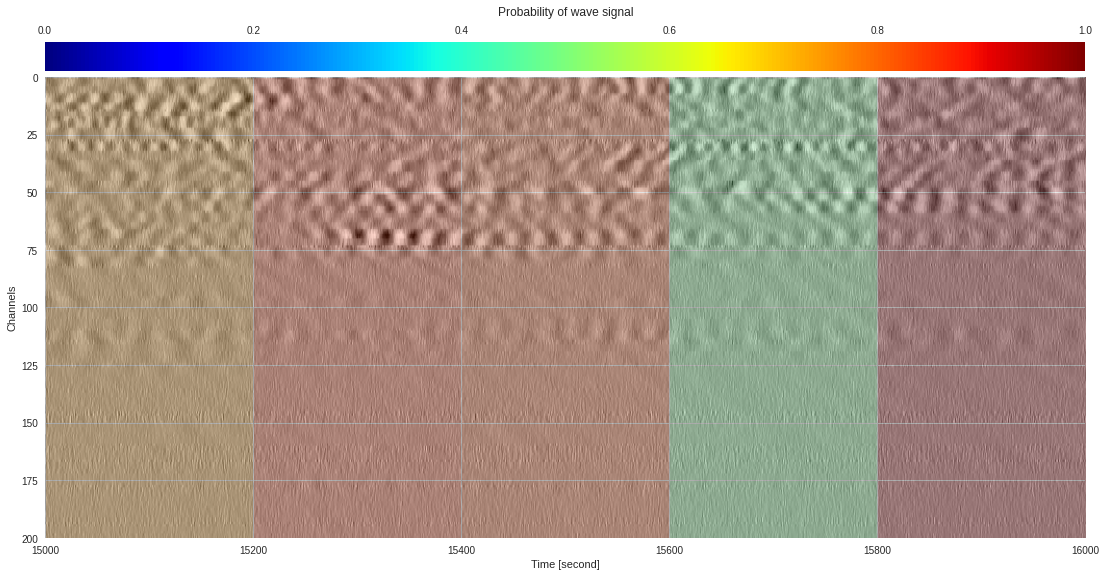
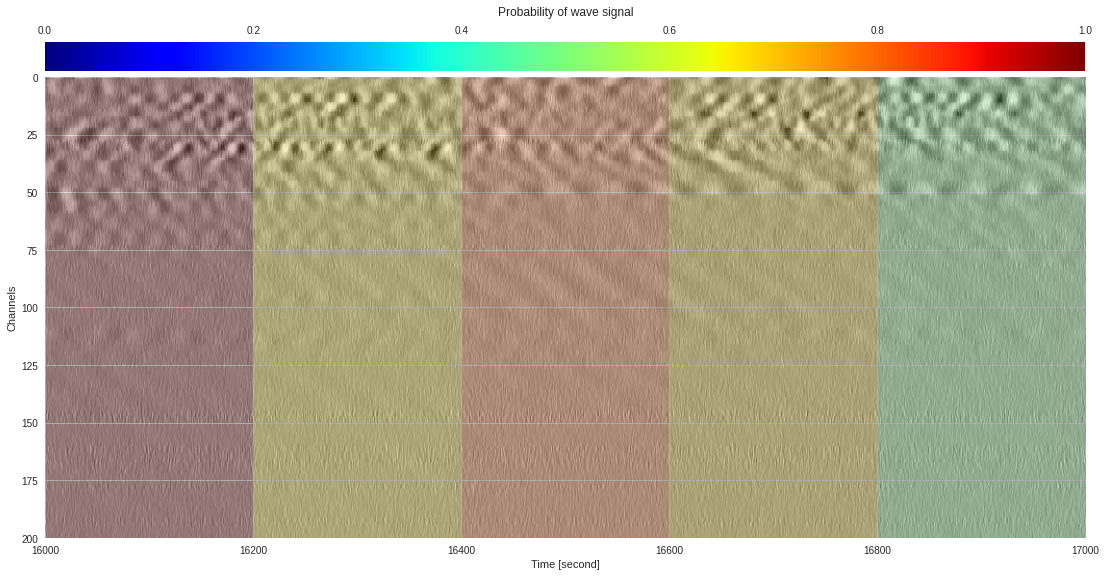
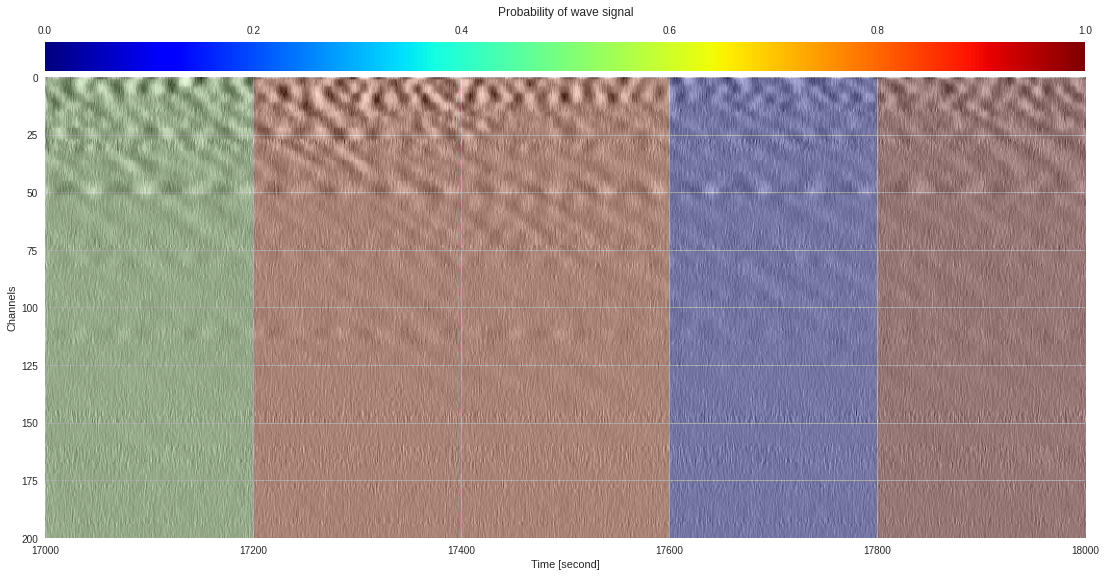
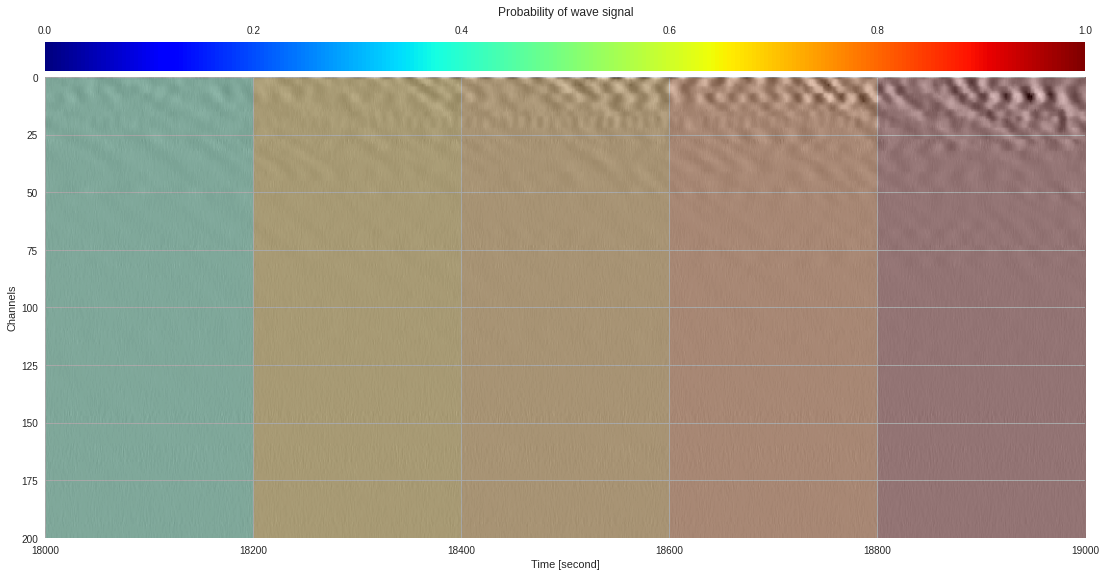
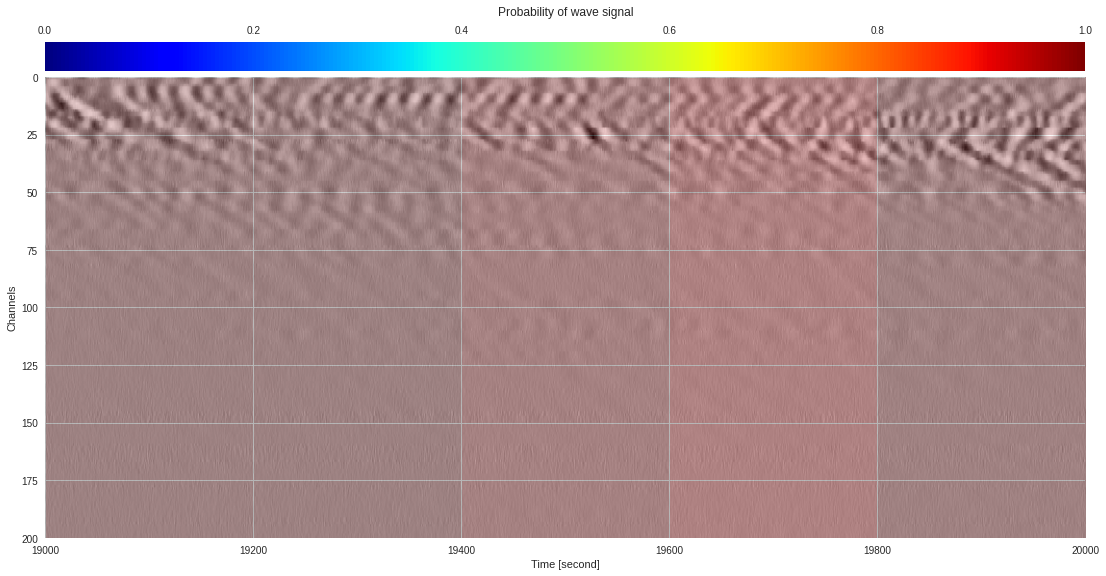
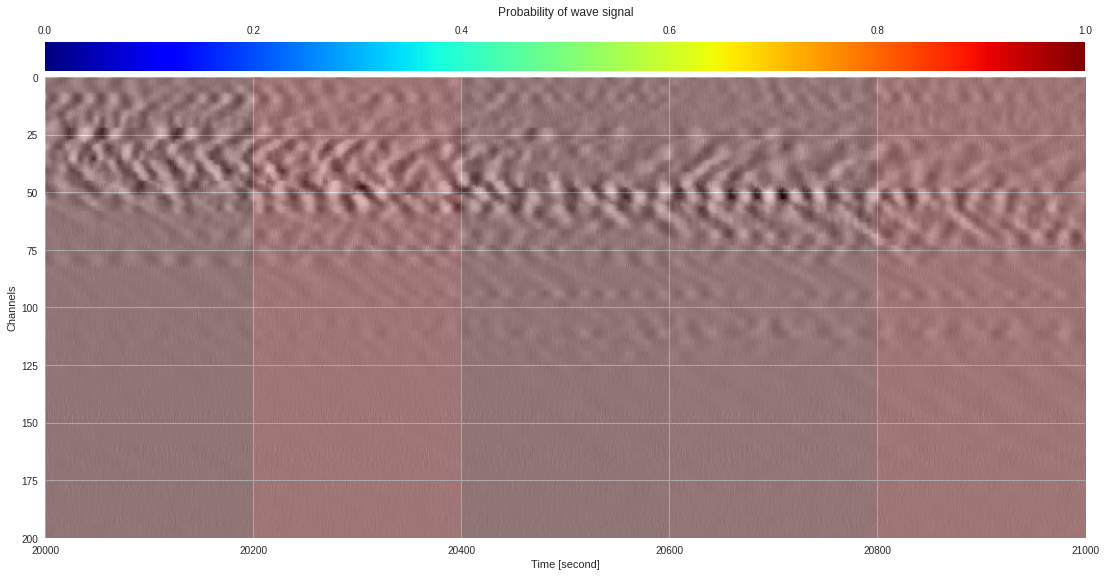
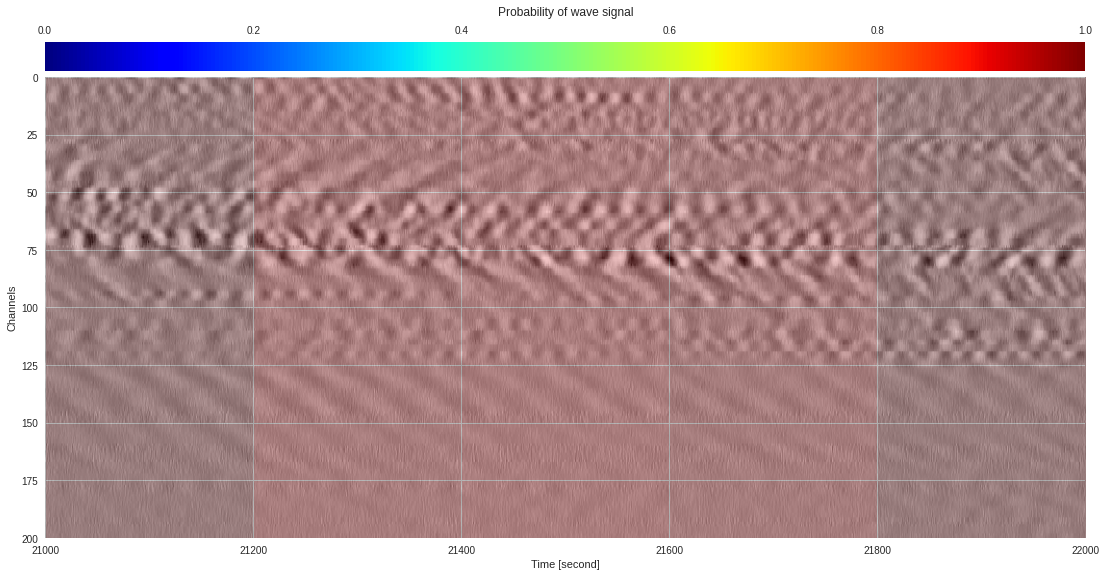
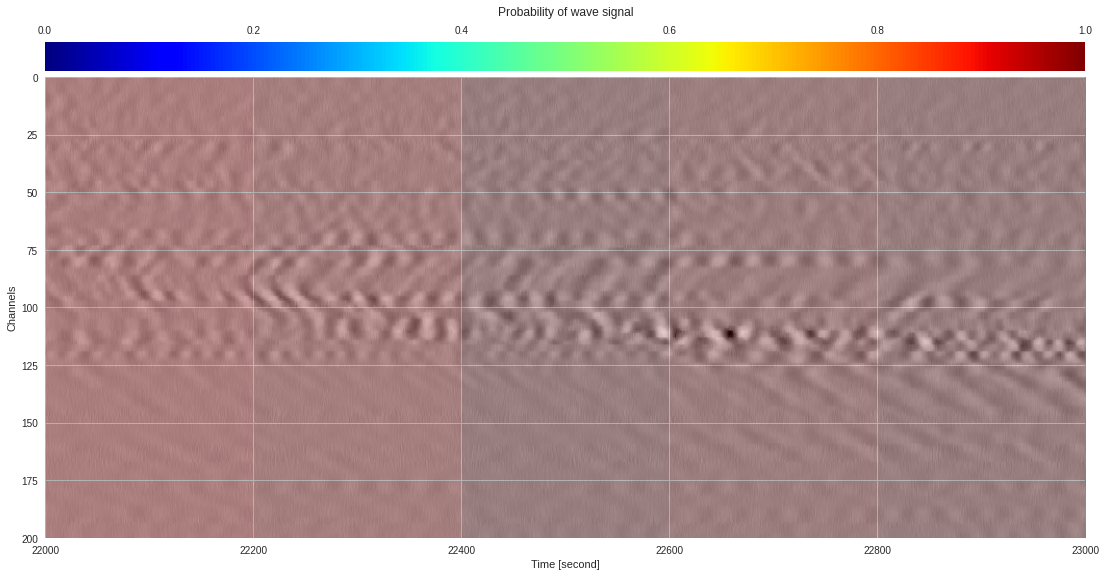
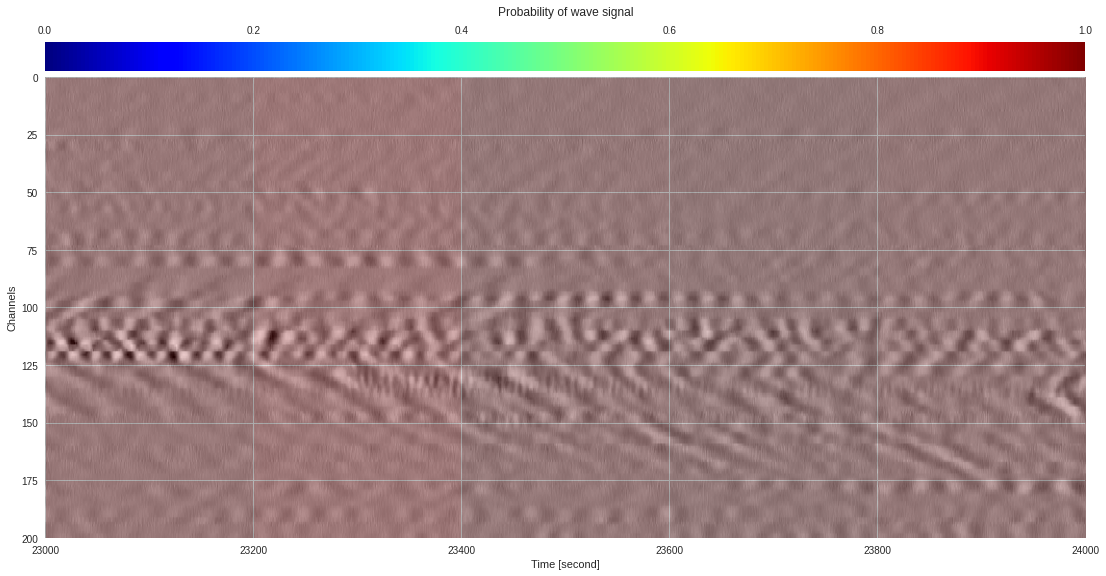
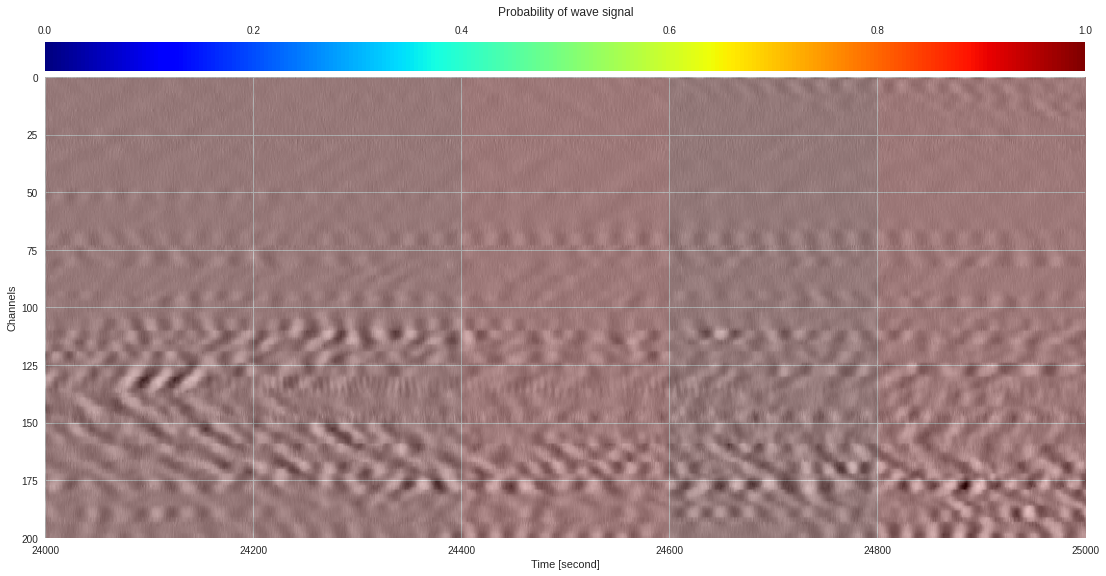
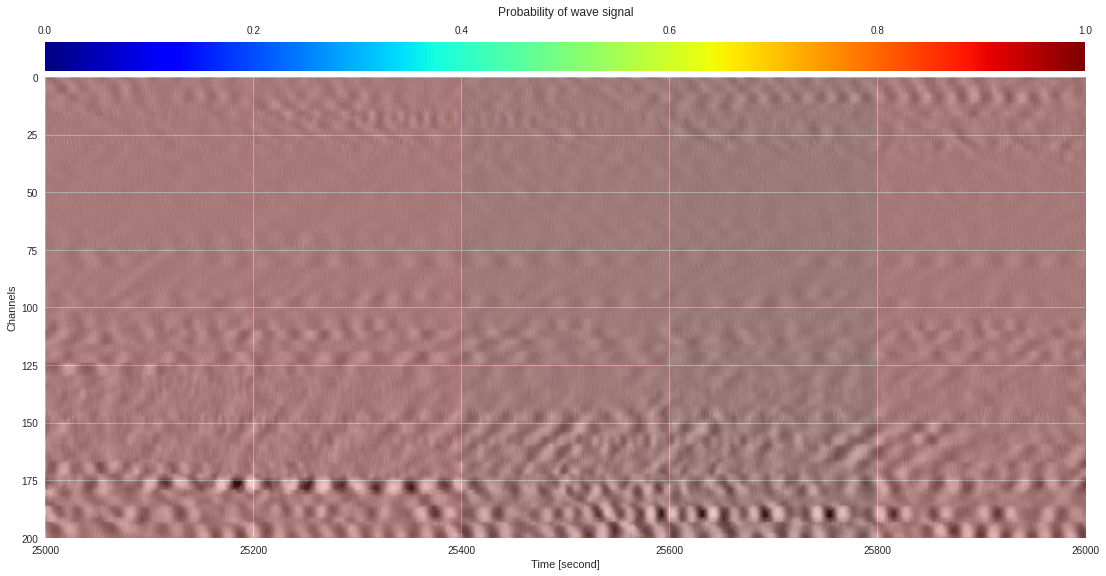
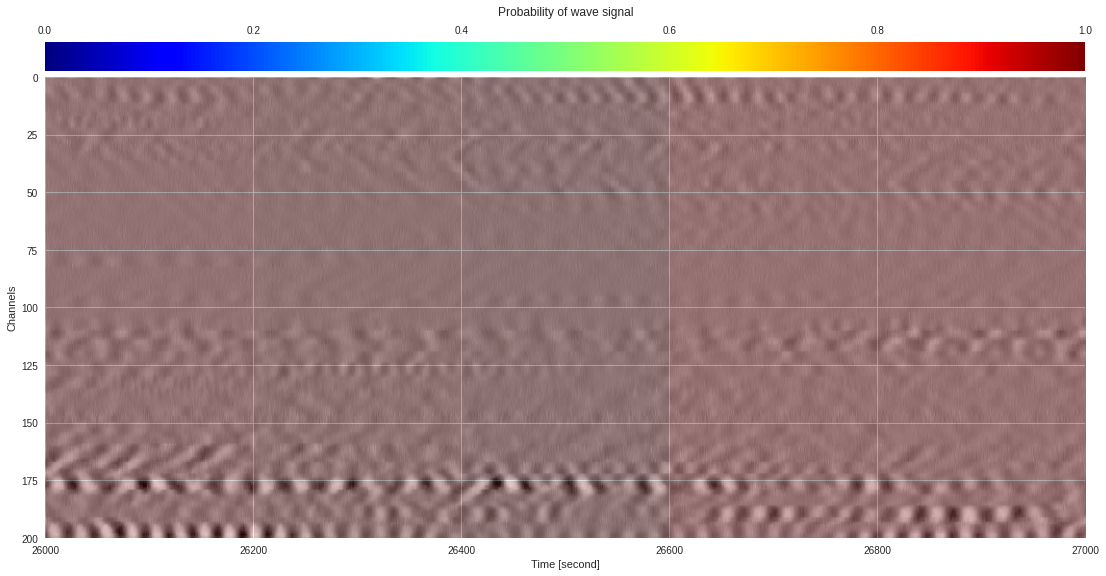
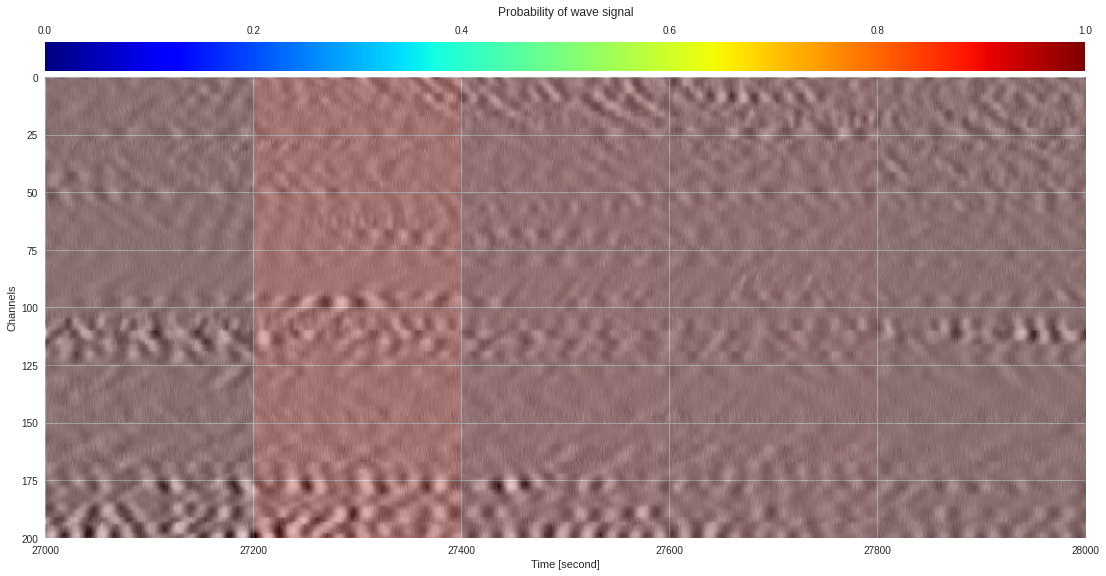
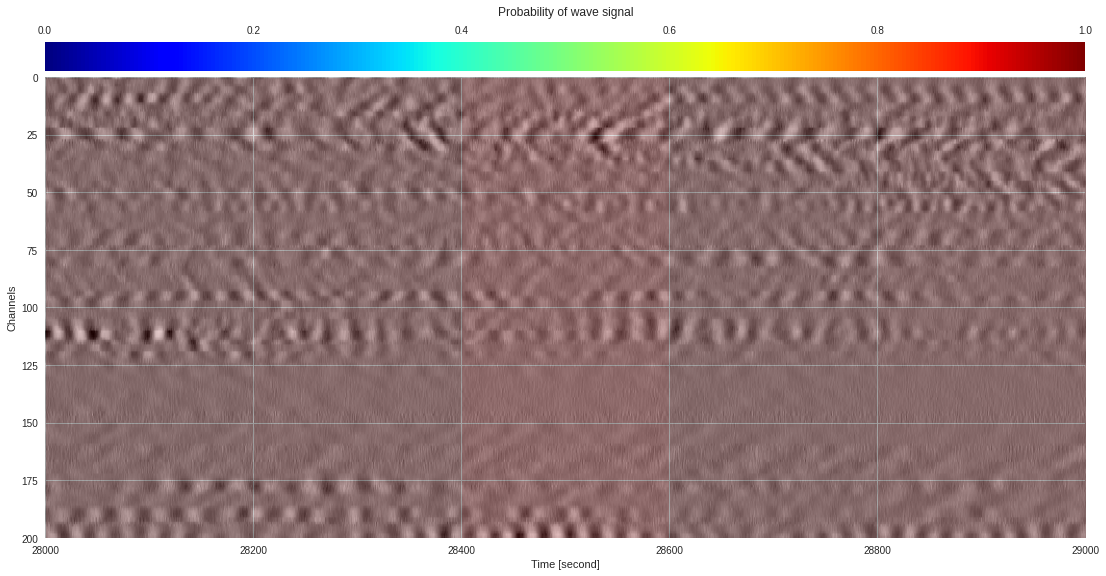
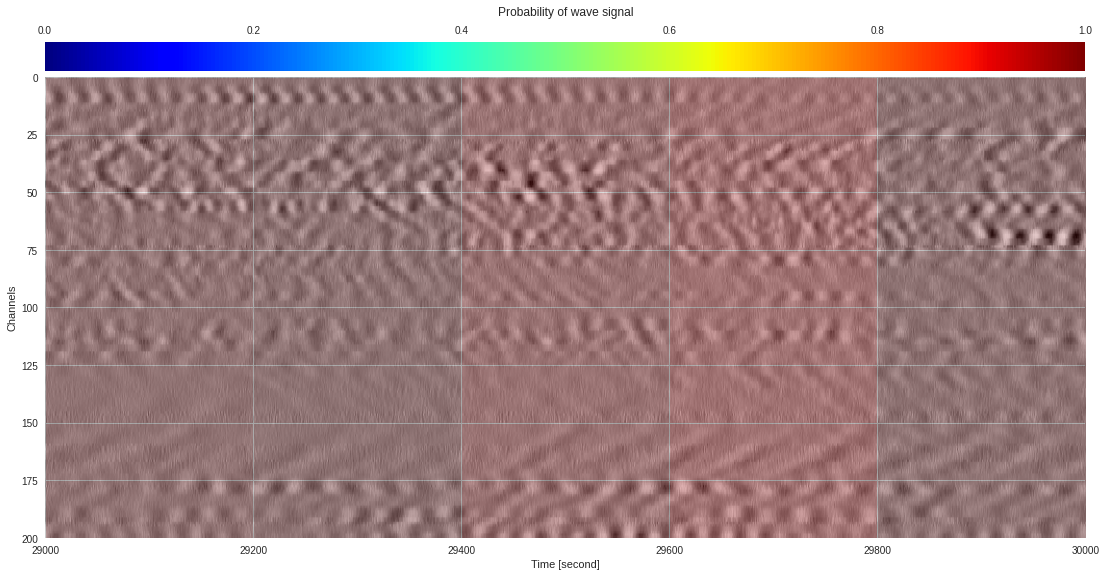
174k 2-class 200x200 dataset ¶
[ ]:
import h5py,numpy
f = h5py.File('/content/drive/Shared drives/ML4DAS/RawData/30min_files_NoTrain/Dsi_30min_170804230056_170804233056_ch5500_6000.mat','r')
data = numpy.array(f[f.get('dsi30/dat')[0,0]][200:500,330900:331800])
f.close()
Training 1: 2-layer 1-epoch model with 0.01 learning rate ¶
[ ]:
import mldas,torch
model = mldas.ResNet(depth=2,num_classes=2)
model.load_state_dict(torch.load('Cori Trained Models/model-hsw-n10-ds174-ep1-dp2-lr0.01.pt'))
prob_map = extract_prob_map(data,model,img_size=50,channel_stride=5,sample_stride=10)
plot_prob_map(data,prob_map)
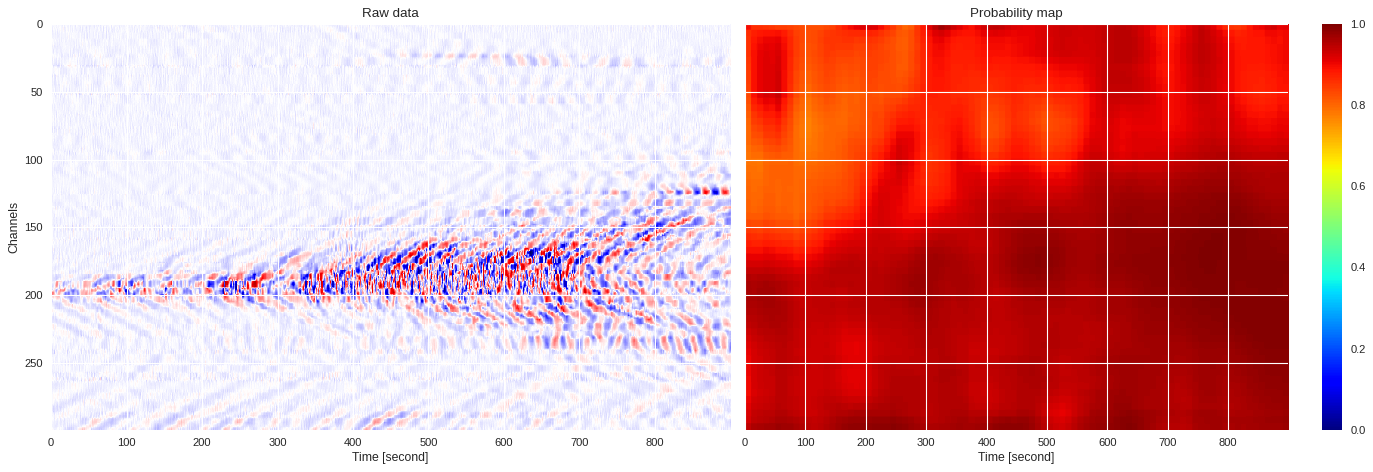
[ ]:
import mldas,torch
from torchsummary import summary
from collections import OrderedDict
model = mldas.ResNet(depth=2,num_classes=2)
checkpoint = torch.load('Cori Trained Models/model_checkpoint_049.pth.tar',map_location=lambda storage, loc: storage)
state_dict = checkpoint['model']
new_state_dict = OrderedDict()
for k, v in state_dict.items():
if k.startswith('module.'):
k = k[7:]
new_state_dict[k] = v
checkpoint['model'] = new_state_dict
model.load_state_dict(checkpoint['model'])
prob_map = extract_prob_map(data,model,img_size=50,channel_stride=5,sample_stride=10)
plot_prob_map(data,prob_map)
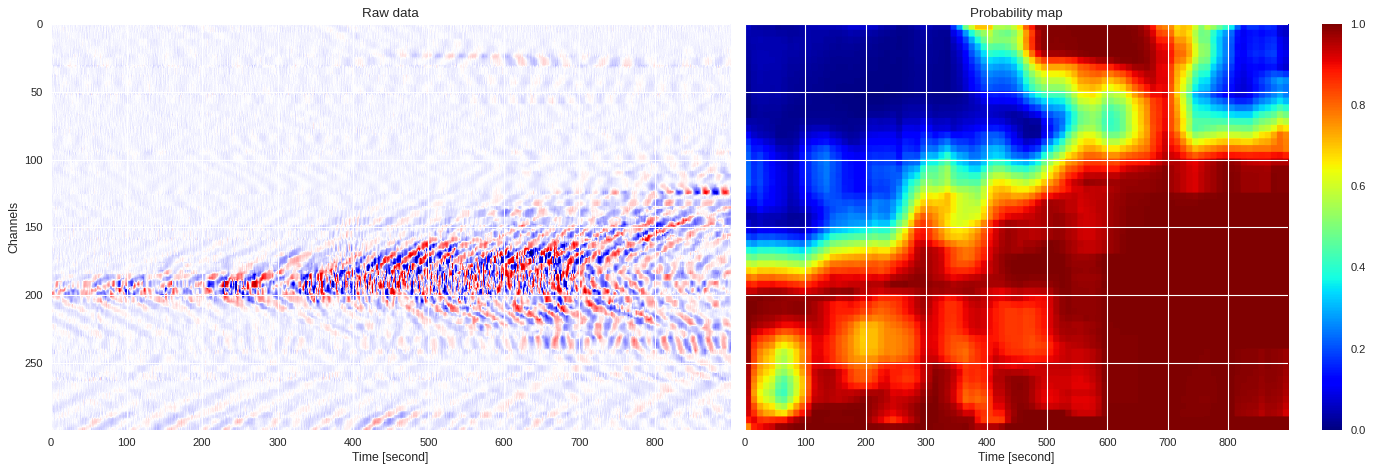
Full day probability map ¶
Load trained model ¶
[ ]:
import mldas,torch
from collections import OrderedDict
model = mldas.ResNet(depth=14,num_classes=2,num_channels=3)
checkpoint = torch.load('Cori Trained Models/multiclass-c2-gpu-n8-ds1-bs128-ep50-dp14-lr0.001.pth.tar',map_location=lambda storage, loc: storage)
# checkpoint = torch.load('Cori Trained Models/multiclass-gpu-n16-ds5-bs128-ep50-dp14-lr0.01.pth.tar',map_location=lambda storage, loc: storage)
state_dict = checkpoint['model']
new_state_dict = OrderedDict()
for k, v in state_dict.items():
if k.startswith('module.'):
k = k[7:]
new_state_dict[k] = v
checkpoint['model'] = new_state_dict
model.load_state_dict(checkpoint['model'])
model.eval()
ResNet(
(conv1): Conv2d(3, 16, kernel_size=(3, 3), stride=(1, 1), padding=(1, 1), bias=False)
(bn1): BatchNorm2d(16, eps=1e-05, momentum=0.1, affine=True, track_running_stats=True)
(relu): ReLU(inplace=True)
(layer1): Sequential(
(0): BasicBlock(
(conv1): Conv2d(16, 16, kernel_size=(3, 3), stride=(1, 1), padding=(1, 1), bias=False)
(bn1): BatchNorm2d(16, eps=1e-05, momentum=0.1, affine=True, track_running_stats=True)
(relu): ReLU(inplace=True)
(conv2): Conv2d(16, 16, kernel_size=(3, 3), stride=(1, 1), padding=(1, 1), bias=False)
(bn2): BatchNorm2d(16, eps=1e-05, momentum=0.1, affine=True, track_running_stats=True)
)
(1): BasicBlock(
(conv1): Conv2d(16, 16, kernel_size=(3, 3), stride=(1, 1), padding=(1, 1), bias=False)
(bn1): BatchNorm2d(16, eps=1e-05, momentum=0.1, affine=True, track_running_stats=True)
(relu): ReLU(inplace=True)
(conv2): Conv2d(16, 16, kernel_size=(3, 3), stride=(1, 1), padding=(1, 1), bias=False)
(bn2): BatchNorm2d(16, eps=1e-05, momentum=0.1, affine=True, track_running_stats=True)
)
)
(layer2): Sequential(
(0): BasicBlock(
(conv1): Conv2d(16, 32, kernel_size=(3, 3), stride=(2, 2), padding=(1, 1), bias=False)
(bn1): BatchNorm2d(32, eps=1e-05, momentum=0.1, affine=True, track_running_stats=True)
(relu): ReLU(inplace=True)
(conv2): Conv2d(32, 32, kernel_size=(3, 3), stride=(1, 1), padding=(1, 1), bias=False)
(bn2): BatchNorm2d(32, eps=1e-05, momentum=0.1, affine=True, track_running_stats=True)
(downsample): Sequential(
(0): Conv2d(16, 32, kernel_size=(1, 1), stride=(2, 2), bias=False)
(1): BatchNorm2d(32, eps=1e-05, momentum=0.1, affine=True, track_running_stats=True)
)
)
(1): BasicBlock(
(conv1): Conv2d(32, 32, kernel_size=(3, 3), stride=(1, 1), padding=(1, 1), bias=False)
(bn1): BatchNorm2d(32, eps=1e-05, momentum=0.1, affine=True, track_running_stats=True)
(relu): ReLU(inplace=True)
(conv2): Conv2d(32, 32, kernel_size=(3, 3), stride=(1, 1), padding=(1, 1), bias=False)
(bn2): BatchNorm2d(32, eps=1e-05, momentum=0.1, affine=True, track_running_stats=True)
)
)
(layer3): Sequential(
(0): BasicBlock(
(conv1): Conv2d(32, 64, kernel_size=(3, 3), stride=(2, 2), padding=(1, 1), bias=False)
(bn1): BatchNorm2d(64, eps=1e-05, momentum=0.1, affine=True, track_running_stats=True)
(relu): ReLU(inplace=True)
(conv2): Conv2d(64, 64, kernel_size=(3, 3), stride=(1, 1), padding=(1, 1), bias=False)
(bn2): BatchNorm2d(64, eps=1e-05, momentum=0.1, affine=True, track_running_stats=True)
(downsample): Sequential(
(0): Conv2d(32, 64, kernel_size=(1, 1), stride=(2, 2), bias=False)
(1): BatchNorm2d(64, eps=1e-05, momentum=0.1, affine=True, track_running_stats=True)
)
)
(1): BasicBlock(
(conv1): Conv2d(64, 64, kernel_size=(3, 3), stride=(1, 1), padding=(1, 1), bias=False)
(bn1): BatchNorm2d(64, eps=1e-05, momentum=0.1, affine=True, track_running_stats=True)
(relu): ReLU(inplace=True)
(conv2): Conv2d(64, 64, kernel_size=(3, 3), stride=(1, 1), padding=(1, 1), bias=False)
(bn2): BatchNorm2d(64, eps=1e-05, momentum=0.1, affine=True, track_running_stats=True)
)
)
(avgpool): AdaptiveAvgPool2d(output_size=1)
(fc): Linear(in_features=64, out_features=2, bias=True)
)
Probabilities per file ¶
[ ]:
import h5py,numpy,os,glob
import torch.nn.functional as F
import matplotlib.pyplot as plt
from PIL import Image
from matplotlib import cm
from matplotlib.colors import LogNorm
from torchvision import transforms
dataset = sorted(glob.glob('/content/drive/Shared drives/ML4DAS/RawData/1min_ch4650_4850/westSac_180104*'))
results = numpy.empty((0,2))
for n,fname in enumerate(dataset):
# print(n+1,os.path.basename(fname))
f = h5py.File(fname,'r')
print(type(f[f.get('variable/dat')[0,0]][0,0]))
data = numpy.array(f[f.get('variable/dat')[0,0]][:200])
f.close()
break
file_prob = 0
for i in range(0,data.shape[1],200):
image = data[:,i:i+200].copy()
image = (image-image.min())/(image.max()-image.min())
image = Image.fromarray(numpy.uint8(image*255))
image = transforms.ToTensor()(image.convert("RGB")).view(1,3,200,200)
# image = transforms.Compose([transforms.Grayscale(), transforms.ToTensor()])(image).view(1,1,200,200)
output = model(image)
wave_prob = F.softmax(output,dim=1)[0,1].item()
file_prob += wave_prob
results = numpy.vstack((results,[os.path.basename(fname),wave_prob]))
file_prob /= (data.shape[1]//200)
print(n+1,os.path.basename(fname),file_prob)
if (n+1)%1440==0:
print(os.path.basename(fname)[8:14])
numpy.savetxt('24hrs_%s.txt'%os.path.basename(fname)[8:14],results,fmt='%s')
results = numpy.empty((0,2))
<class 'numpy.float64'>
24-hour evolution ¶
[ ]:
import numpy,re,datetime
data = numpy.loadtxt('24hrs_results_1.txt',dtype=str)
date = [int(i) for i in re.findall('..?',data[0,0].split('_')[1])]
date = datetime.datetime(2000+date[0],*date[1:])+datetime.timedelta(hours=-8)
dates, probs = [], []
for n,(fname, prob) in enumerate(data):
dates.append(date+datetime.timedelta(seconds=n*200./500))
probs.append(float(prob))
[ ]:
import numpy
results = numpy.vstack((dates[::150],[numpy.mean(probs[i:i+150]) for i in range(0,len(probs),150)])).T
[ ]:
import numpy
from scipy import signal,ndimage
y = signal.resample(probs,len(probs)//500)
b = signal.gaussian(39, 10)
y = ndimage.filters.convolve1d(y, b/b.sum())
x = dates[::len(dates)//len(y)]
[ ]:
import matplotlib.pyplot as plt
import matplotlib.dates as mdates
plt.style.use('seaborn')
plt.rc('font', size=15)
plt.rc('axes', labelsize=15)
plt.rc('legend', fontsize=15)
plt.rc('xtick', labelsize=15)
plt.rc('ytick', labelsize=15)
fig,ax = plt.subplots(figsize=(14,6),dpi=100)
ax.plot(dates[::150],mean_probs)
ax.plot(x,y,color='red')
ax.xaxis.set_major_formatter(mdates.DateFormatter('%A\n%Y-%m-%d\n%H:%M:%S'))
ax.set_ylabel('Surface wave probability')
ax.set_xlim(dates[0],dates[-1])
plt.tight_layout()
plt.savefig('24hr_prob_1.pdf')
plt.show()
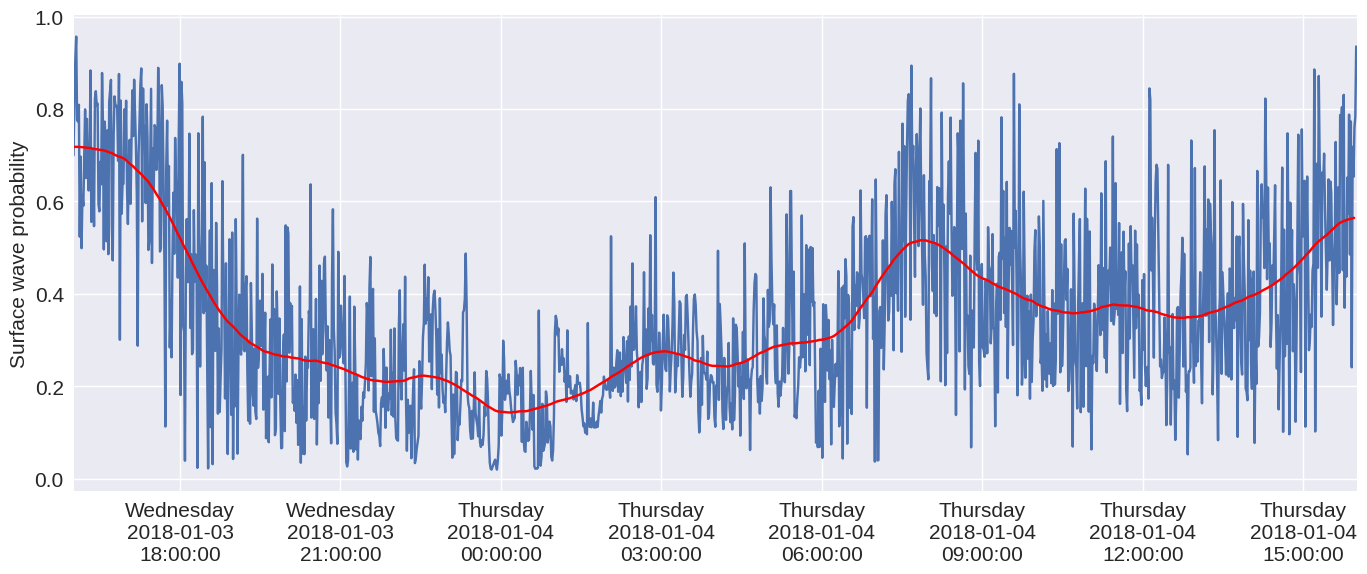
Multi-day evolution ¶
[ ]:
%%capture
!tar -zxvf probs.tar.gz -C /content
[ ]:
import glob
file_list = glob.glob('/content/probmaps/*.txt')
print(len(file_list),'files')
14399 files
[ ]:
import numpy,re,datetime
results = numpy.empty((0,2))
for fname in sorted(file_list):
date = [int(i) for i in re.findall('..?',fname.split('_')[1])]
date = datetime.datetime(2000+date[0],*date[1:])+datetime.timedelta(hours=-8)
prob = numpy.mean(numpy.loadtxt(fname))
results = numpy.vstack((results,[date,prob]))
print(results.shape)
(14399, 2)
[ ]:
import numpy
from scipy import signal,ndimage
y = signal.resample(results[:,1],len(results[:,1])//10)
b = signal.gaussian(39, 10)
y = ndimage.filters.convolve1d(y, b/b.sum())
x = results[::len(results)//len(y),0][:-1]
print(len(x),len(y))
1439 1439
[ ]:
import matplotlib.pyplot as plt
import matplotlib.dates as mdates
plt.style.use('seaborn')
plt.rc('font', size=15)
plt.rc('axes', labelsize=15)
plt.rc('legend', fontsize=15)
plt.rc('xtick', labelsize=15)
plt.rc('ytick', labelsize=15)
fig,ax = plt.subplots(figsize=(14,6),dpi=100)
ax.plot(results[:,0],results[:,1])
ax.plot(x,y,color='yellow')
# ax.xaxis.set_major_formatter(mdates.DateFormatter('%A\n%Y-%m-%d\n%I %p'))
ax.xaxis.set_major_formatter(mdates.DateFormatter('%A\n%Y-%m-%d'))
ax.set_ylabel('Surface wave probability')
ax.set_xlim(results[0,0],results[-1,0])
plt.tight_layout()
plt.savefig('/content/prob_time_series.pdf')
plt.show()
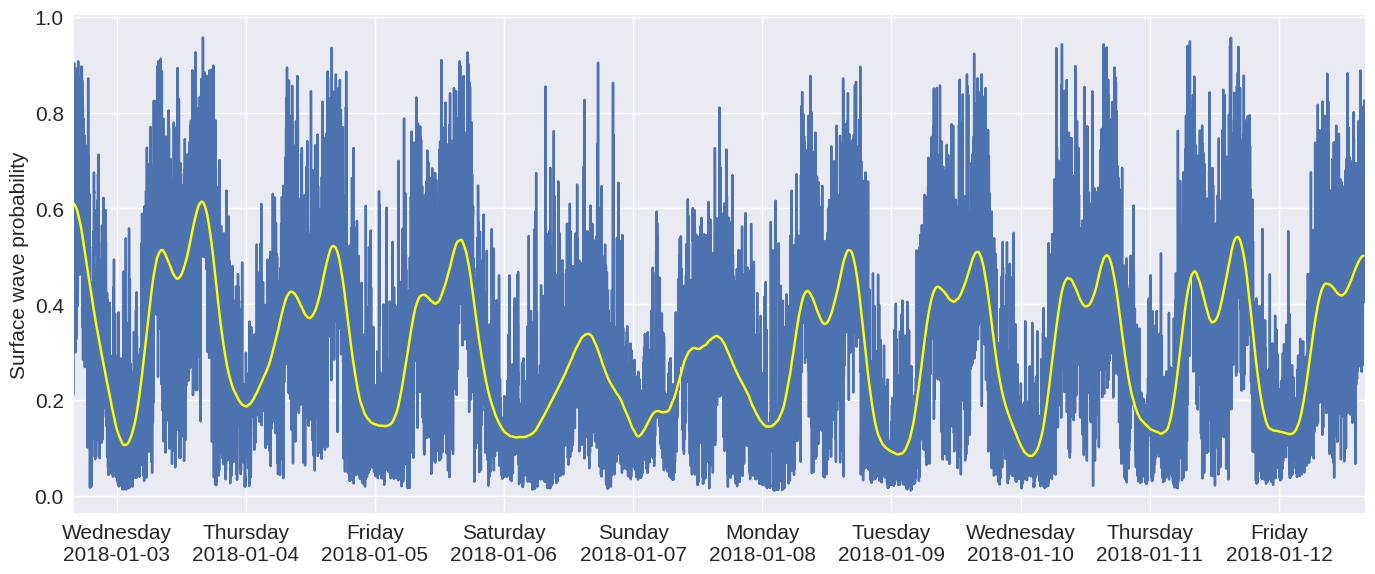
Streaming video ¶
[ ]:
import h5py,numpy,os,glob
import matplotlib.pyplot as plt
from matplotlib.colors import LogNorm
dataset = numpy.loadtxt('5hrs_results.txt',dtype=str)
file_per_plot = 15
k = 1
for i in range(len(dataset)-file_per_plot):
print(i+1)
plt.style.use('seaborn')
fig,ax = plt.subplots(1,file_per_plot,figsize=(18,6),dpi=80)
plt.subplots_adjust(left=0,bottom=0,right=1,top=0.95,wspace=0,hspace=0)
for n in range(file_per_plot):
f = h5py.File('/content/drive/Shared drives/ML4DAS/RawData/1min_ch4650_4850/'+dataset[i+n,0],'r')
data = numpy.array(f[f.get('variable/dat')[0,0]][:200])
f.close()
ax[n].imshow(abs(data),aspect='auto',cmap='inferno',norm=LogNorm())
ax[n].set_title('%.4f'%float(dataset[i+n,1]))
ax[n].axis('off')
plt.savefig('video/%03i.png'%k)
plt.close()
k+=1
[ ]:
%%capture
!rm video/video.mp4
!ffmpeg -r 2 -i video/%03d.png video/video.mp4
[ ]:
from IPython.display import HTML
from base64 import b64encode
mp4 = open('video/video.mp4','rb').read()
data_url = "data:video/mp4;base64," + b64encode(mp4).decode()
HTML("""<video width=100%% controls loop><source src="%s" type="video/mp4"></video>""" % data_url)
Full minute visualization ¶
Channels 5500 to 6000 ¶
Full minute ¶
[ ]:
import h5py,numpy,torch
from PIL import Image
from matplotlib import cm
import matplotlib.pyplot as plt
from torchvision import transforms
def prob_map(data,model,img_size=50,channel_stride=10,sample_stride=50,binary=False,inverse=False):
# Calculate probability map
model.eval() # Set model to evalutation mode
prob_array = numpy.zeros((2,*data.shape)) # Initialize probability map
idxs = numpy.array([[[i,j] for j in range(0,data.shape[1]-img_size+1,sample_stride)] for i in range(0,data.shape[0]-img_size+1,channel_stride)])
idxs = idxs.reshape(idxs.shape[0]*idxs.shape[1],2)
for k,(i,j) in enumerate(idxs):
im = data[i:i+img_size,j:j+img_size].copy() # Create copy of square data window
im = (im-im.min())/(im.max()-im.min()) # Normalize data
im = Image.fromarray(numpy.uint8(cm.gist_earth(im)*255)).convert("RGB") # Convert data to RGB image
# im = transforms.Compose([transforms.Grayscale(), transforms.ToTensor()])(image).view(1,1,200,200)
image = transforms.ToTensor()(im).float().unsqueeze(0) # Convert image to tensor and use first channel
output = model(image) # Run trained model to image
prob = torch.nn.functional.softmax(output,dim=1)
wave_prob = prob[0,1].item()
prob_array[0,i:i+img_size,j:j+img_size]+=wave_prob # Increment probability to map
prob_array[1,i:i+img_size,j:j+img_size]+=1 # Increment scanning index to map
prob_map = prob_array[0]/prob_array[1]
return prob_map[::channel_stride,::sample_stride]
[ ]:
import mldas,torch
f = h5py.File('/content/drive/Shared drives/ML4DAS/RawData/30min_files_NoTrain/Dsi_30min_170803233007_170804000007_ch5500_6000.mat','r')
data = f[f.get('data/dat')[0,0]][300:500,:30000]
model = mldas.ResNet(depth=8,num_classes=2,num_channels=3)
model.load_state_dict(torch.load('training2/model39.pt'))
probmap = prob_map(data,model)
[ ]:
import h5py,numpy,os,glob
import matplotlib.pyplot as plt
from matplotlib.colors import LogNorm
from mpl_toolkits.axes_grid1.axes_divider import make_axes_locatable
plt.style.use('seaborn')
plt.rc('font', size=15)
plt.rc('axes', labelsize=15)
plt.rc('legend', fontsize=15)
plt.rc('xtick', labelsize=15)
plt.rc('ytick', labelsize=15)
fig,(ax0,ax1) = plt.subplots(2,1,figsize=(18,10),dpi=80,sharex=True)
im = ax0.imshow(abs(data),aspect='auto',extent=[0,60,200,0],cmap='inferno',norm=LogNorm(vmin=40))
divider = make_axes_locatable(ax0)
cax = divider.append_axes('right', size='2%', pad=0.1)
plt.colorbar(im, pad=0.02, cax=cax, orientation='vertical').set_label('Absolute raw measurement amplitude')
ax0.set_ylabel('Channels')
im = ax1.imshow(probmap,aspect='auto',extent=[0,60,200,0],cmap='rainbow',interpolation='bicubic')
divider = make_axes_locatable(ax1)
cax = divider.append_axes('right', size='2%', pad=0.1)
plt.colorbar(im, pad=0.02, cax=cax, orientation='vertical').set_label('Coherent surface wave probability')
ax1.set_ylabel('Channels')
ax1.set_xlabel('Time [in seconds]')
plt.tight_layout()
plt.savefig('/content/probmap.pdf')
plt.show()
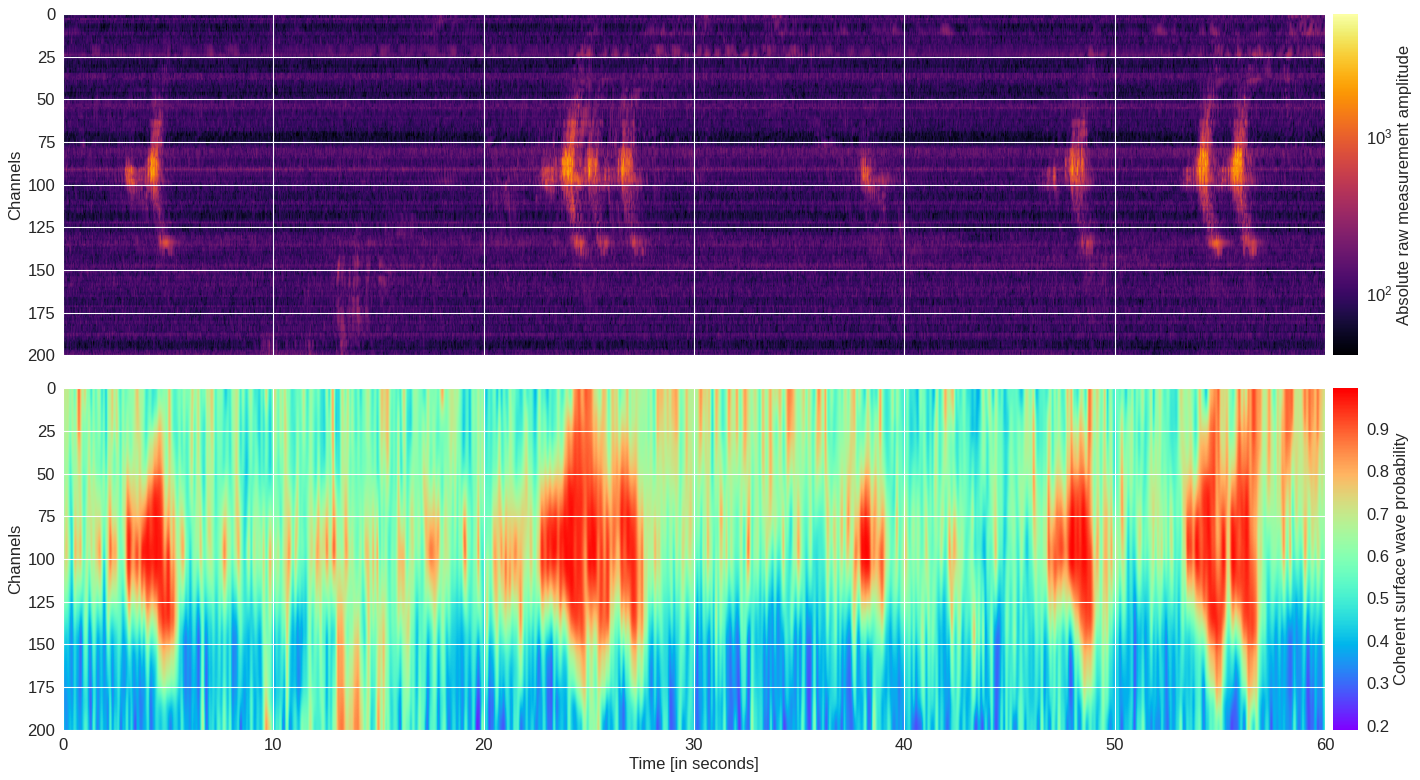
Zoomed-in region ¶
[ ]:
import h5py,mldas,torch
f = h5py.File('/content/drive/Shared drives/ML4DAS/RawData/30min_files_NoTrain/Dsi_30min_170803233007_170804000007_ch5500_6000.mat','r')
data = f[f.get('data/dat')[0,0]][50:150,15500:17000]
model = mldas.ResNet(depth=8,num_classes=2,num_channels=3)
model.load_state_dict(torch.load('training2/model39.pt'))
probmap = prob_map(data,model,img_size=50,channel_stride=50,sample_stride=50)
[ ]:
import h5py,numpy,os,glob
import matplotlib.pyplot as plt
from matplotlib.colors import LogNorm
from mpl_toolkits.axes_grid1.axes_divider import make_axes_locatable
plt.style.use('seaborn')
plt.rc('font', size=15)
plt.rc('axes', labelsize=15)
plt.rc('legend', fontsize=15)
plt.rc('xtick', labelsize=15)
plt.rc('ytick', labelsize=15)
fig,(ax0,ax1) = plt.subplots(2,1,figsize=(10,10),dpi=80)
abs(data).max()
im = ax0.imshow(data,aspect='auto',cmap='seismic',vmin=-500,vmax=500)
divider = make_axes_locatable(ax0)
cax = divider.append_axes('right', size='2%', pad=0.05)
plt.colorbar(im, pad=0.02, cax=cax, orientation='vertical').set_label('Absolute strain measurement')
ax0.set_ylabel('Channels')
im = ax1.imshow(probmap,aspect='auto',cmap='rainbow',interpolation='bicubic',vmin=0,vmax=1)
divider = make_axes_locatable(ax1)
cax = divider.append_axes('right', size='2%', pad=0.05)
plt.colorbar(im, pad=0.02, cax=cax, orientation='vertical').set_label('Surface wave probability')
ax1.set_ylabel('Channels')
ax1.set_xlabel('Samples')
plt.tight_layout()
plt.show()
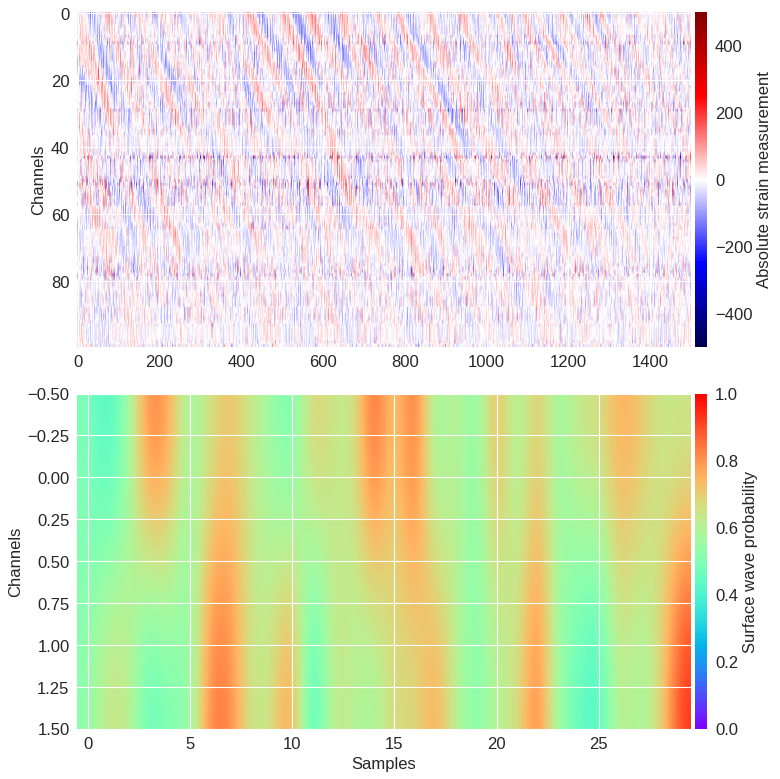
Channels 4650 to 4850 ¶
Two random
[ ]:
import h5py,numpy,os,glob
import matplotlib.pyplot as plt
from matplotlib.colors import LogNorm
plt.style.use('seaborn')
fig,ax = plt.subplots(2,1,figsize=(18,10),dpi=80,sharex=True,sharey=True)
for i,fname in enumerate(['westSac_180104000231_ch4650_4850','westSac_180104001631_ch4650_4850']):
f = h5py.File('/content/drive/Shared drives/ML4DAS/RawData/1min_ch4650_4850/%s.mat'%fname,'r')
data = numpy.array(f[f.get('variable/dat')[0,0]][:200])
f.close()
ax[i].imshow(abs(data),aspect='auto',cmap='inferno',norm=LogNorm())
plt.tight_layout()
plt.show()
plt.close()
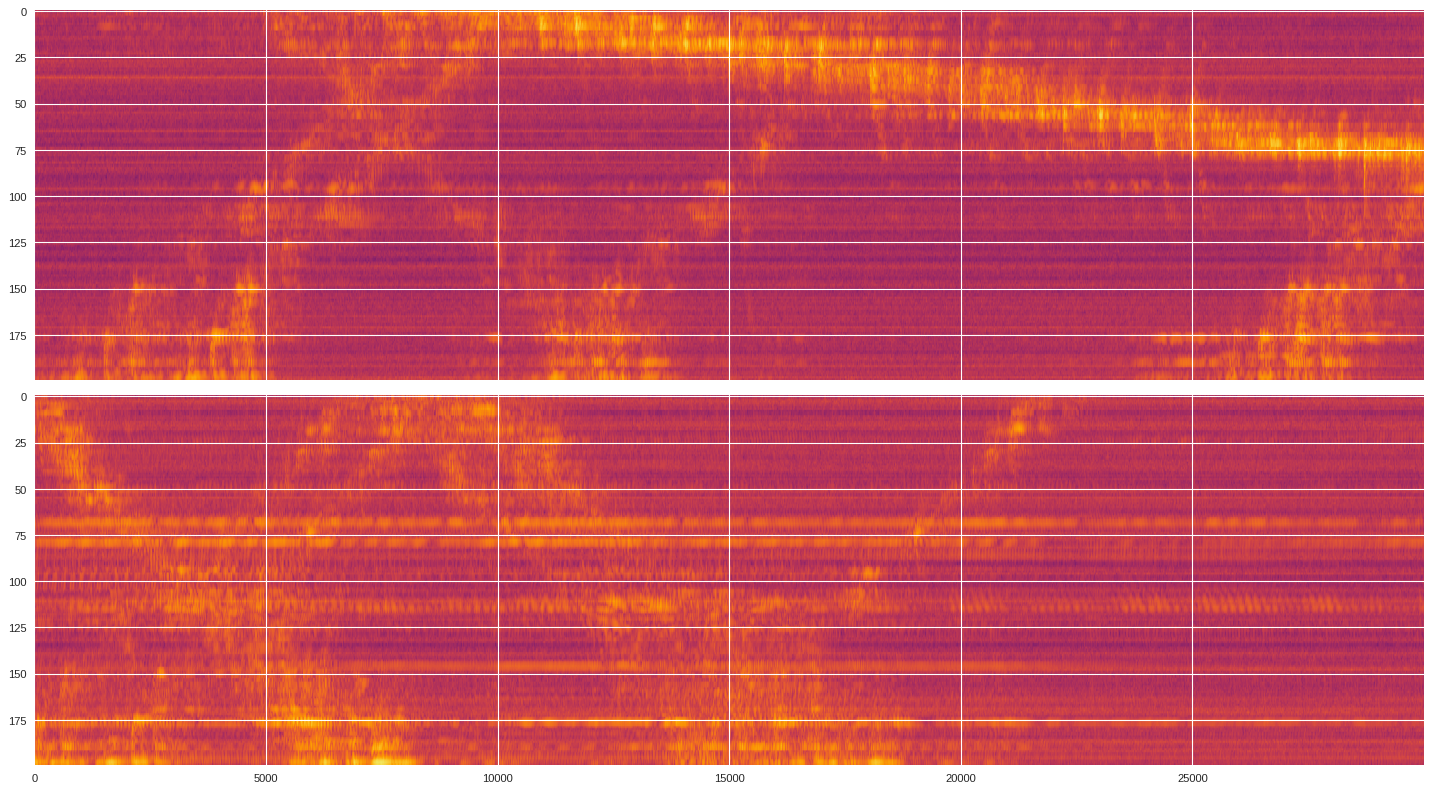
Night time ¶
[ ]:
import h5py,numpy,os,glob
import matplotlib.pyplot as plt
from matplotlib.colors import LogNorm
plt.style.use('seaborn')
plt.figure(figsize=(18,6),dpi=80)
f = h5py.File('/content/drive/Shared drives/ML4DAS/RawData/1min_ch4650_4850/westSac_180104070131_ch4650_4850.mat','r')
data = numpy.array(f[f.get('variable/dat')[0,0]][:200])
f.close()
plt.imshow(abs(data),aspect='auto',cmap='inferno',norm=LogNorm())
plt.colorbar(pad=0.01)
plt.show()
plt.close()
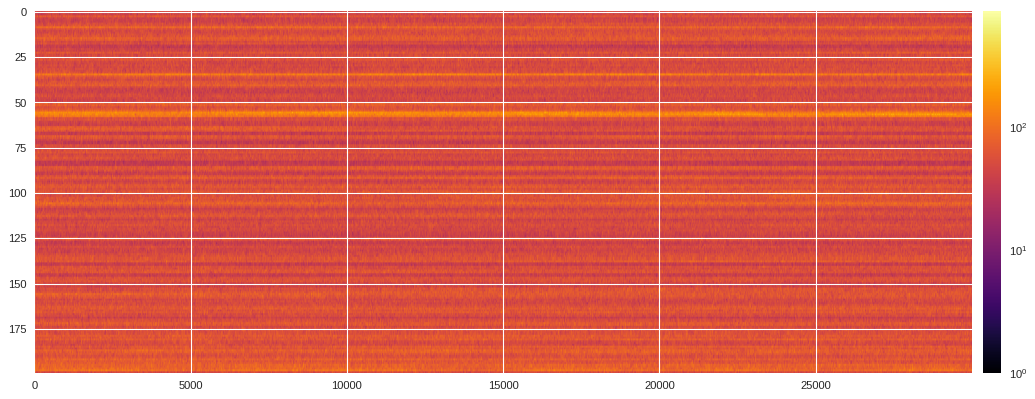
[ ]:
plt.figure(figsize=(18,6),dpi=80)
f = h5py.File('/content/drive/Shared drives/ML4DAS/RawData/1min_ch4650_4850/westSac_180104070131_ch4650_4850.mat','r')
data = numpy.array(f[f.get('variable/dat')[0,0]][:200,:200])
f.close()
vmax = abs(data).max()
plt.imshow(data,aspect='auto',cmap='seismic',vmin=-vmax,vmax=vmax)
plt.colorbar(pad=0.01)
plt.show()
plt.close()
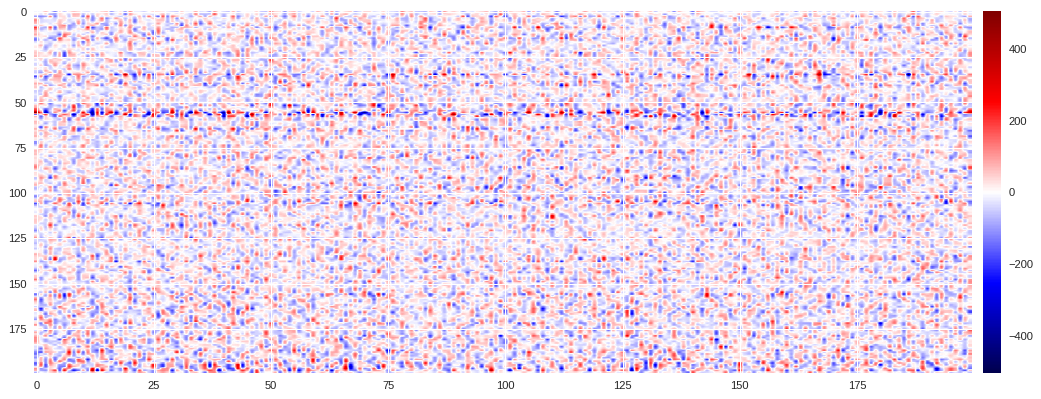
Frequency content ¶
[ ]:
regions = [['westSac_180104001631_ch4650_4850.mat',0,0],
['westSac_180104001631_ch4650_4850.mat',20,0],
['westSac_180104001631_ch4650_4850.mat',50,0],
['westSac_180104001631_ch4650_4850.mat',100,15000],
['westSac_180104001631_ch4650_4850.mat',100,15100],
['westSac_180104001631_ch4650_4850.mat',100,15200],
['westSac_180104001631_ch4650_4850.mat',100,15300],
['westSac_180104001631_ch4650_4850.mat',100,15400],
['westSac_180104001631_ch4650_4850.mat',100,15500],
['westSac_180104001631_ch4650_4850.mat',100,15600],
['westSac_180104000231_ch4650_4850.mat',0,20000]
]
for fname,i,j in regions:
freq_content(fname,i,j,model,img_size=200)
0.8195279836654663
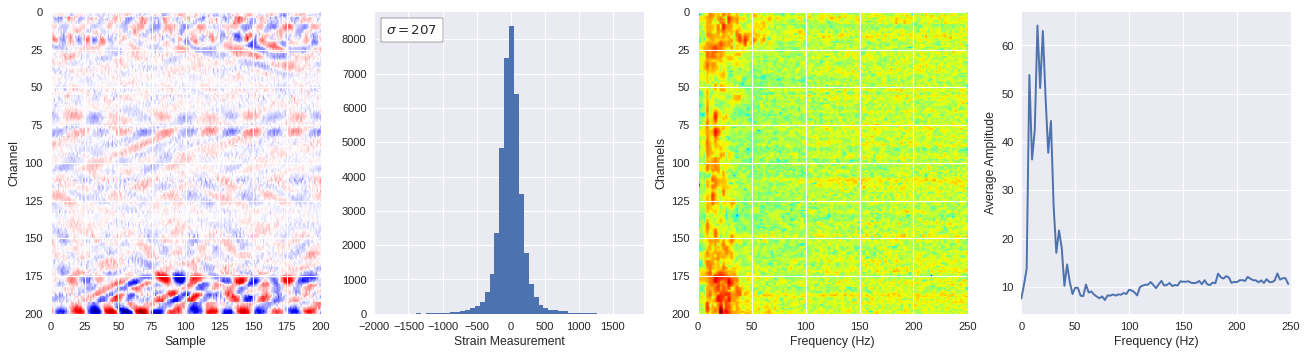
0.8026489615440369
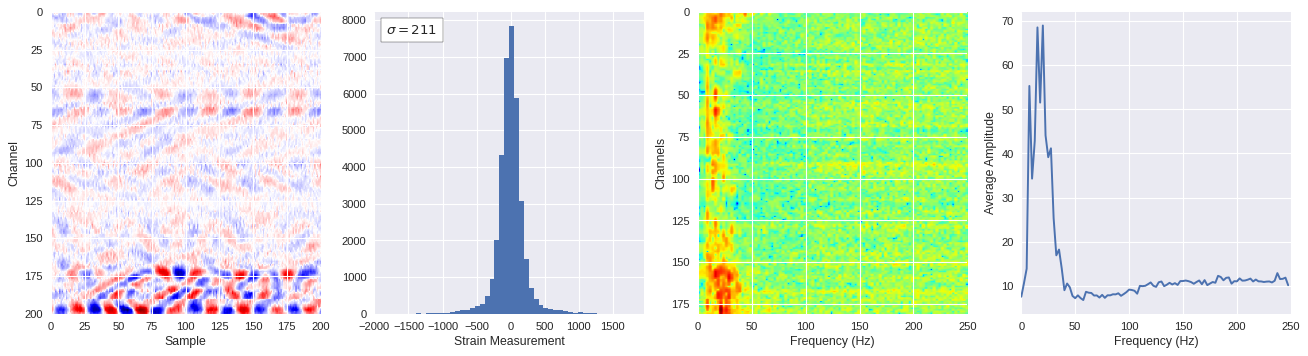
0.8924567699432373
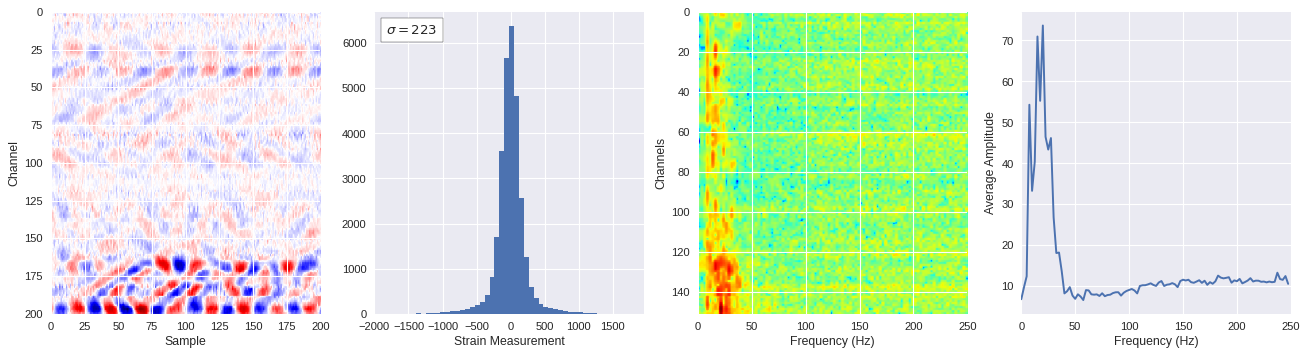
0.9999896287918091
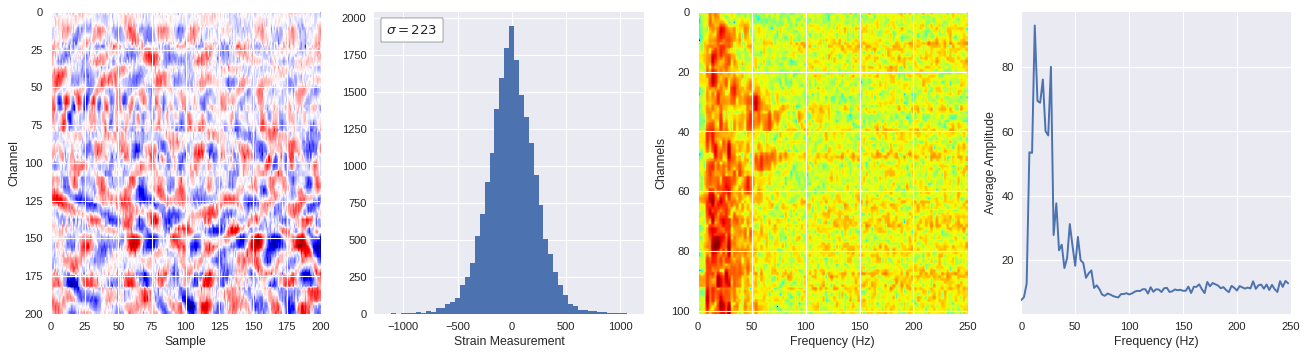
0.32581669092178345
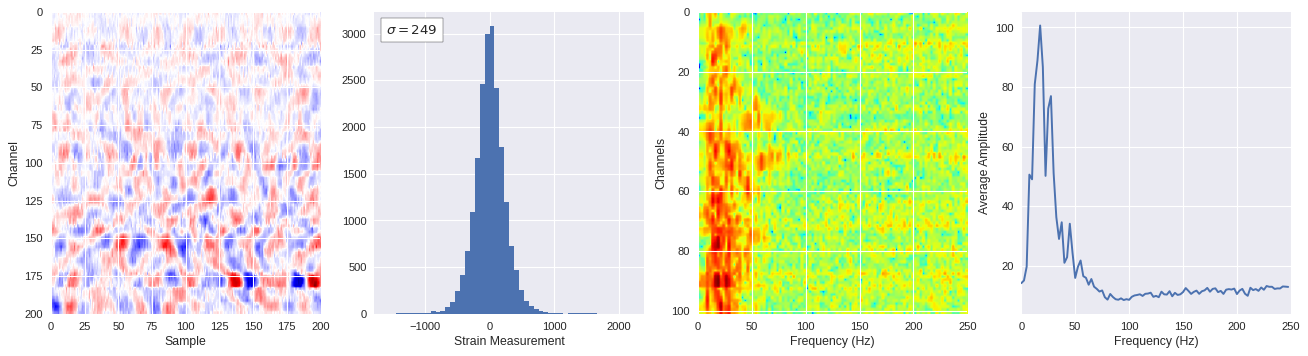
0.5502912998199463
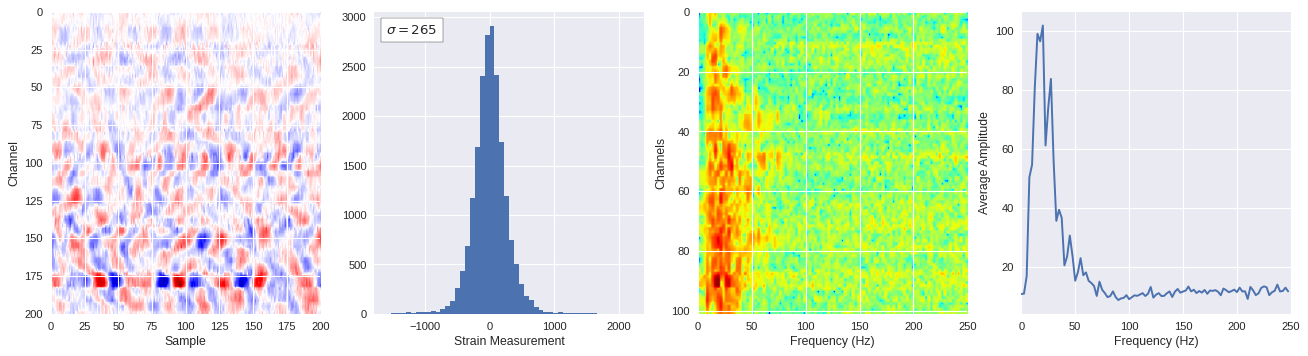
0.9999995231628418
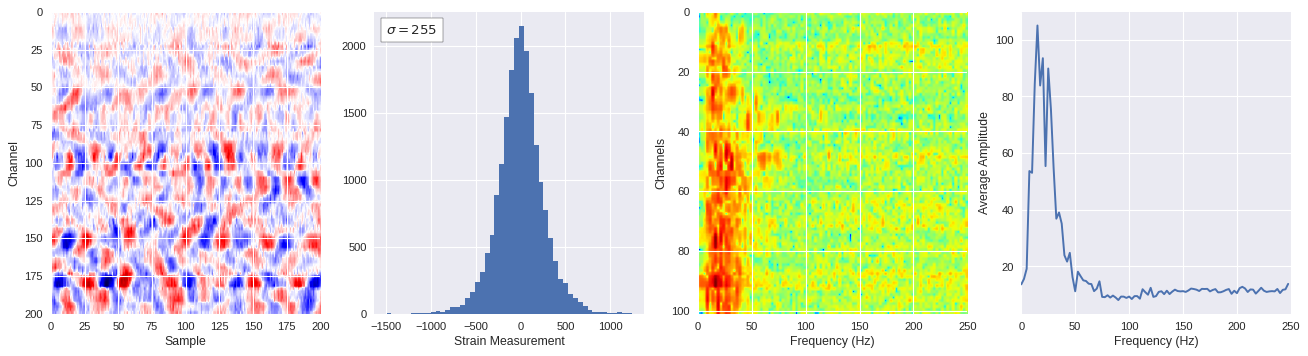
0.999998927116394
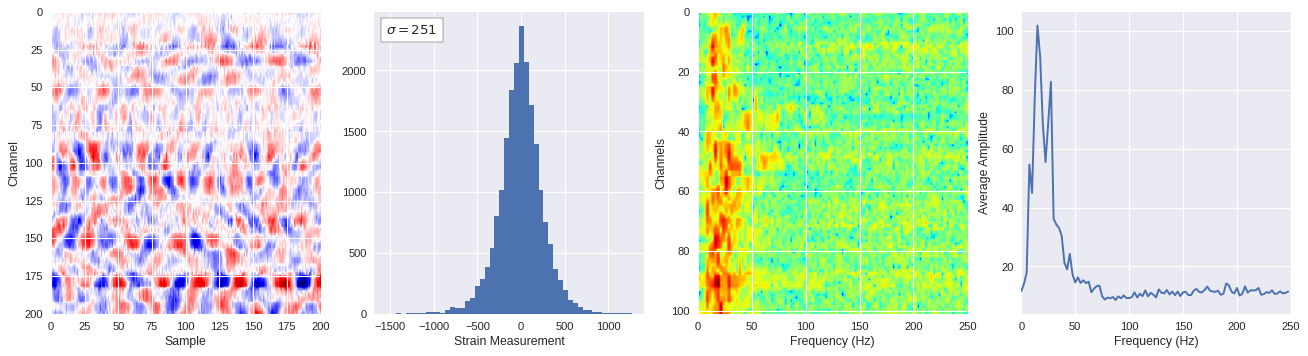
0.9999995231628418
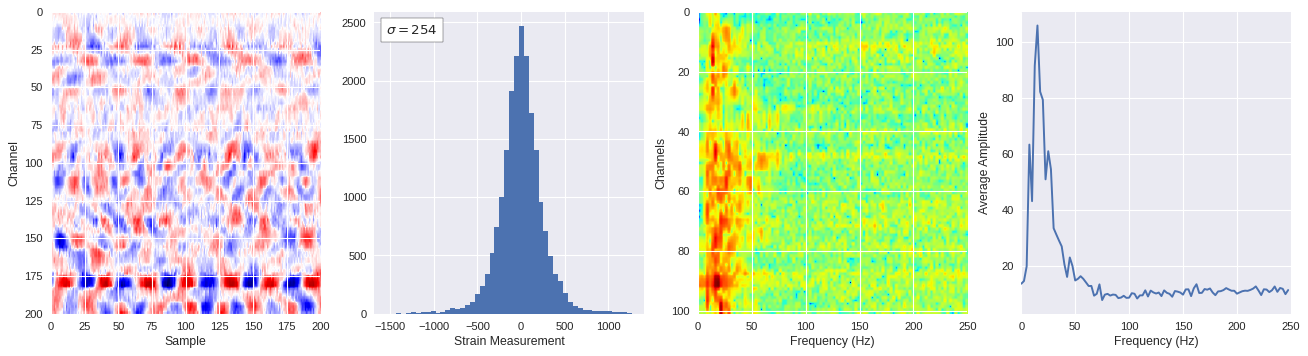
0.9999767541885376
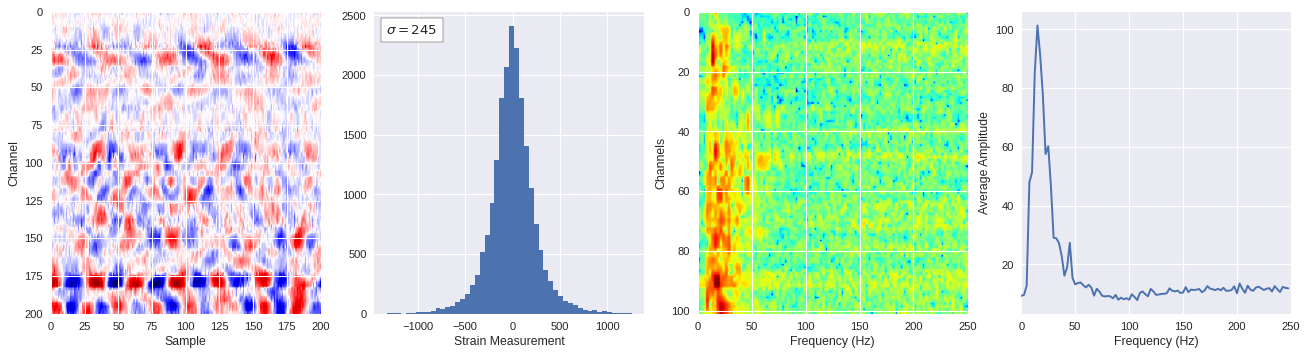
0.03018486499786377
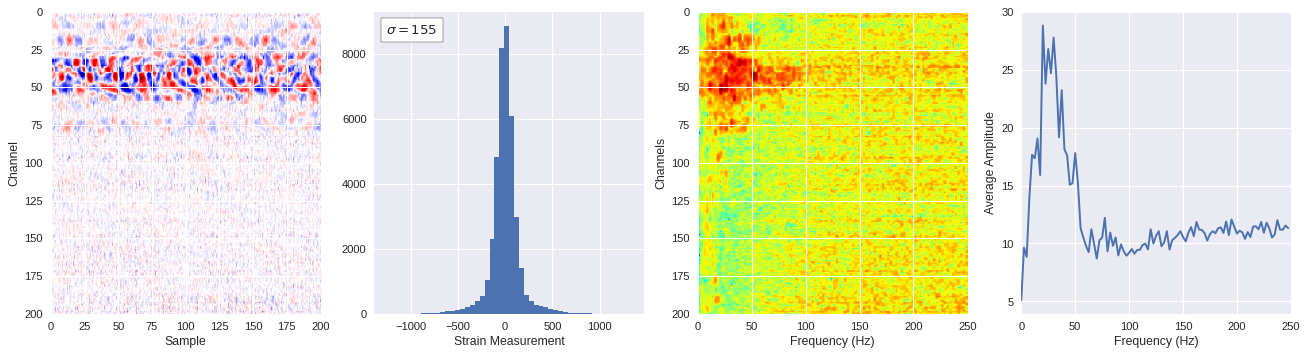
[ ]:
import mldas,torch
from collections import OrderedDict
model = mldas.ResNet(depth=14,num_classes=2)
checkpoint = torch.load('Cori Trained Models/gpu-n8-ds5-bs128-ep50-dp14-lr0.01.pth.tar',map_location=lambda storage, loc: storage)
state_dict = checkpoint['model']
new_state_dict = OrderedDict()
for k, v in state_dict.items():
if k.startswith('module.'):
k = k[7:]
new_state_dict[k] = v
checkpoint['model'] = new_state_dict
model.load_state_dict(checkpoint['model'])
model.eval()
ResNet(
(conv1): Conv2d(3, 16, kernel_size=(3, 3), stride=(1, 1), padding=(1, 1), bias=False)
(bn1): BatchNorm2d(16, eps=1e-05, momentum=0.1, affine=True, track_running_stats=True)
(relu): ReLU(inplace=True)
(layer1): Sequential(
(0): BasicBlock(
(conv1): Conv2d(16, 16, kernel_size=(3, 3), stride=(1, 1), padding=(1, 1), bias=False)
(bn1): BatchNorm2d(16, eps=1e-05, momentum=0.1, affine=True, track_running_stats=True)
(relu): ReLU(inplace=True)
(conv2): Conv2d(16, 16, kernel_size=(3, 3), stride=(1, 1), padding=(1, 1), bias=False)
(bn2): BatchNorm2d(16, eps=1e-05, momentum=0.1, affine=True, track_running_stats=True)
)
(1): BasicBlock(
(conv1): Conv2d(16, 16, kernel_size=(3, 3), stride=(1, 1), padding=(1, 1), bias=False)
(bn1): BatchNorm2d(16, eps=1e-05, momentum=0.1, affine=True, track_running_stats=True)
(relu): ReLU(inplace=True)
(conv2): Conv2d(16, 16, kernel_size=(3, 3), stride=(1, 1), padding=(1, 1), bias=False)
(bn2): BatchNorm2d(16, eps=1e-05, momentum=0.1, affine=True, track_running_stats=True)
)
)
(layer2): Sequential(
(0): BasicBlock(
(conv1): Conv2d(16, 32, kernel_size=(3, 3), stride=(2, 2), padding=(1, 1), bias=False)
(bn1): BatchNorm2d(32, eps=1e-05, momentum=0.1, affine=True, track_running_stats=True)
(relu): ReLU(inplace=True)
(conv2): Conv2d(32, 32, kernel_size=(3, 3), stride=(1, 1), padding=(1, 1), bias=False)
(bn2): BatchNorm2d(32, eps=1e-05, momentum=0.1, affine=True, track_running_stats=True)
(downsample): Sequential(
(0): Conv2d(16, 32, kernel_size=(1, 1), stride=(2, 2), bias=False)
(1): BatchNorm2d(32, eps=1e-05, momentum=0.1, affine=True, track_running_stats=True)
)
)
(1): BasicBlock(
(conv1): Conv2d(32, 32, kernel_size=(3, 3), stride=(1, 1), padding=(1, 1), bias=False)
(bn1): BatchNorm2d(32, eps=1e-05, momentum=0.1, affine=True, track_running_stats=True)
(relu): ReLU(inplace=True)
(conv2): Conv2d(32, 32, kernel_size=(3, 3), stride=(1, 1), padding=(1, 1), bias=False)
(bn2): BatchNorm2d(32, eps=1e-05, momentum=0.1, affine=True, track_running_stats=True)
)
)
(layer3): Sequential(
(0): BasicBlock(
(conv1): Conv2d(32, 64, kernel_size=(3, 3), stride=(2, 2), padding=(1, 1), bias=False)
(bn1): BatchNorm2d(64, eps=1e-05, momentum=0.1, affine=True, track_running_stats=True)
(relu): ReLU(inplace=True)
(conv2): Conv2d(64, 64, kernel_size=(3, 3), stride=(1, 1), padding=(1, 1), bias=False)
(bn2): BatchNorm2d(64, eps=1e-05, momentum=0.1, affine=True, track_running_stats=True)
(downsample): Sequential(
(0): Conv2d(32, 64, kernel_size=(1, 1), stride=(2, 2), bias=False)
(1): BatchNorm2d(64, eps=1e-05, momentum=0.1, affine=True, track_running_stats=True)
)
)
(1): BasicBlock(
(conv1): Conv2d(64, 64, kernel_size=(3, 3), stride=(1, 1), padding=(1, 1), bias=False)
(bn1): BatchNorm2d(64, eps=1e-05, momentum=0.1, affine=True, track_running_stats=True)
(relu): ReLU(inplace=True)
(conv2): Conv2d(64, 64, kernel_size=(3, 3), stride=(1, 1), padding=(1, 1), bias=False)
(bn2): BatchNorm2d(64, eps=1e-05, momentum=0.1, affine=True, track_running_stats=True)
)
)
(avgpool): AdaptiveAvgPool2d(output_size=1)
(fc): Linear(in_features=64, out_features=2, bias=True)
)
[ ]:
regions = [['westSac_180104001631_ch4650_4850.mat',0,0],
['westSac_180104001631_ch4650_4850.mat',0,0],
['westSac_180104001631_ch4650_4850.mat',0,0],
['westSac_180104001631_ch4650_4850.mat',0
,15000],
['westSac_180104001631_ch4650_4850.mat',100,15100],
['westSac_180104001631_ch4650_4850.mat',100,15200],
['westSac_180104001631_ch4650_4850.mat',100,15300],
['westSac_180104001631_ch4650_4850.mat',100,15400],
['westSac_180104001631_ch4650_4850.mat',100,15500],
['westSac_180104001631_ch4650_4850.mat',100,15600],
['westSac_180104000231_ch4650_4850.mat',0,20000]
]
for fname,i,j in regions:
freq_content(fname,i,j,model)
0.9999995231628418
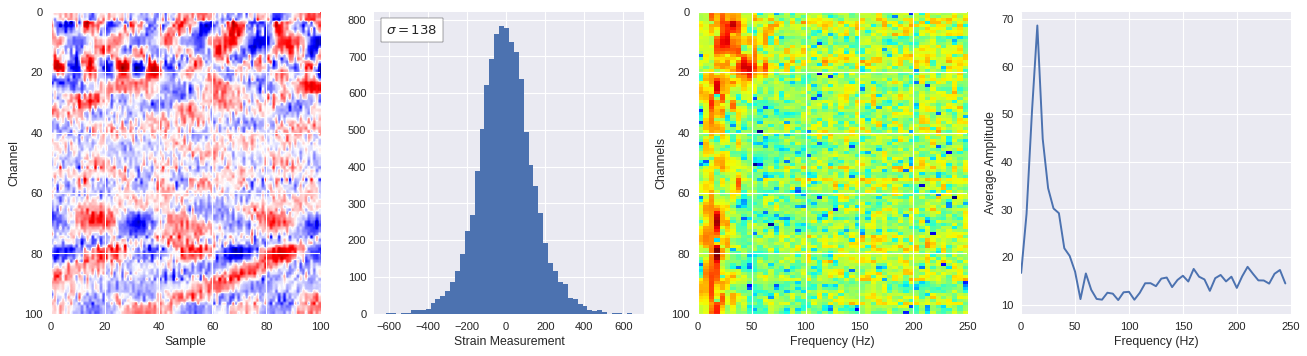
1.0
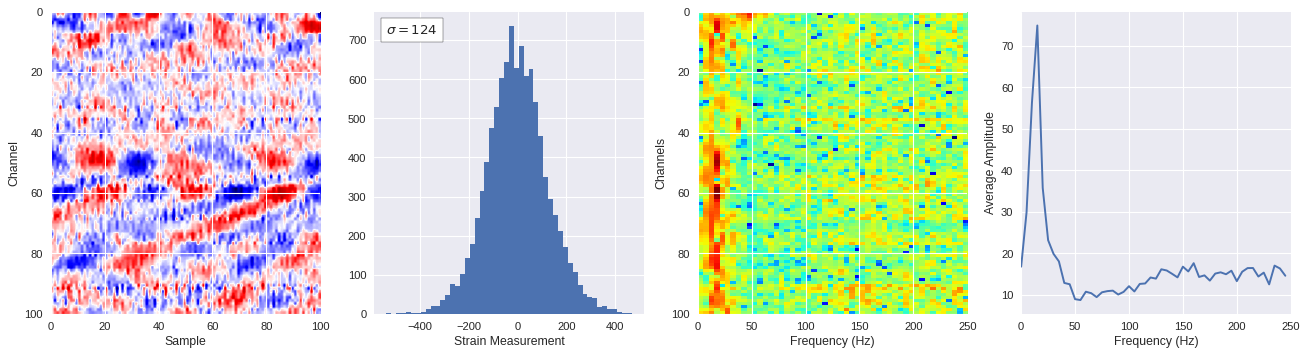
1.0
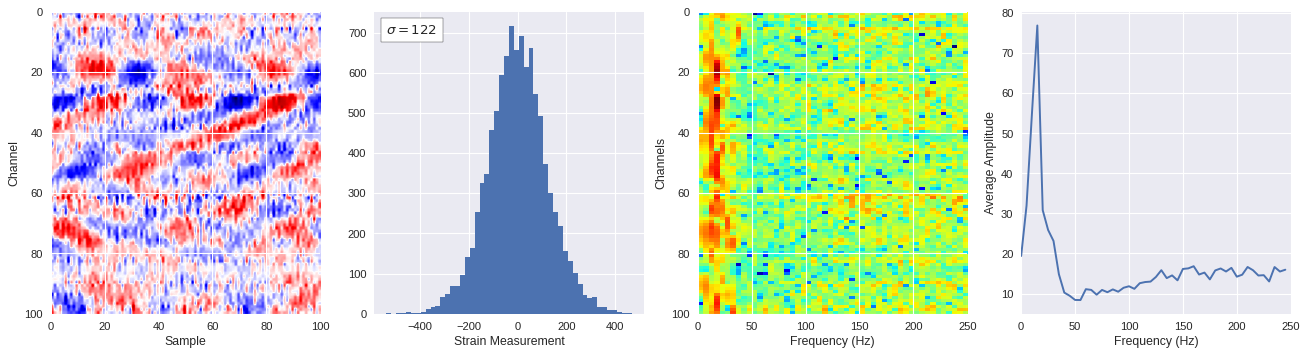
1.0
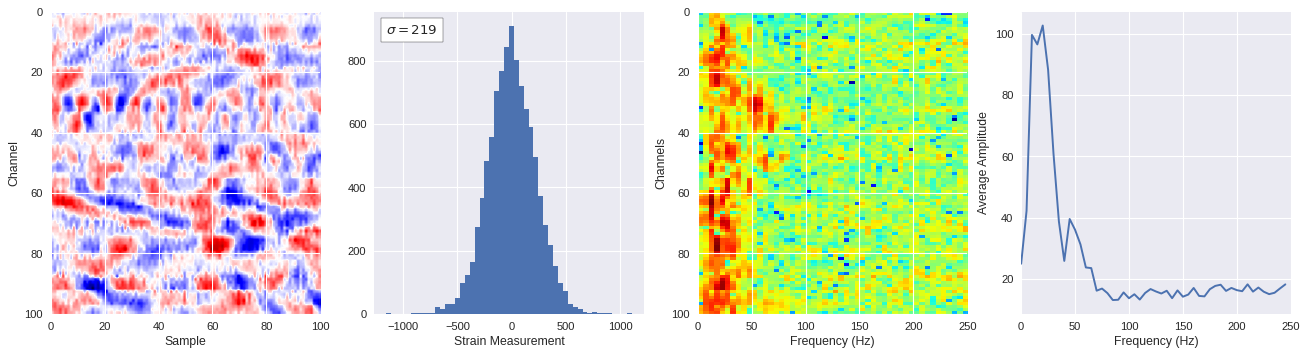
1.0
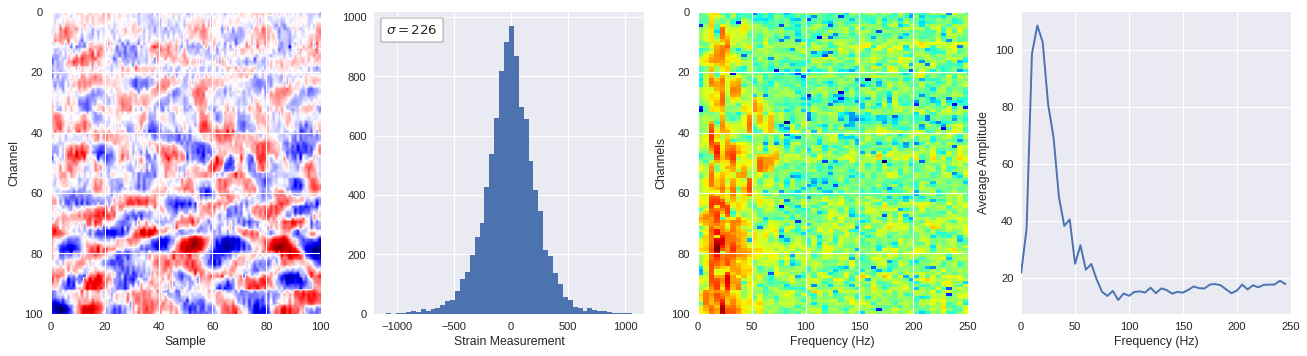
0.7566111087799072
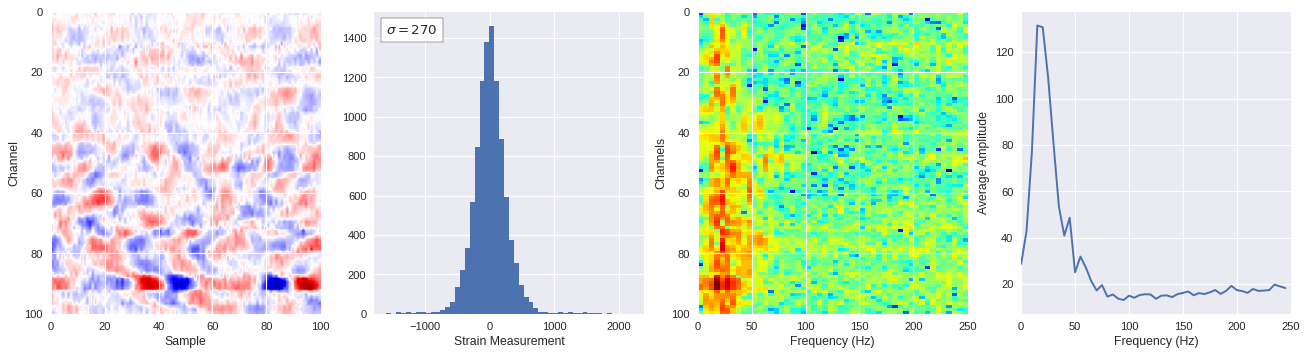
1.0
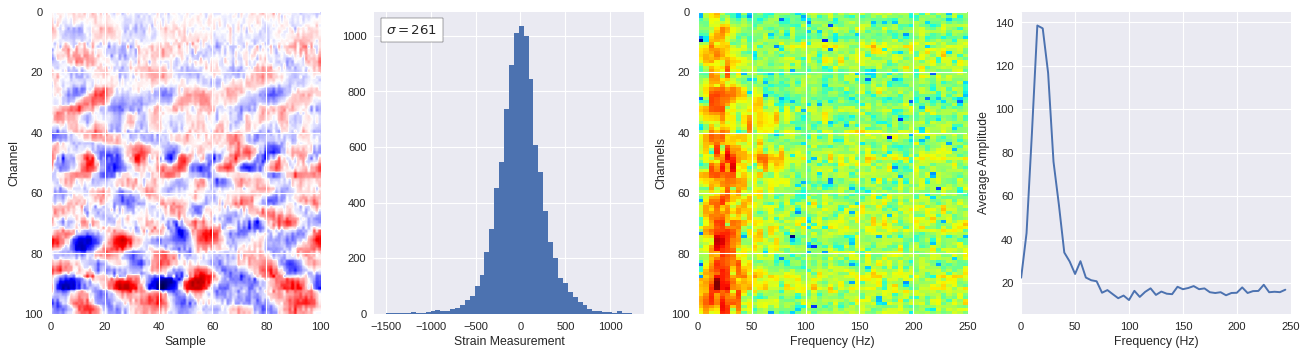
1.0
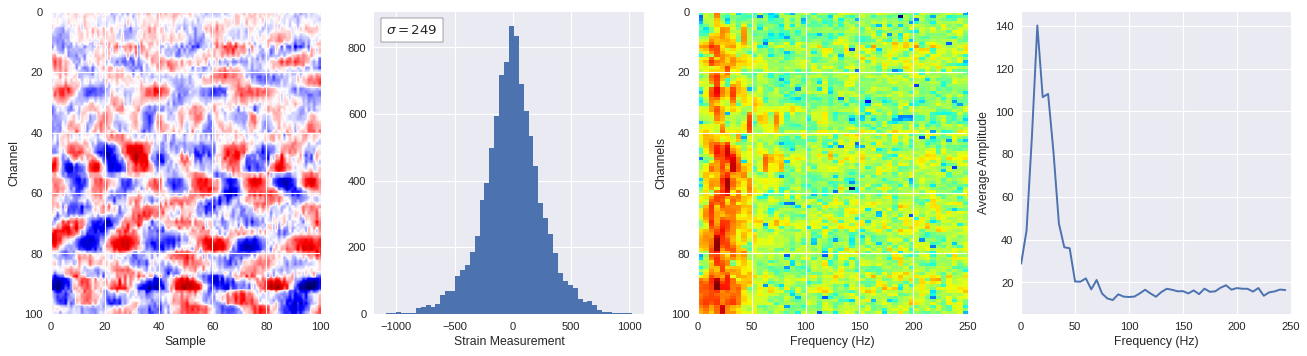
1.0
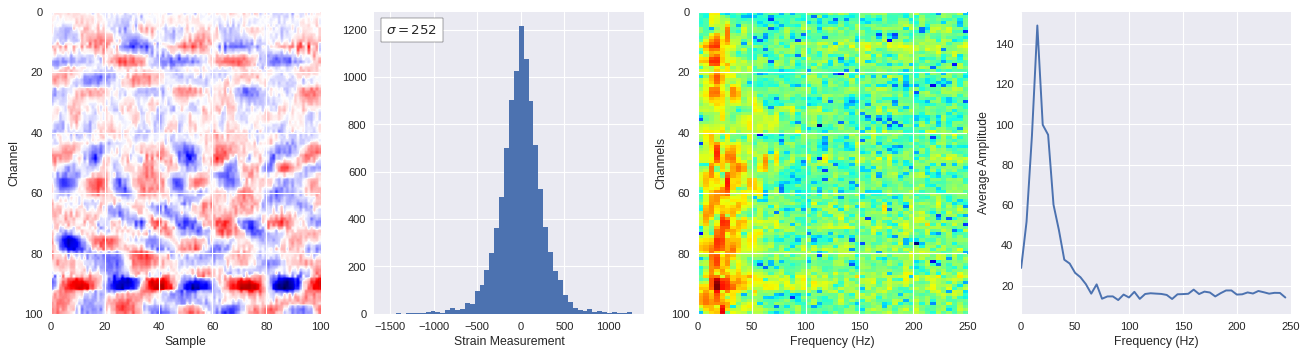
1.0
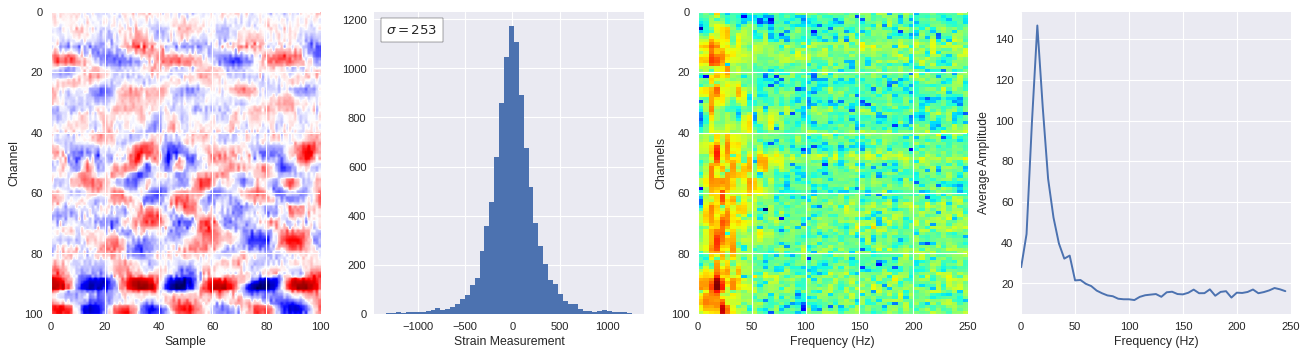
0.9994951486587524
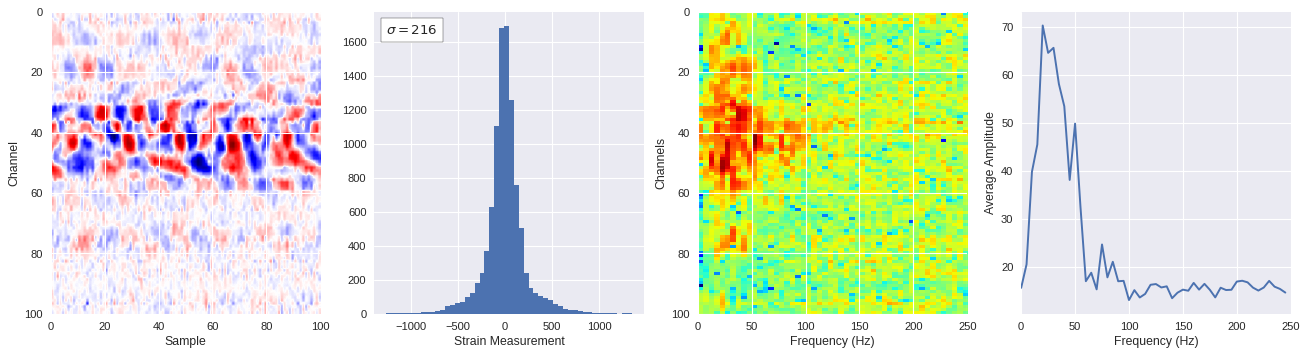
[ ]:
import mldas,torch
from collections import OrderedDict
model = mldas.ResNet(depth=8,num_classes=2)
checkpoint = torch.load('Cori Trained Models/gpu-n16-ds100-bs512-ep5-dp8-lr0.05.pth.tar',map_location=lambda storage, loc: storage)
state_dict = checkpoint['model']
new_state_dict = OrderedDict()
for k, v in state_dict.items():
if k.startswith('module.'):
k = k[7:]
new_state_dict[k] = v
checkpoint['model'] = new_state_dict
model.load_state_dict(checkpoint['model'])
model.eval()
ResNet(
(conv1): Conv2d(3, 16, kernel_size=(3, 3), stride=(1, 1), padding=(1, 1), bias=False)
(bn1): BatchNorm2d(16, eps=1e-05, momentum=0.1, affine=True, track_running_stats=True)
(relu): ReLU(inplace=True)
(layer1): Sequential(
(0): BasicBlock(
(conv1): Conv2d(16, 16, kernel_size=(3, 3), stride=(1, 1), padding=(1, 1), bias=False)
(bn1): BatchNorm2d(16, eps=1e-05, momentum=0.1, affine=True, track_running_stats=True)
(relu): ReLU(inplace=True)
(conv2): Conv2d(16, 16, kernel_size=(3, 3), stride=(1, 1), padding=(1, 1), bias=False)
(bn2): BatchNorm2d(16, eps=1e-05, momentum=0.1, affine=True, track_running_stats=True)
)
)
(layer2): Sequential(
(0): BasicBlock(
(conv1): Conv2d(16, 32, kernel_size=(3, 3), stride=(2, 2), padding=(1, 1), bias=False)
(bn1): BatchNorm2d(32, eps=1e-05, momentum=0.1, affine=True, track_running_stats=True)
(relu): ReLU(inplace=True)
(conv2): Conv2d(32, 32, kernel_size=(3, 3), stride=(1, 1), padding=(1, 1), bias=False)
(bn2): BatchNorm2d(32, eps=1e-05, momentum=0.1, affine=True, track_running_stats=True)
(downsample): Sequential(
(0): Conv2d(16, 32, kernel_size=(1, 1), stride=(2, 2), bias=False)
(1): BatchNorm2d(32, eps=1e-05, momentum=0.1, affine=True, track_running_stats=True)
)
)
)
(layer3): Sequential(
(0): BasicBlock(
(conv1): Conv2d(32, 64, kernel_size=(3, 3), stride=(2, 2), padding=(1, 1), bias=False)
(bn1): BatchNorm2d(64, eps=1e-05, momentum=0.1, affine=True, track_running_stats=True)
(relu): ReLU(inplace=True)
(conv2): Conv2d(64, 64, kernel_size=(3, 3), stride=(1, 1), padding=(1, 1), bias=False)
(bn2): BatchNorm2d(64, eps=1e-05, momentum=0.1, affine=True, track_running_stats=True)
(downsample): Sequential(
(0): Conv2d(32, 64, kernel_size=(1, 1), stride=(2, 2), bias=False)
(1): BatchNorm2d(64, eps=1e-05, momentum=0.1, affine=True, track_running_stats=True)
)
)
)
(avgpool): AdaptiveAvgPool2d(output_size=1)
(fc): Linear(in_features=64, out_features=2, bias=True)
)
[ ]:
regions = [['westSac_180104001631_ch4650_4850.mat',0,0],
['westSac_180104001631_ch4650_4850.mat',20,0],
['westSac_180104001631_ch4650_4850.mat',50,0],
['westSac_180104001631_ch4650_4850.mat',100,15000],
['westSac_180104001631_ch4650_4850.mat',100,15100],
['westSac_180104001631_ch4650_4850.mat',100,15200],
['westSac_180104001631_ch4650_4850.mat',100,15300],
['westSac_180104001631_ch4650_4850.mat',100,15400],
['westSac_180104001631_ch4650_4850.mat',100,15500],
['westSac_180104001631_ch4650_4850.mat',100,15600],
['westSac_180104000231_ch4650_4850.mat',0,20000]
]
for fname,i,j in regions:
freq_content(fname,i,j,model)
0.9999991655349731
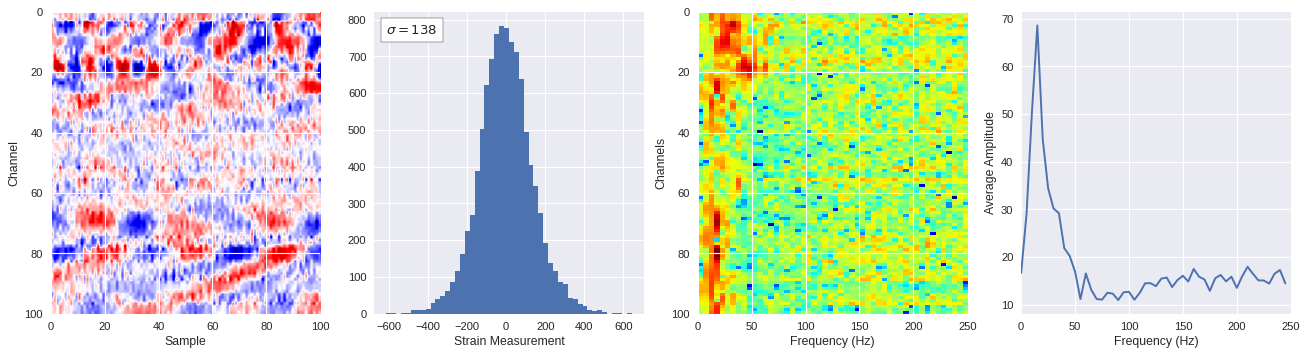
1.0
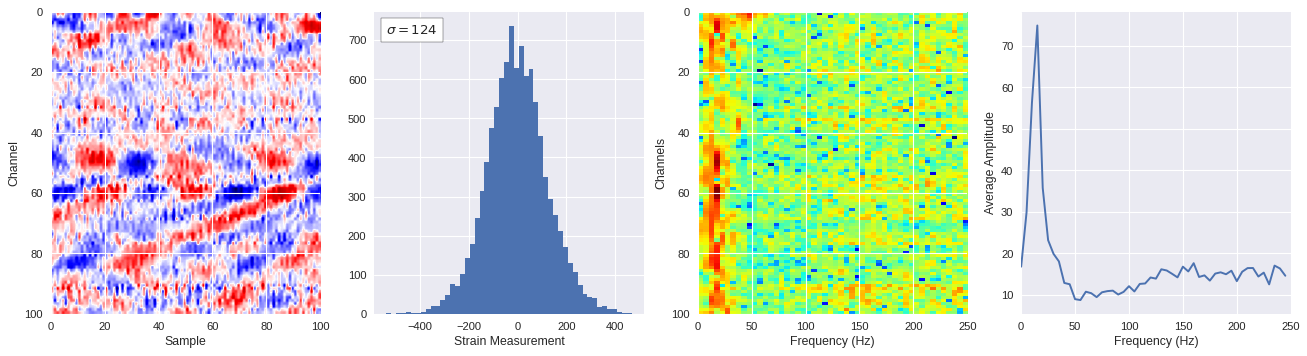
1.0
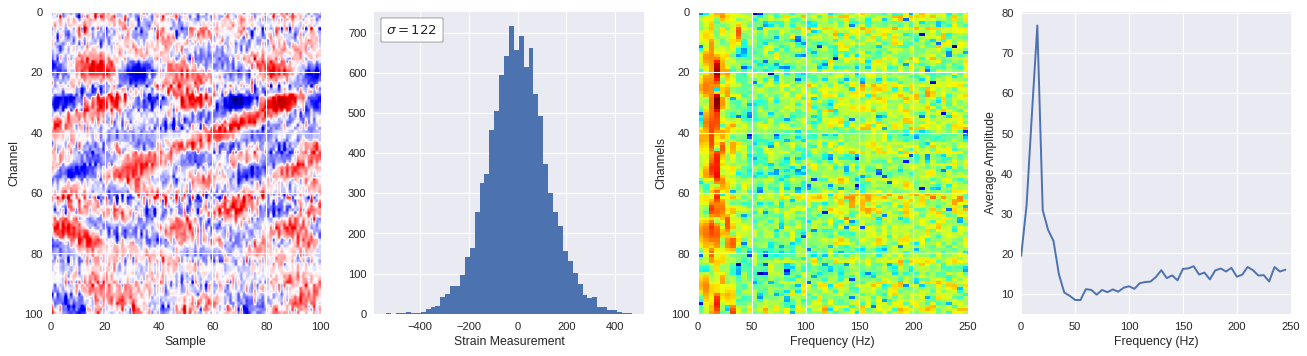
1.0
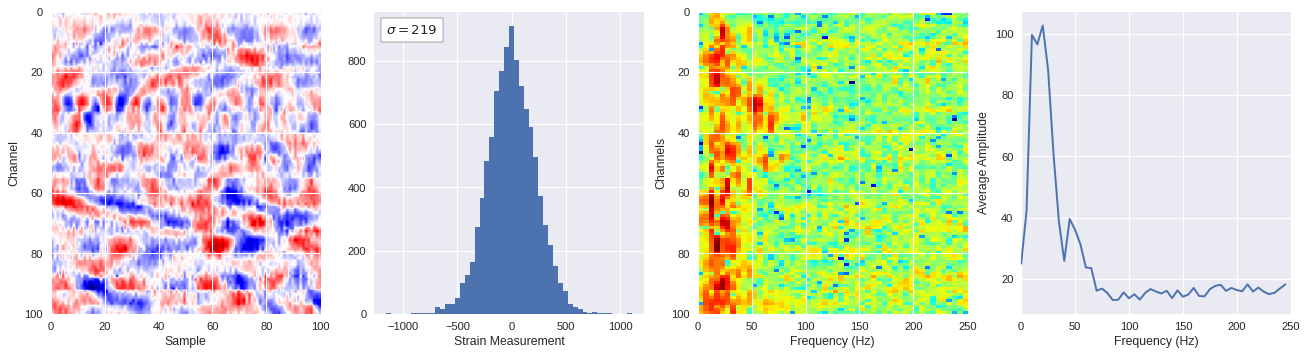
1.0
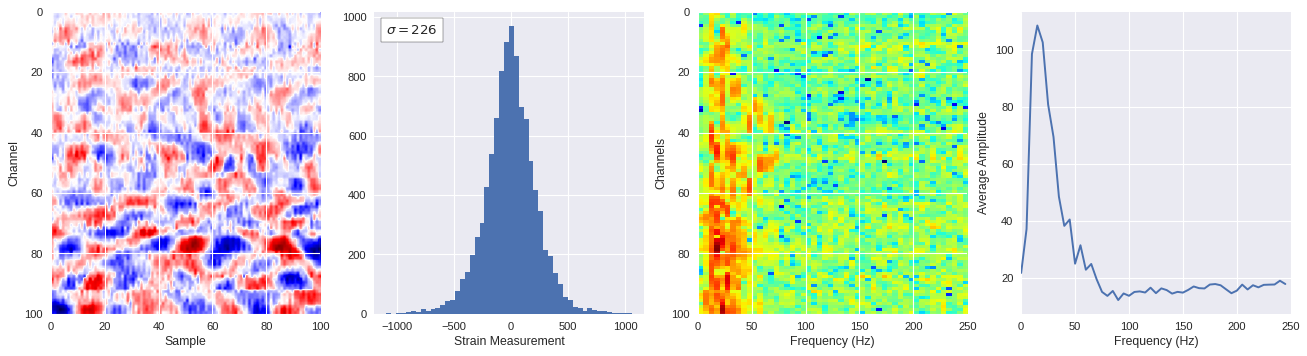
0.9838690161705017
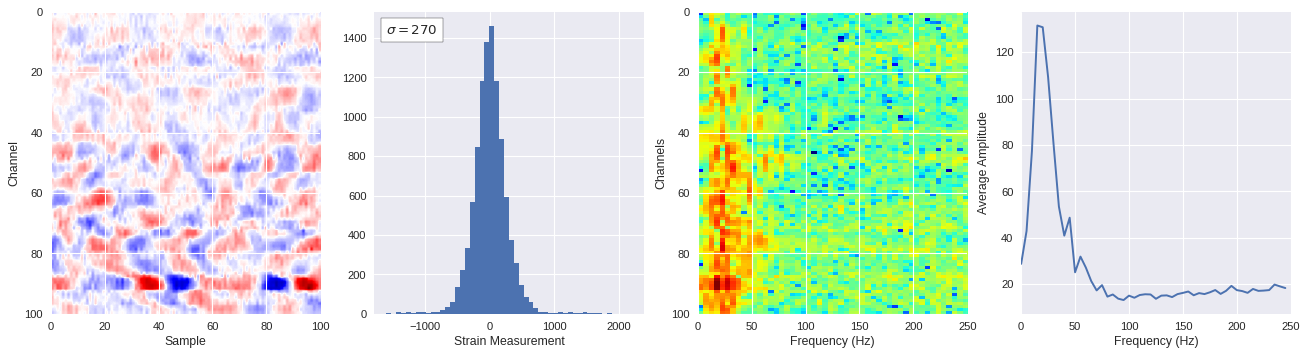
1.0
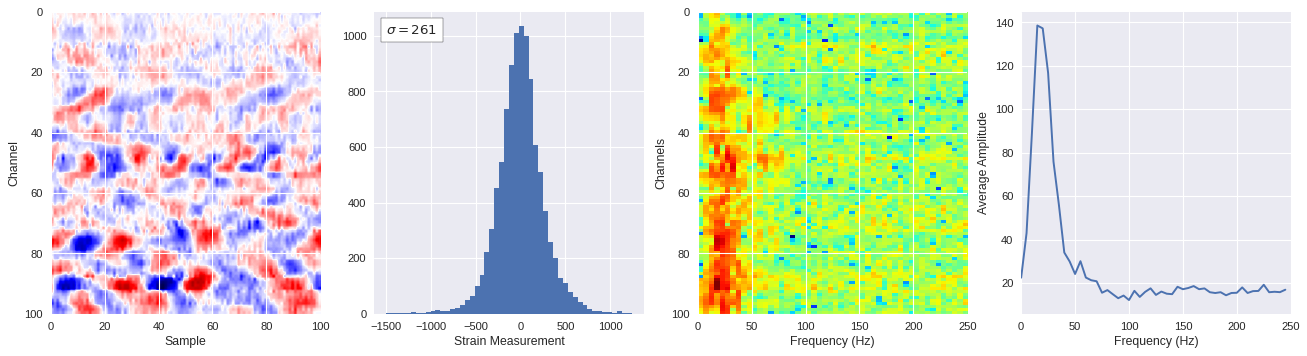
1.0
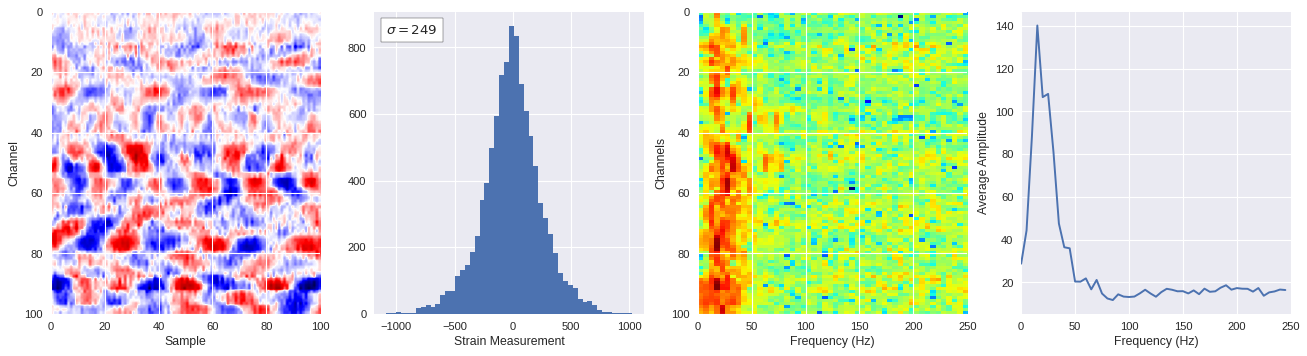
1.0
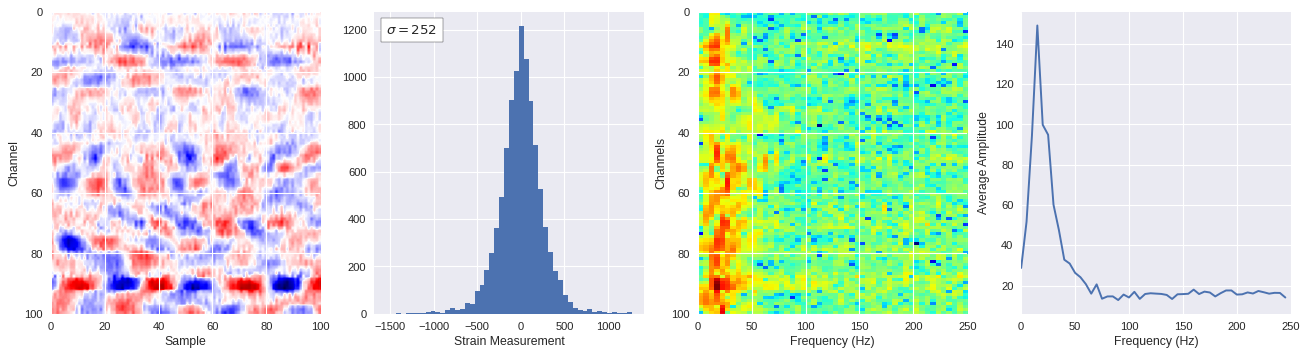
1.0
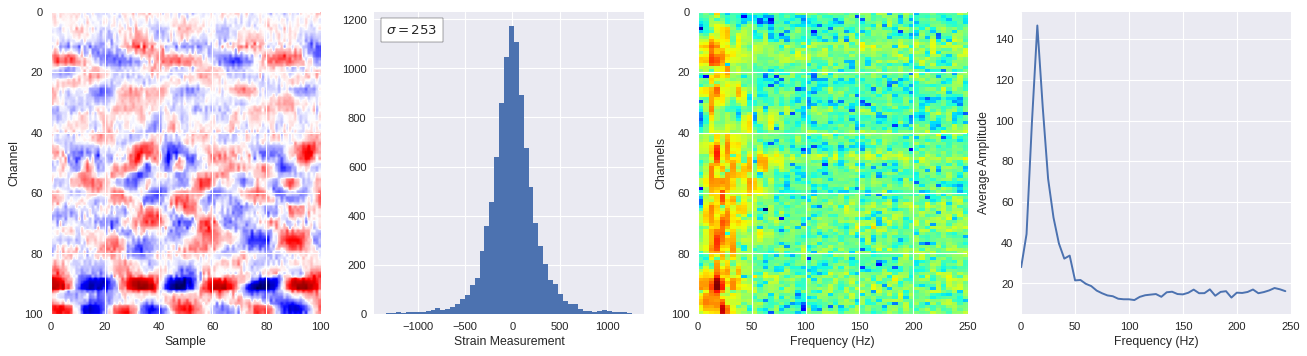
0.999638557434082
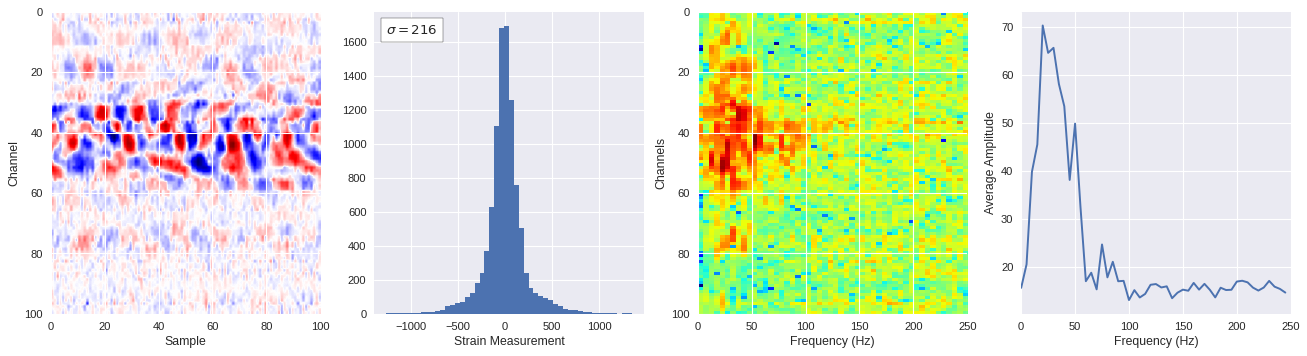
[ ]:
import mldas,torch
from collections import OrderedDict
model = mldas.ResNet(depth=20,num_classes=1)
print(model)
checkpoint = torch.load('../Unary vs. Binary/model_111_lr0.05_bis.pt',map_location=lambda storage, loc: storage)
print(checkpoint.keys())
model.load_state_dict(checkpoint)
model.eval()
[ ]: